-
Book Overview & Buying
-
Table Of Contents
-
Feedback & Rating
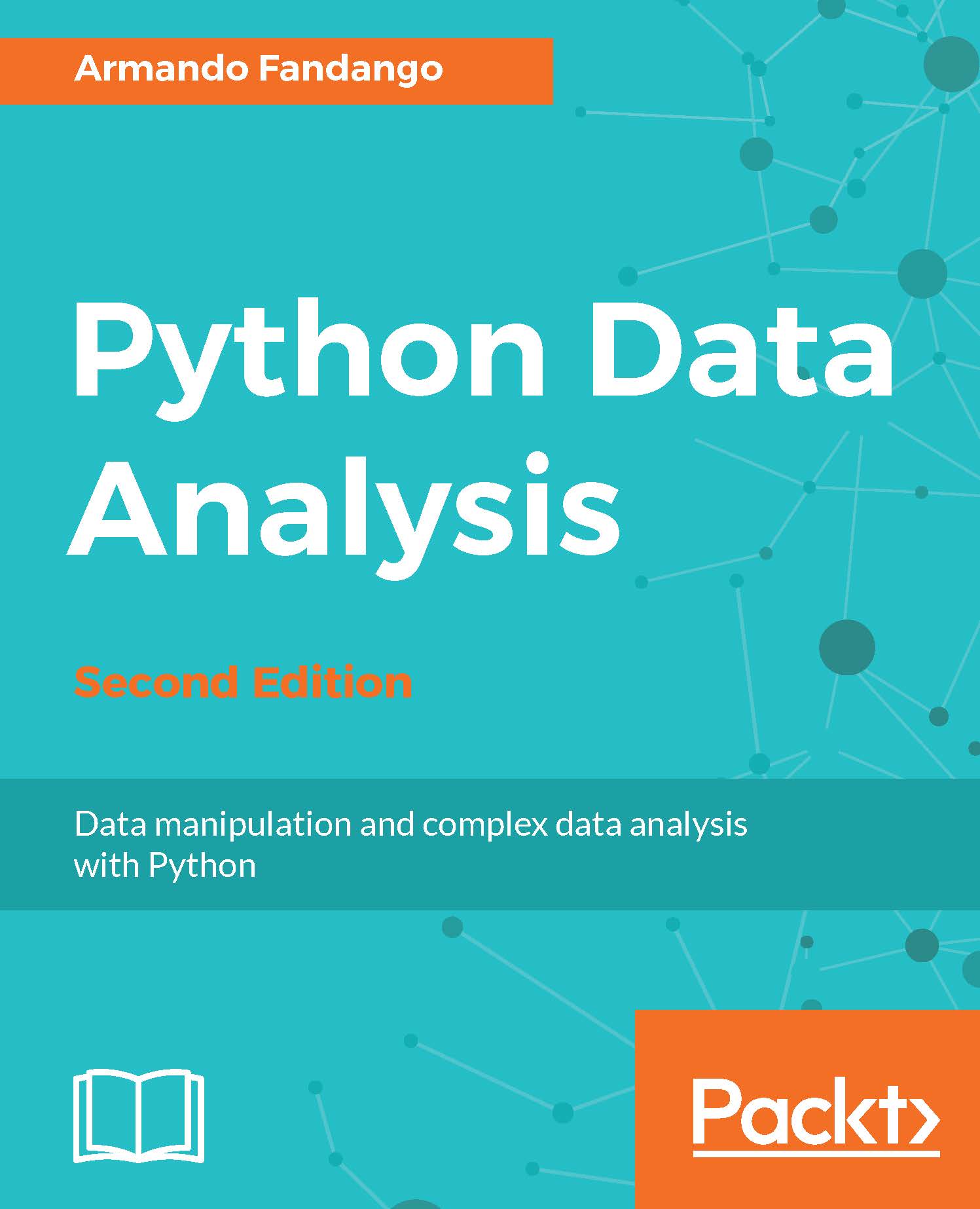
Python Data Analysis, Second Edition
By :
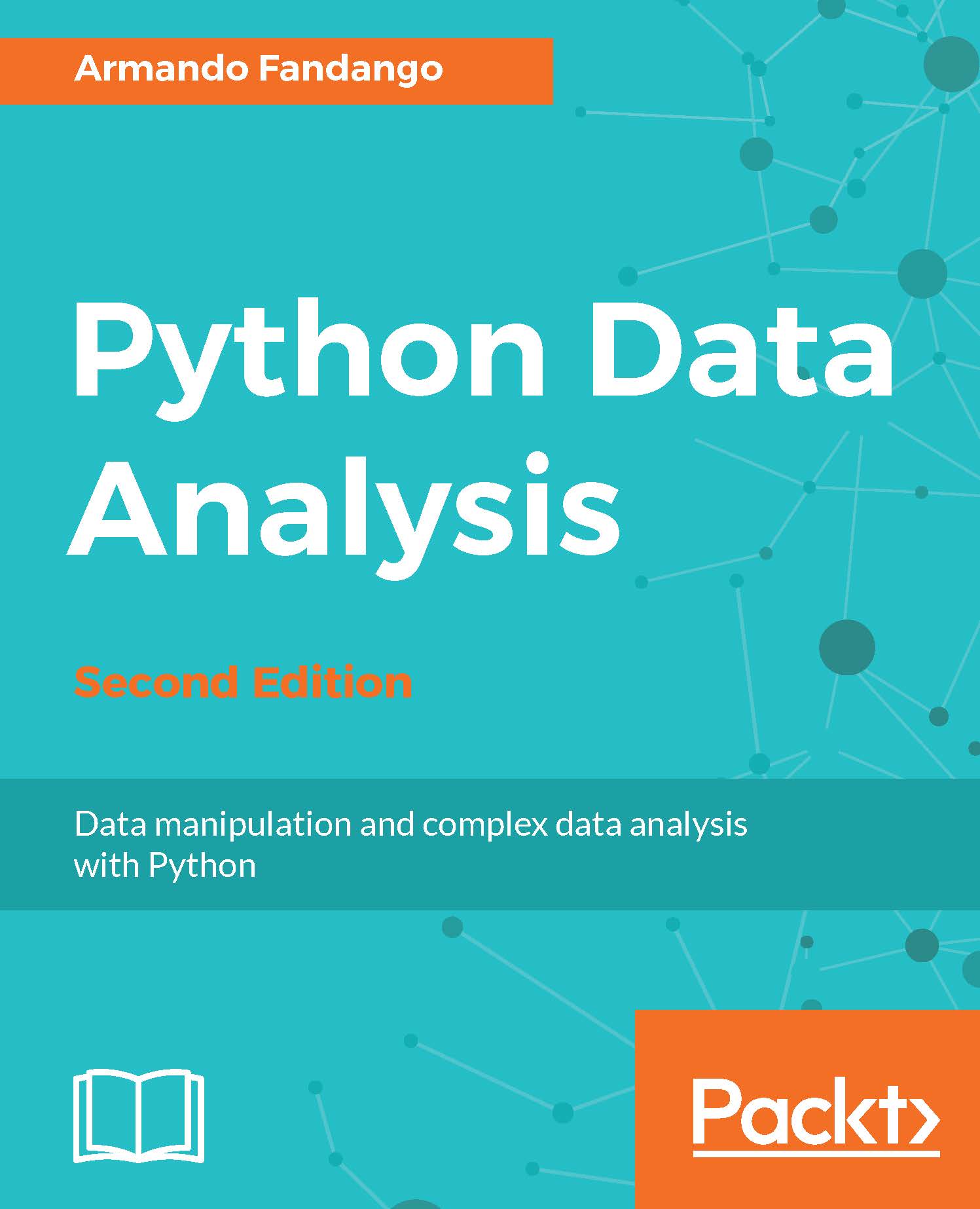
This is the most controversial section in the book so far. Genetic algorithms are based on the biological theory of evolution (see http://en.wikipedia.org/wiki/Evolutionary_algorithm). This type of algorithm is useful for searching and optimization. For instance, we can use it to find the optimal parameters for a regression or classification problem.
Humans and other life forms on Earth carry genetic information in chromosomes. Chromosomes are frequently modeled as strings. A similar representation is used in genetic algorithms. The first step is to initialize the population with random individuals and related representations of genetic information. We can also initialize with already-known candidate solutions for the problem. After that, we go through many iterations, called generations. During each generation, individuals are selected for mating based on a predefined fitness function. The fitness function evaluates how close an individual is to the desired solution.
Two...