-
Book Overview & Buying
-
Table Of Contents
-
Feedback & Rating
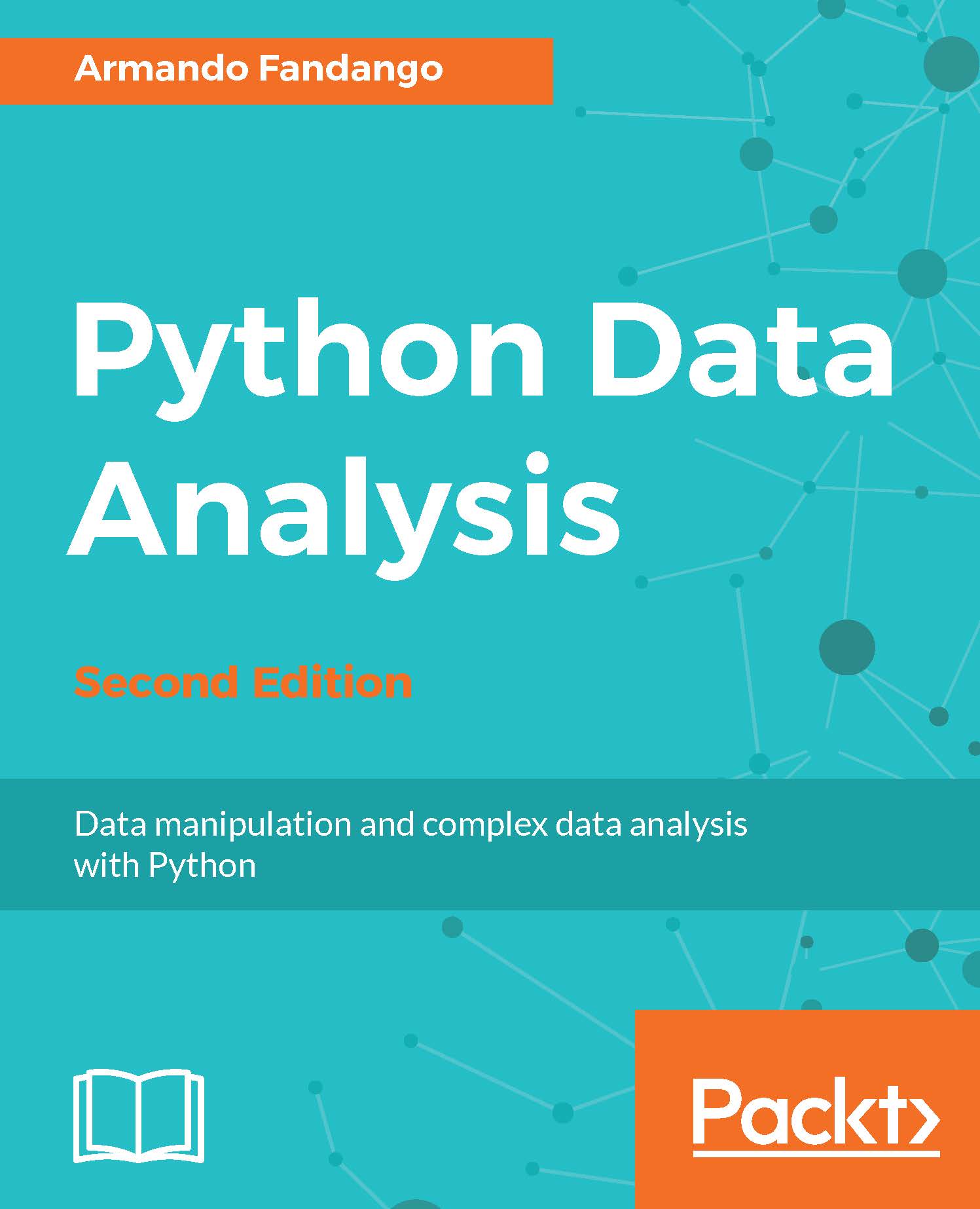
Python Data Analysis, Second Edition
By :
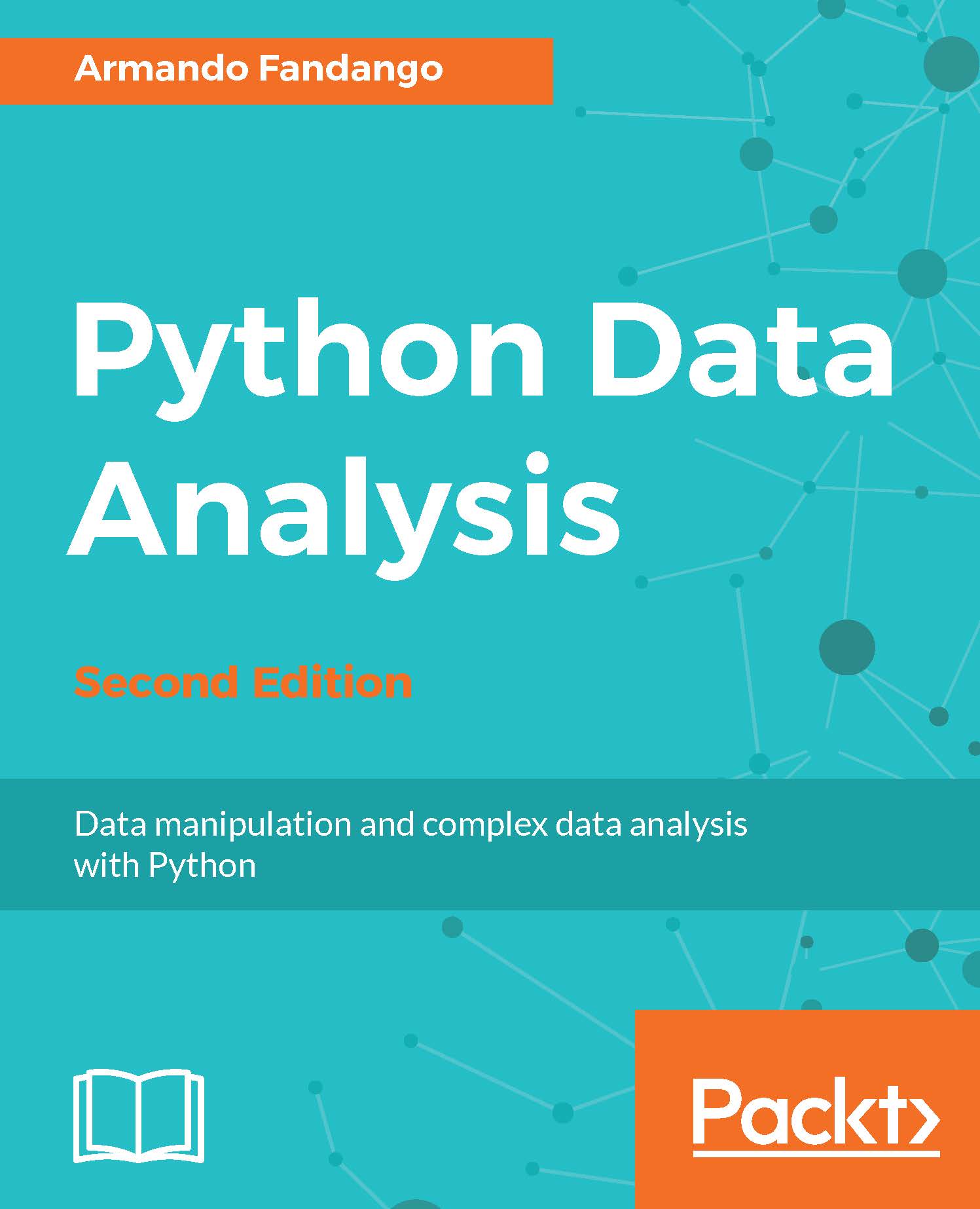
We looked over the borders of Python in this chapter. Outside the Python ecosystem, programming languages such as R, C, Java, and Fortran are fairly popular. We looked at libraries that provide glue to connect Python with external code, rpy2
for R, SWIG and Boost for C, JPype
for Java, and f2py
for Fortran. Cloud computing aims to deliver computing power as a utility over the Internet. A brief overview of PythonAnywhere--a Cloud computing services specialized in Python was also given.
The next chapter, Chapter 12, Performance Tuning, Profiling, and Concurrency, gives hints on improving performance. Typically, we can speed up Python code by optimizing our code using parallelization or rewriting parts of our code in C. We will also discuss several profiling tools and concurrency APIs.