-
Book Overview & Buying
-
Table Of Contents
-
Feedback & Rating
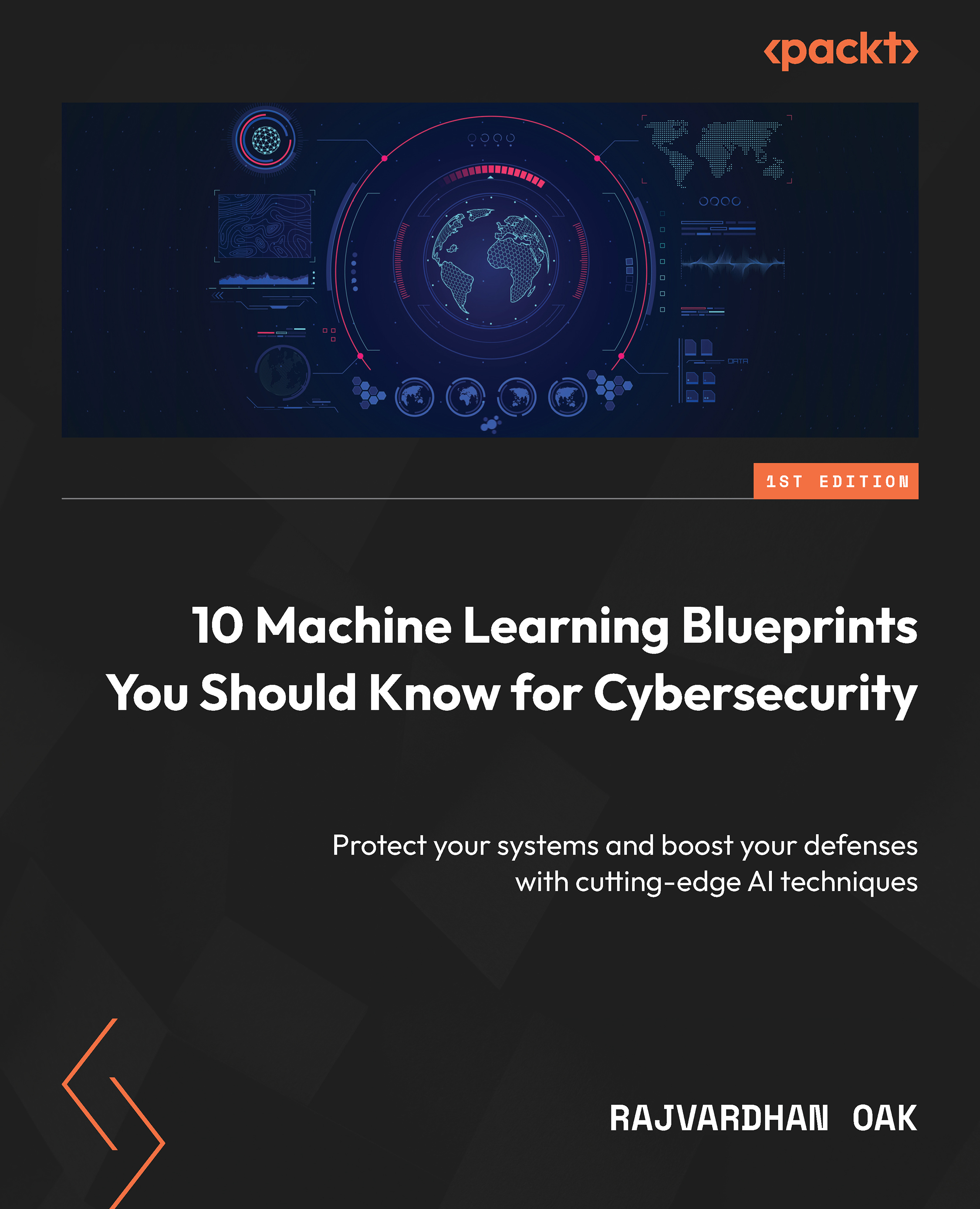
10 Machine Learning Blueprints You Should Know for Cybersecurity
By :
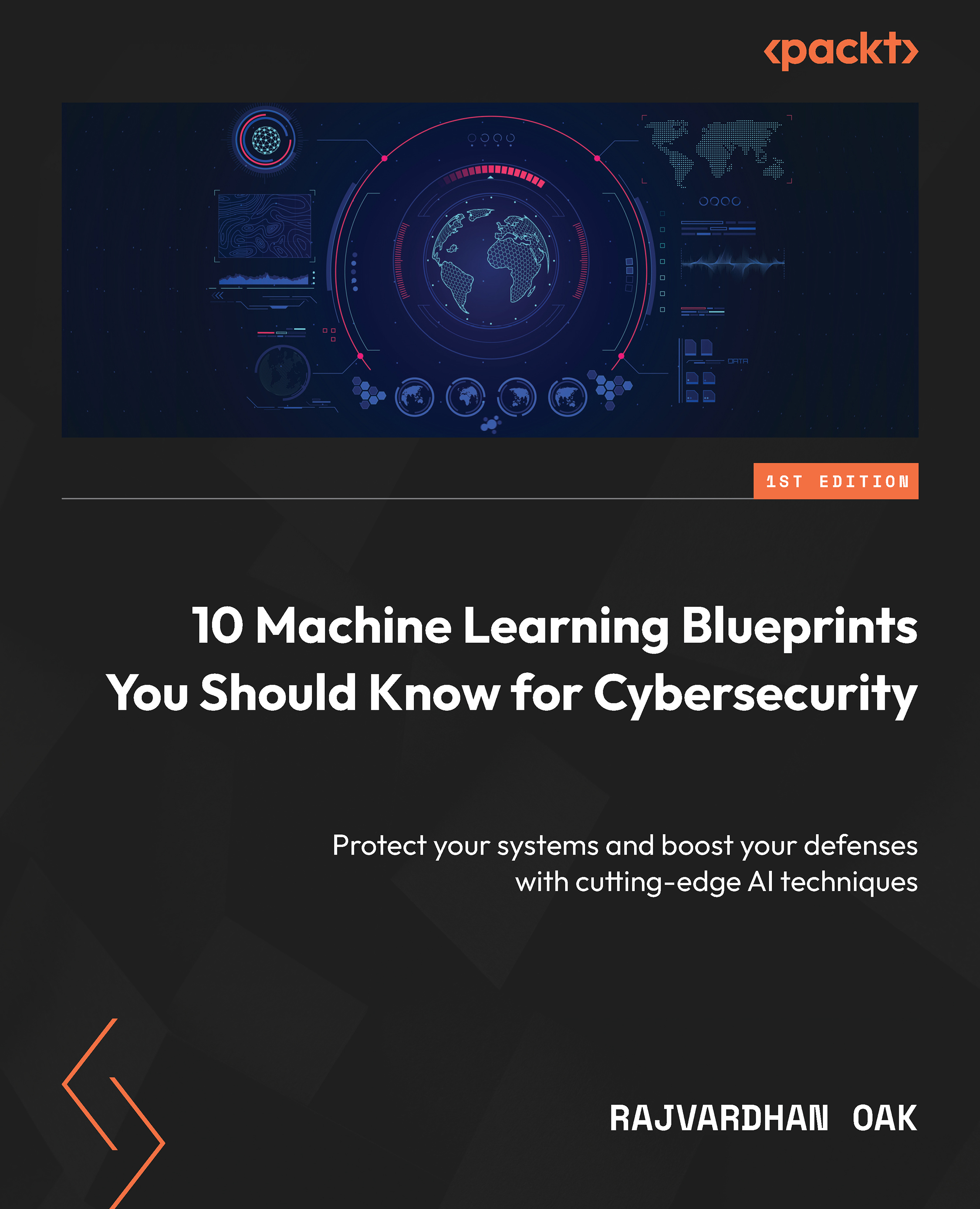
In this section, we will cover the basics of differential privacy, including the mathematical definition and a real-world example.
Differential privacy (DP) is a framework for preserving the privacy of individuals in a dataset when it is used for statistical analysis or machine learning. The goal of DP is to ensure that the output of a computation on a dataset does not reveal sensitive information about any individual in the dataset. This is accomplished by adding controlled noise to the computation in order to mask the contribution of any individual data point.
DP provides a mathematically rigorous definition of privacy protection by quantifying the amount of information that an attacker can learn about an individual by observing the output of a computation. Specifically, DP requires that the probability of observing a particular output from a computation is roughly the same whether a particular individual is included in...