-
Book Overview & Buying
-
Table Of Contents
-
Feedback & Rating
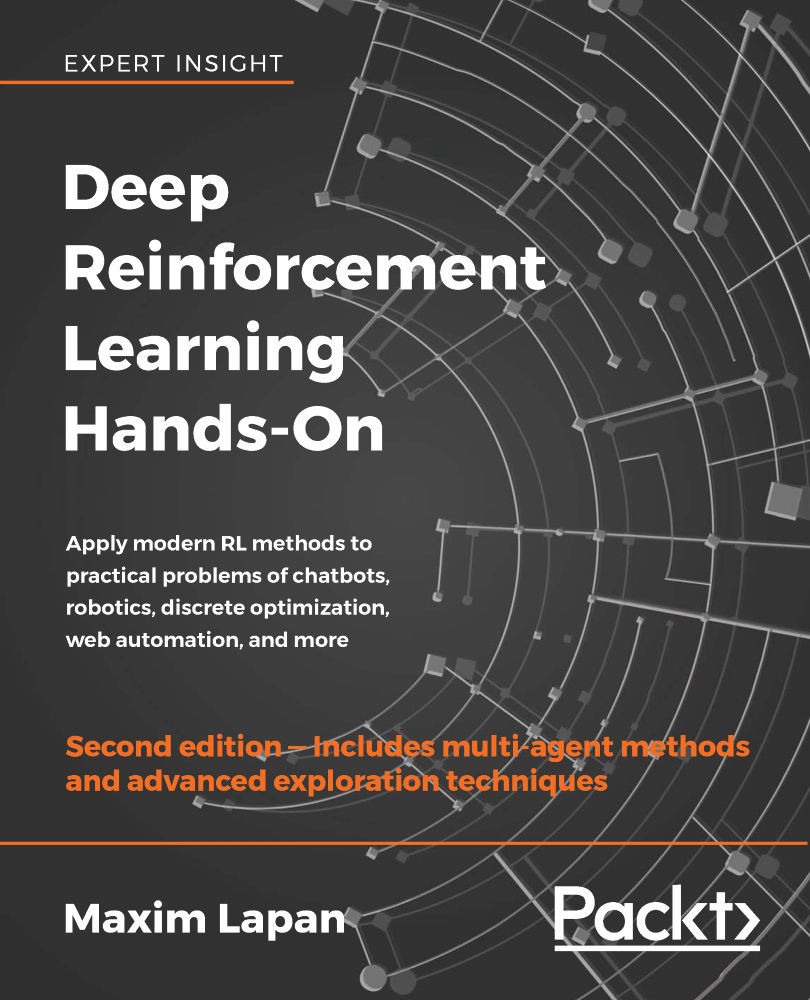
Deep Reinforcement Learning Hands-On
By :
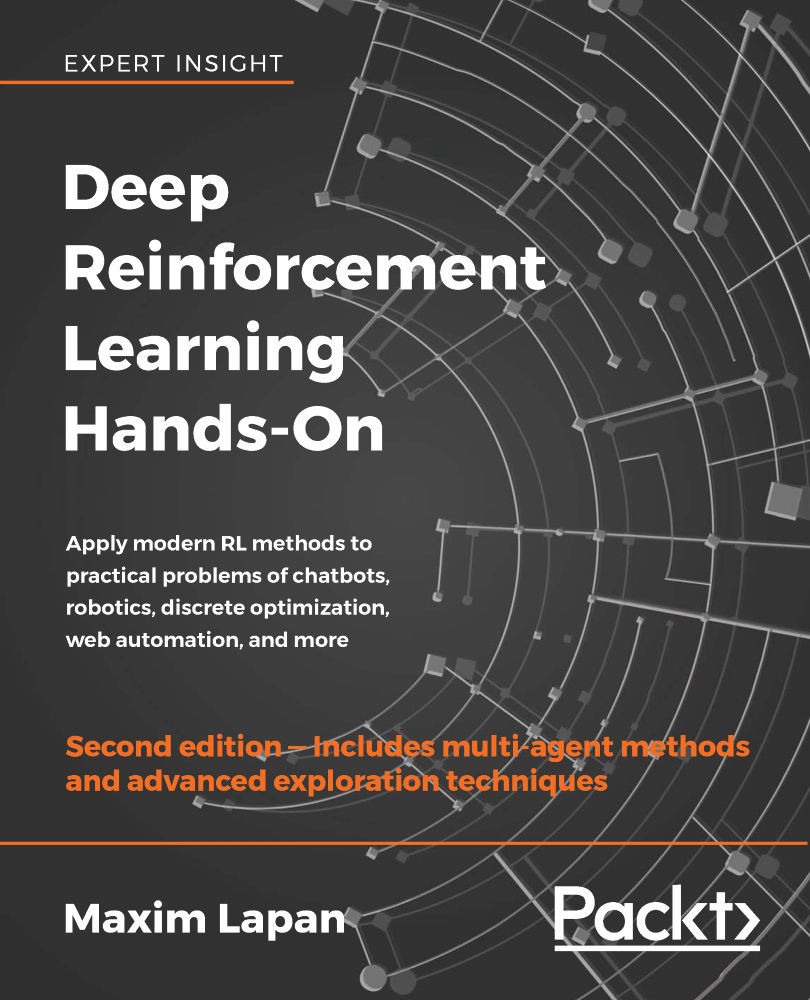
The next environment that we will try to solve using the cross-entropy method is FrozenLake. Its world is from the so-called grid world category, when your agent lives in a grid of size 4×4 and can move in four directions: up, down, left, and right. The agent always starts at a top-left position, and its goal is to reach the bottom-right cell of the grid. There are holes in the fixed cells of the grid and if you get into those holes, the episode ends and your reward is zero. If the agent reaches the destination cell, then it obtains a reward of 1.0 and the episode ends.
To make life more complicated, the world is slippery (it's a frozen lake after all), so the agent's actions do not always turn out as expected—there is a 33% chance that it will slip to the right or to the left. If you want the agent to move left, for example, there is a 33% probability that it will, indeed, move left, a 33% chance that it will end up in...