-
Book Overview & Buying
-
Table Of Contents
-
Feedback & Rating
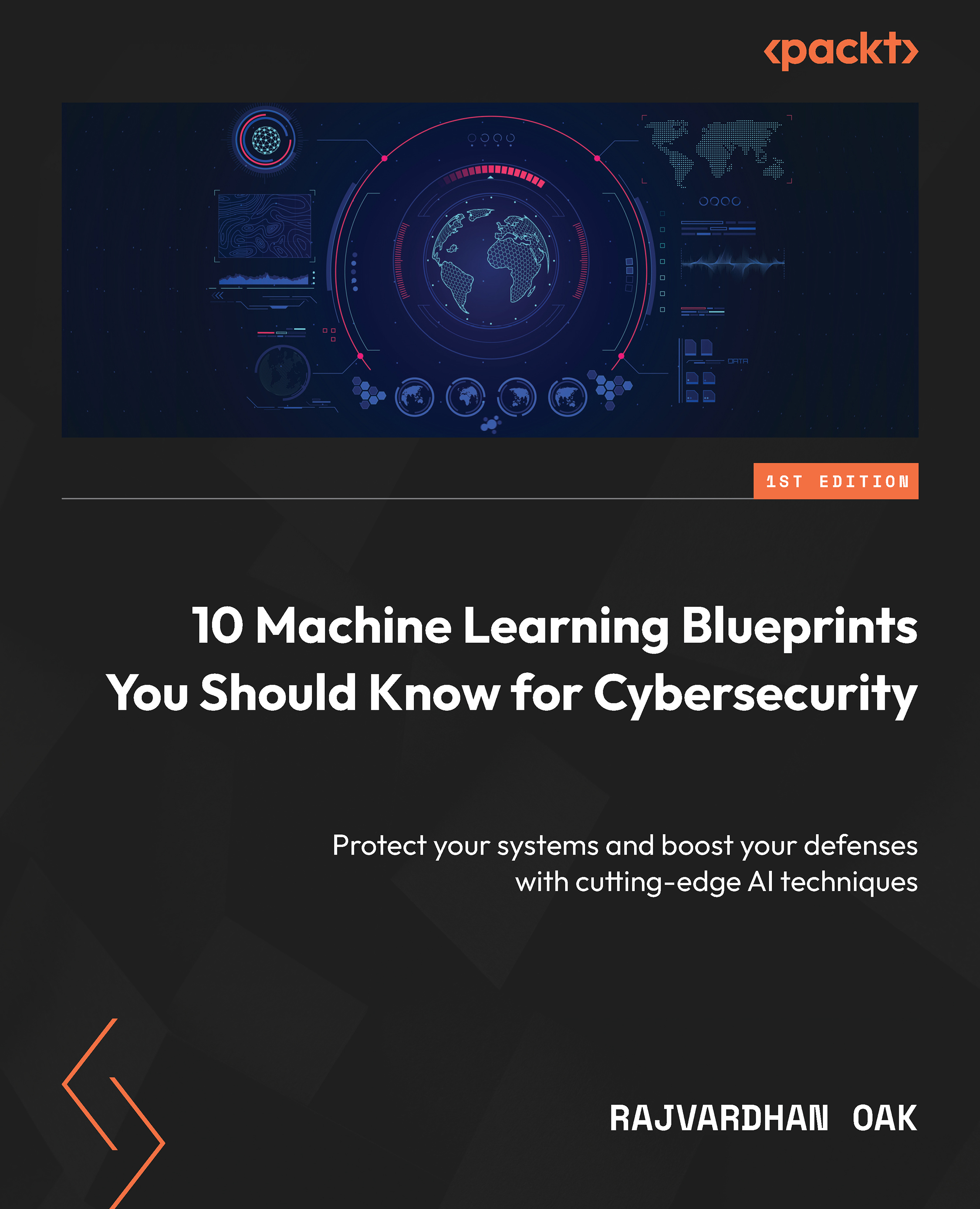
10 Machine Learning Blueprints You Should Know for Cybersecurity
By :
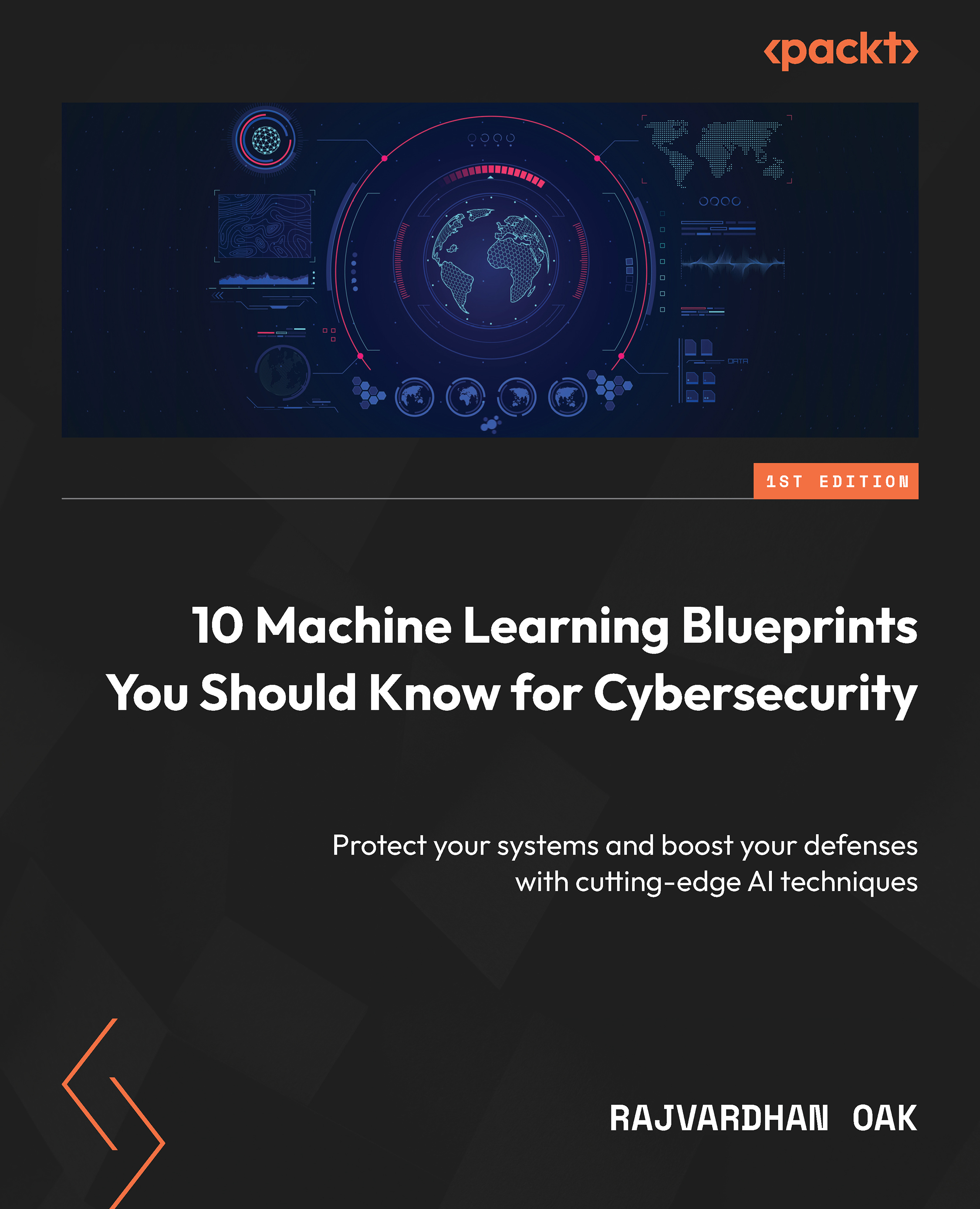
Many problems in cybersecurity are constructed as anomaly detection tasks, as attacker behavior is generally deviant from good actor behavior. An anomaly is anything that is out of the ordinary—an event that doesn’t fit in with normal behavior and hence is considered suspicious. For example, if a person has been consistently using their credit card in Bangalore, a transaction using the same card in Paris might be an anomaly. If a website receives roughly 10,000 visits every day, a day when it receives 2 million visits might be anomalous.
Anomalies are few and rare and indicate behavior that is strange and suspicious. Anomaly detection algorithms are unsupervised; we do not have labeled data to train a model. We learn what the normal expected behavior is and flag anything that deviates from it as abnormal. Because labeled data is very rarely available in security-related areas, anomaly detection methods are crucial in identifying attacks, fraud, and intrusions.
In this chapter, we will cover the following main topics:
By the end of this chapter, you will know how to detect outliers and anomalies using statistical and ML methods.