-
Book Overview & Buying
-
Table Of Contents
-
Feedback & Rating
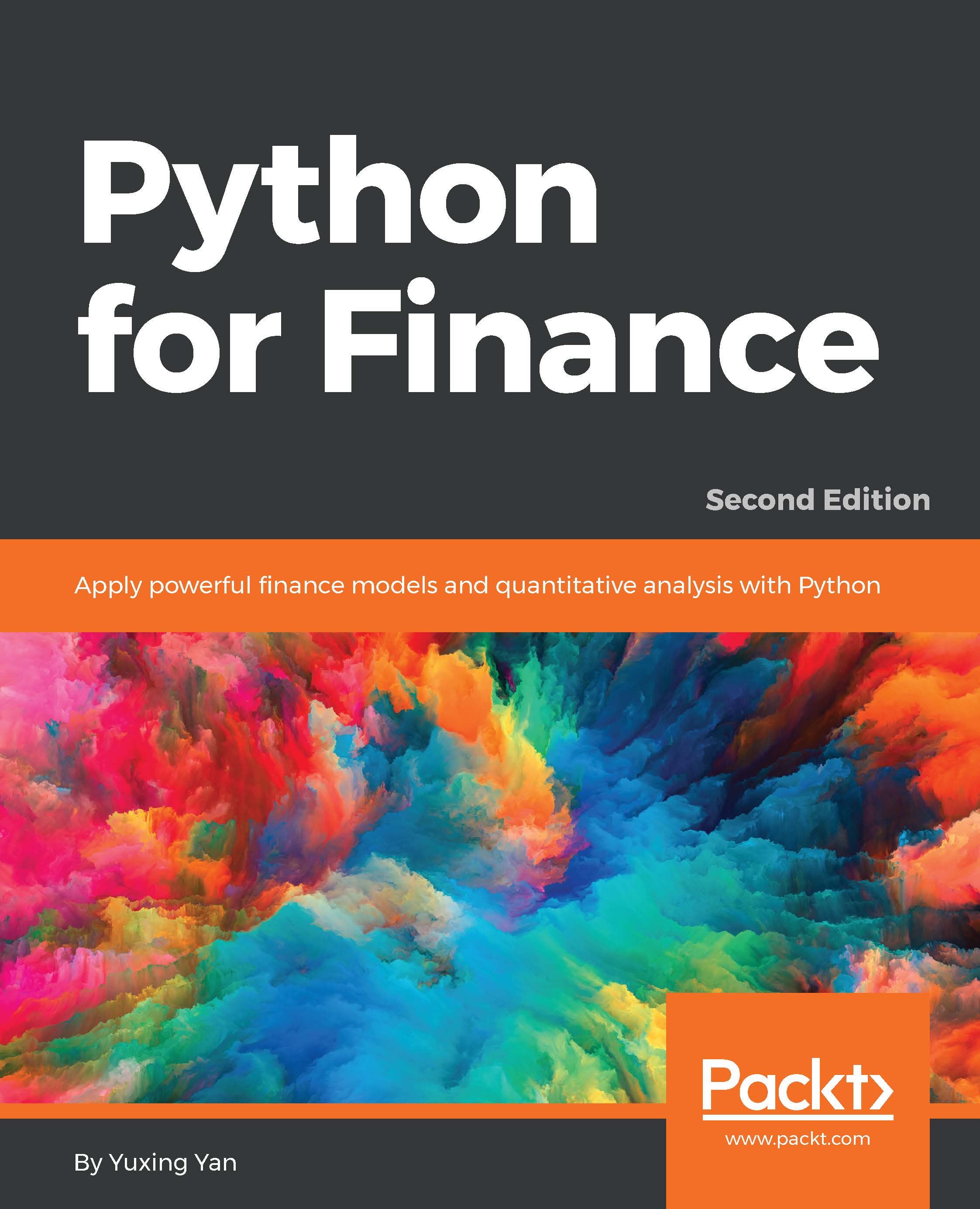
Python for Finance
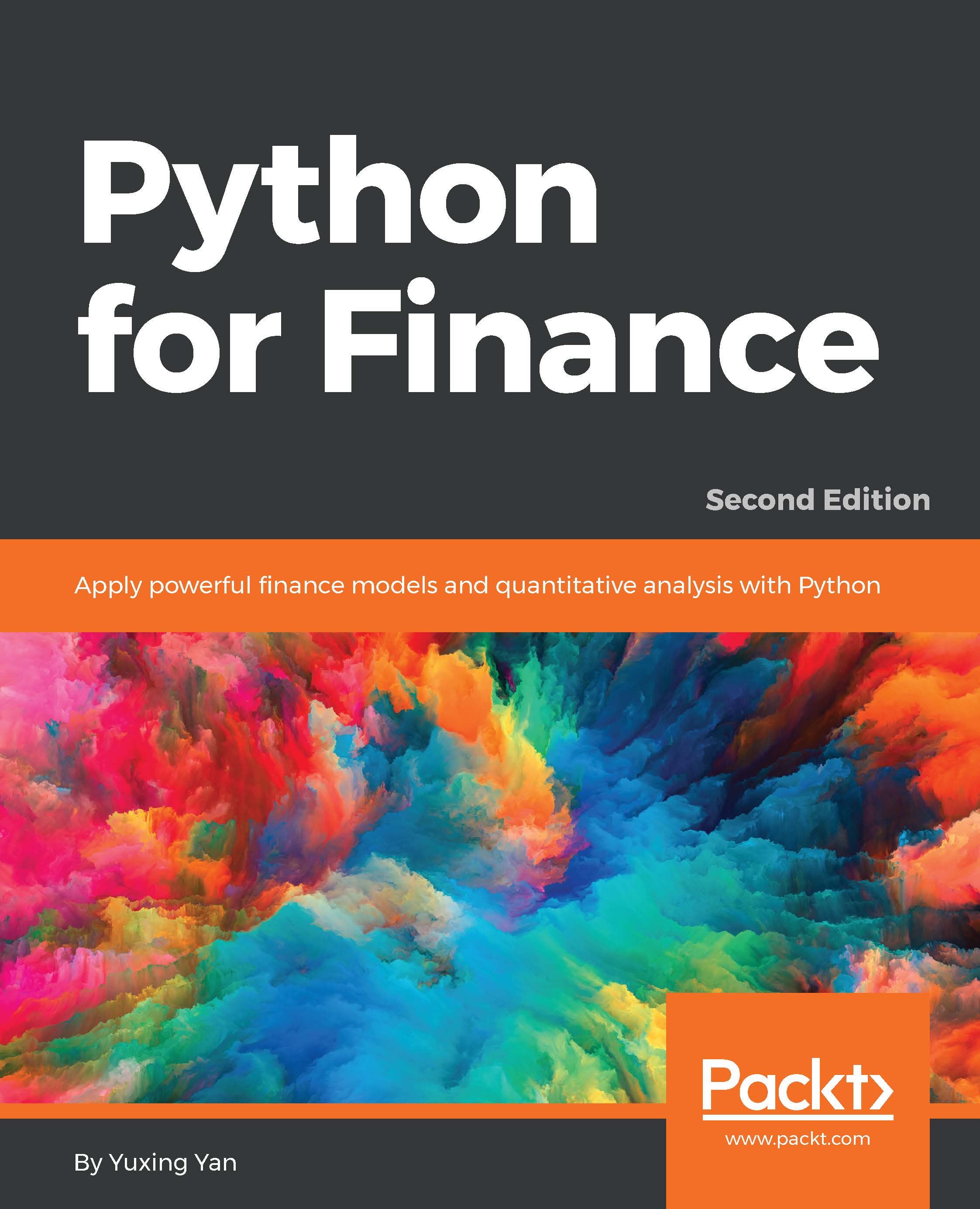
Python for Finance
Overview of this book
This book uses Python as its computational tool. Since Python is free, any school or
organization can download and use it.
This book is organized according to various finance subjects. In other words, the first edition focuses more on Python, while the second edition is truly trying to apply Python to finance.
The book starts by explaining topics exclusively related to Python. Then we deal with critical parts of Python, explaining concepts such as time value of money stock and bond evaluations, capital asset pricing model, multi-factor models, time series analysis, portfolio theory,
options and futures.
This book will help us to learn or review the basics of quantitative finance and apply Python to solve various problems, such as estimating IBM’s market risk,
running a Fama-French 3-factor, 5-factor, or Fama-French-Carhart 4 factor model, estimating the VaR of a 5-stock portfolio, estimating the optimal portfolio, and constructing the efficient frontier for a 20-stock portfolio with real-world stock, and with Monte Carlo Simulation. Later, we will also learn how to replicate the famous Black-Scholes-Merton option model and how to price exotic options such as the average price call option.
Table of Contents (17 chapters)
Preface
1. Python Basics
2. Introduction to Python Modules
3. Time Value of Money
4. Sources of Data
5. Bond and Stock Valuation
6. Capital Asset Pricing Model
7. Multifactor Models and Performance Measures
8. Time-Series Analysis
9. Portfolio Theory
10. Options and Futures
11. Value at Risk
12. Monte Carlo Simulation
13. Credit Risk Analysis
14. Exotic Options
15. Volatility, Implied Volatility, ARCH, and GARCH
Index
Customer Reviews