-
Book Overview & Buying
-
Table Of Contents
-
Feedback & Rating
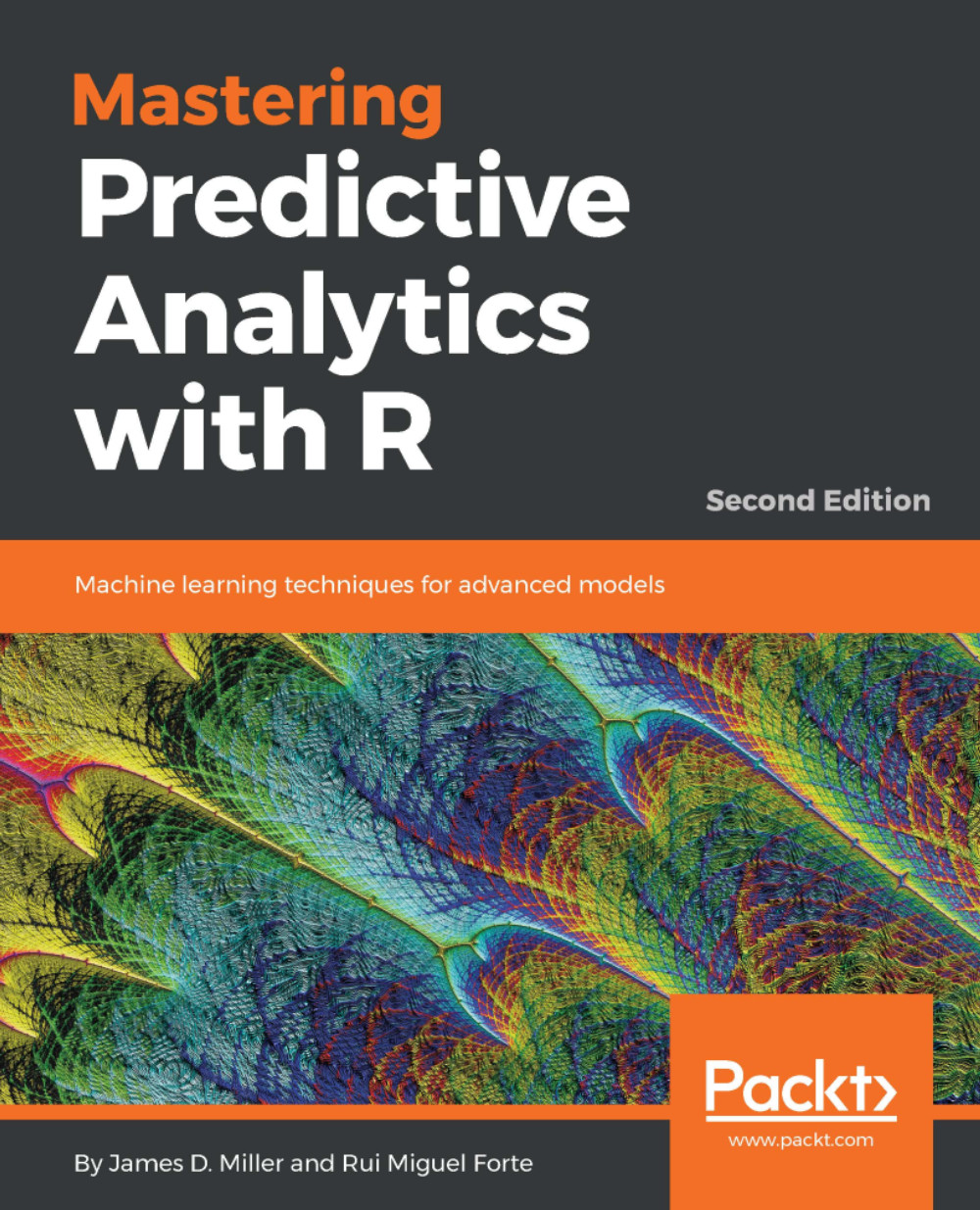
Mastering Predictive Analytics with R, Second Edition
By :
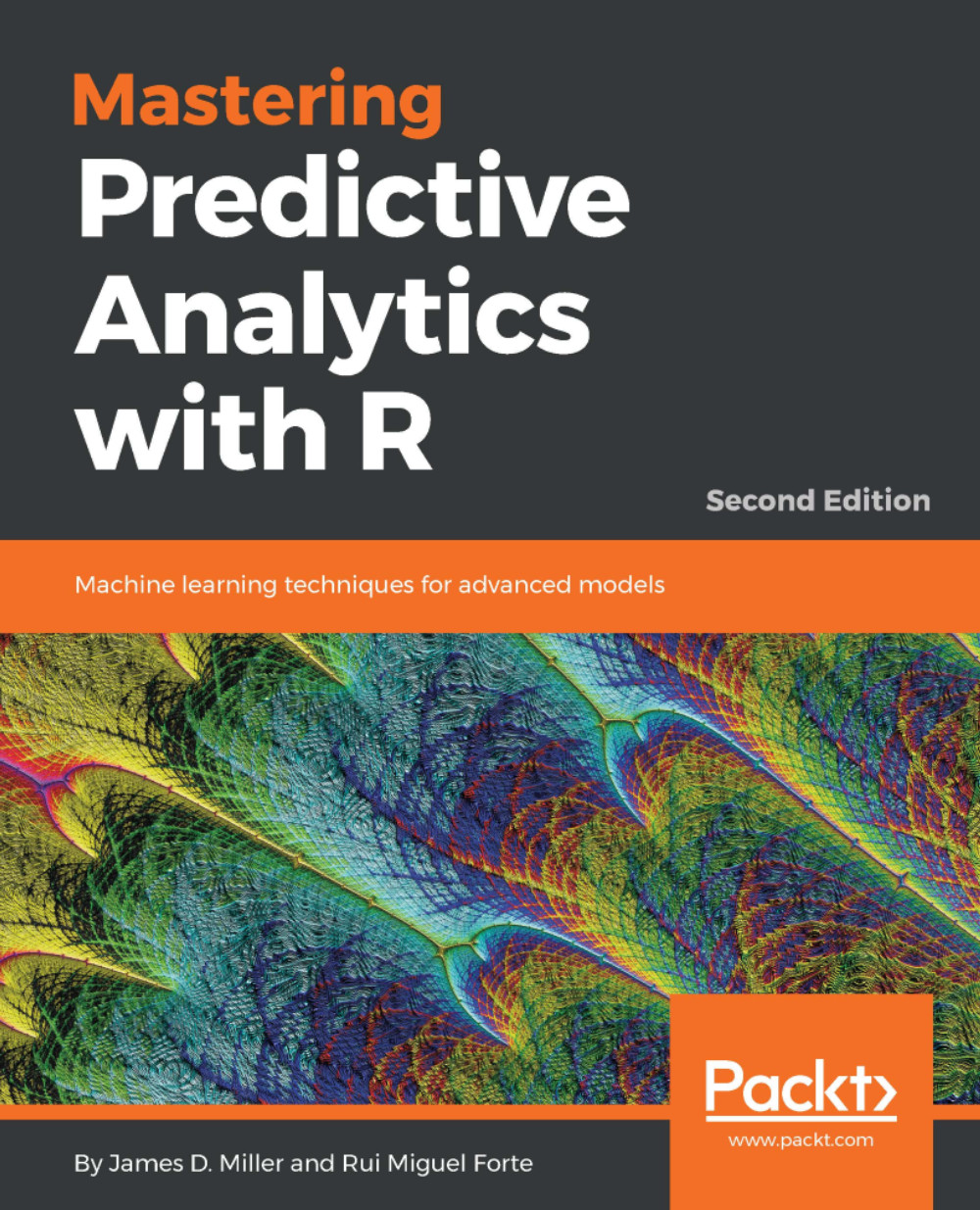
In this chapter, we discussed the topics of machine and deep learning and the difference between the two. We also mentioned how deep learning has the capacity to drive change in the world.
We saw how deep learning reduces the effort required by humans and listed some of the current applications where these algorithms have been successfully applied. We then looked at using word embedding for a use case such as NLP applications, and explained how it works.
Finally, we wrapped up with a discussion on neural networks, specifically RNNs.
With this chapter, we bring our journey to its end, having provided in-depth information around performance metrics and learning curves, polynomial regression, Poisson, and negative binomial regression, back-propagation, radial basis function networks, and others. We also discussed the process of working with very large datasets.
Hopefully you have enjoyed exploring and testing these popular modeling techniques and mastered a range of predictive analytics...