-
Book Overview & Buying
-
Table Of Contents
-
Feedback & Rating
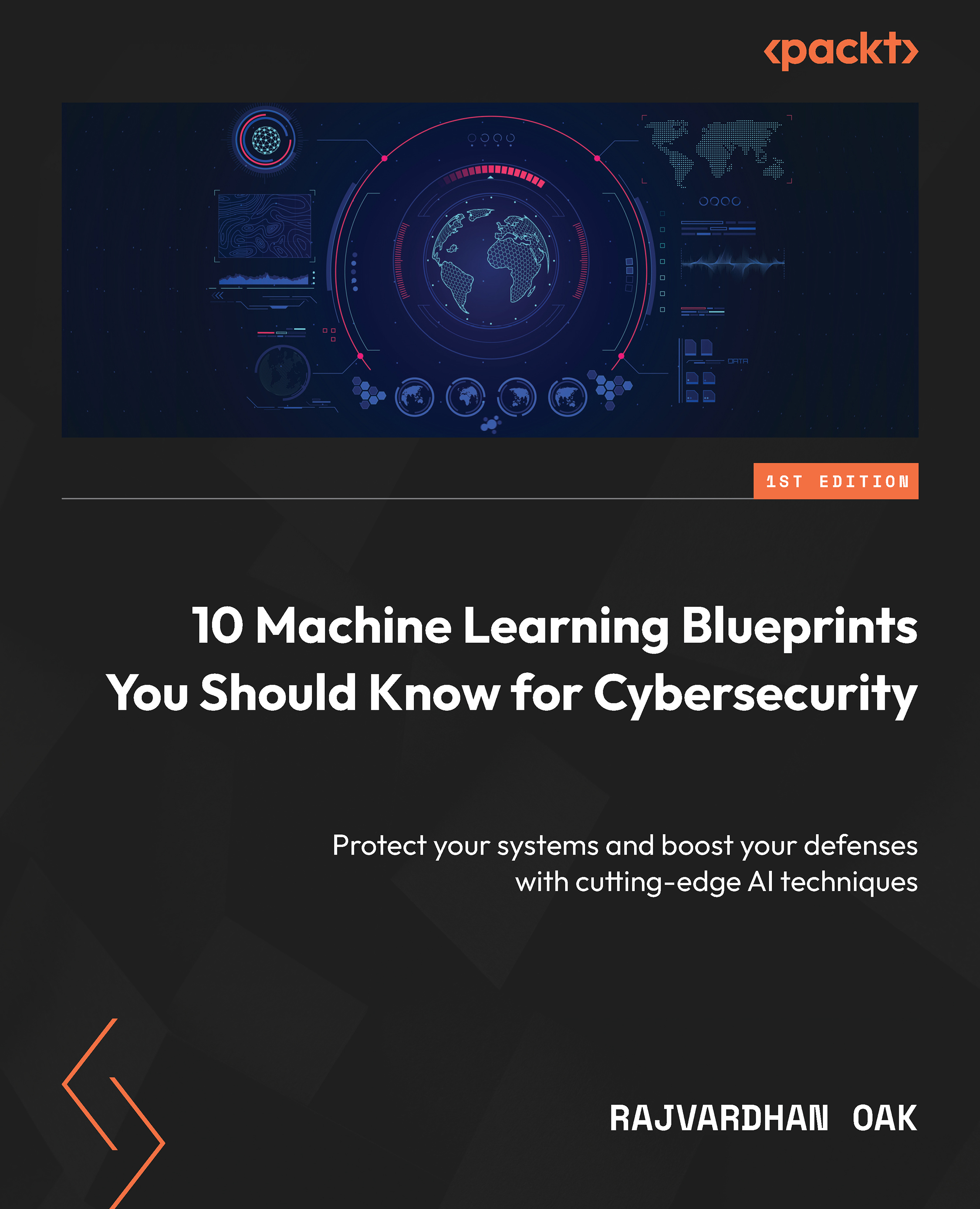
10 Machine Learning Blueprints You Should Know for Cybersecurity
By :
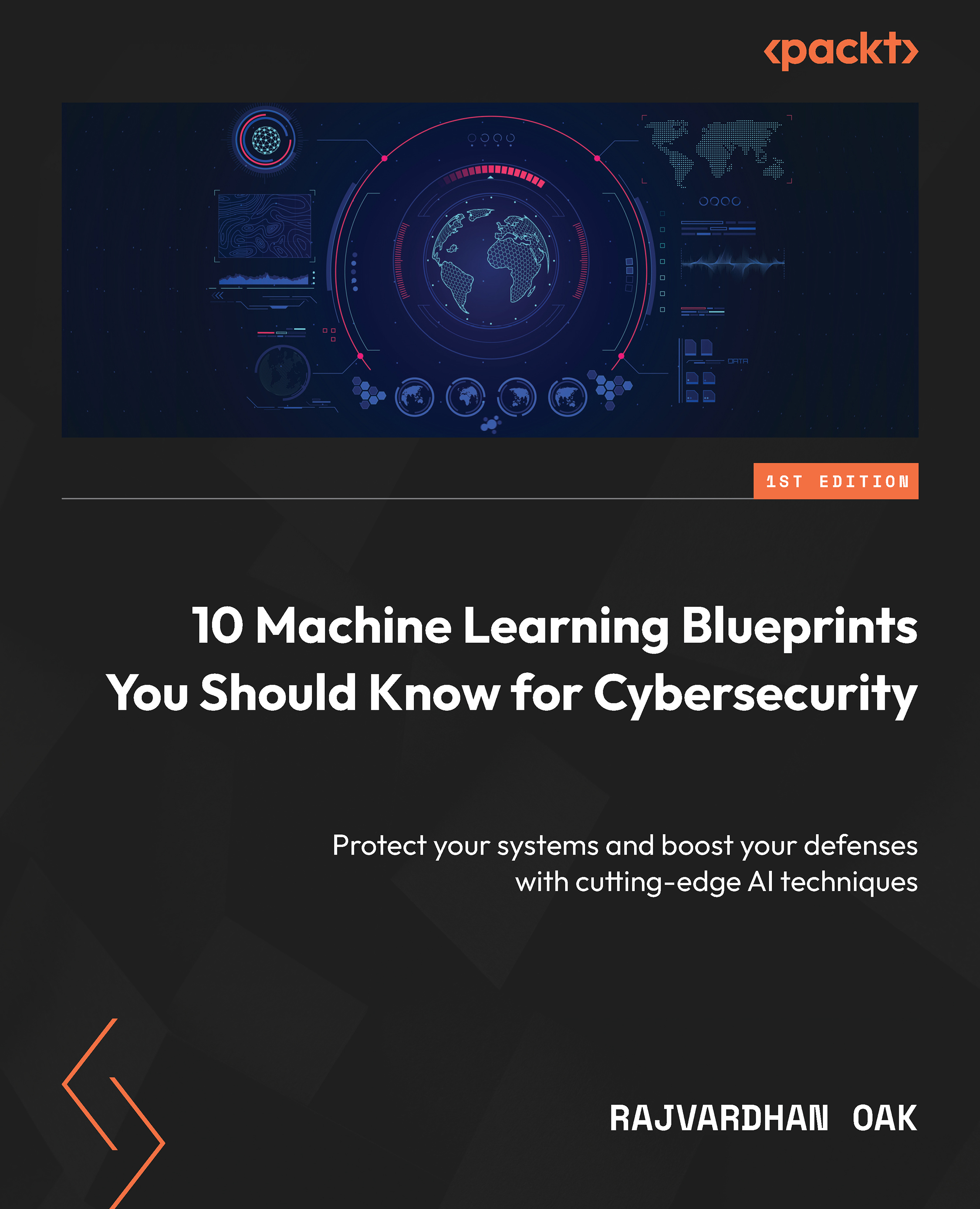
Please note that this section contains examples of hate speech and racist content online.
Just as with images, text models are also susceptible to adversarial attacks. Attackers can modify the text so as to trigger a misclassification by ML models. Doing so can allow an adversary to escape detection.
A good example of this can be seen on social media platforms. Most platforms have rules against abusive language and hate speech. Automated systems such as keyword-based filters and ML models are used to detect such content, flag it, and remove it. If something outrageous is posted, the platform will block it at the source (that is, not allow it to be posted at all) or remove it in the span of a few minutes.
A malicious adversary can purposely manipulate the content in order to fool a model into thinking that the words are out of vocabulary or are not certain abusive words. For example, according to a study (Poster | Proceedings of the 2019 ACM SIGSAC Conference...