-
Book Overview & Buying
-
Table Of Contents
-
Feedback & Rating
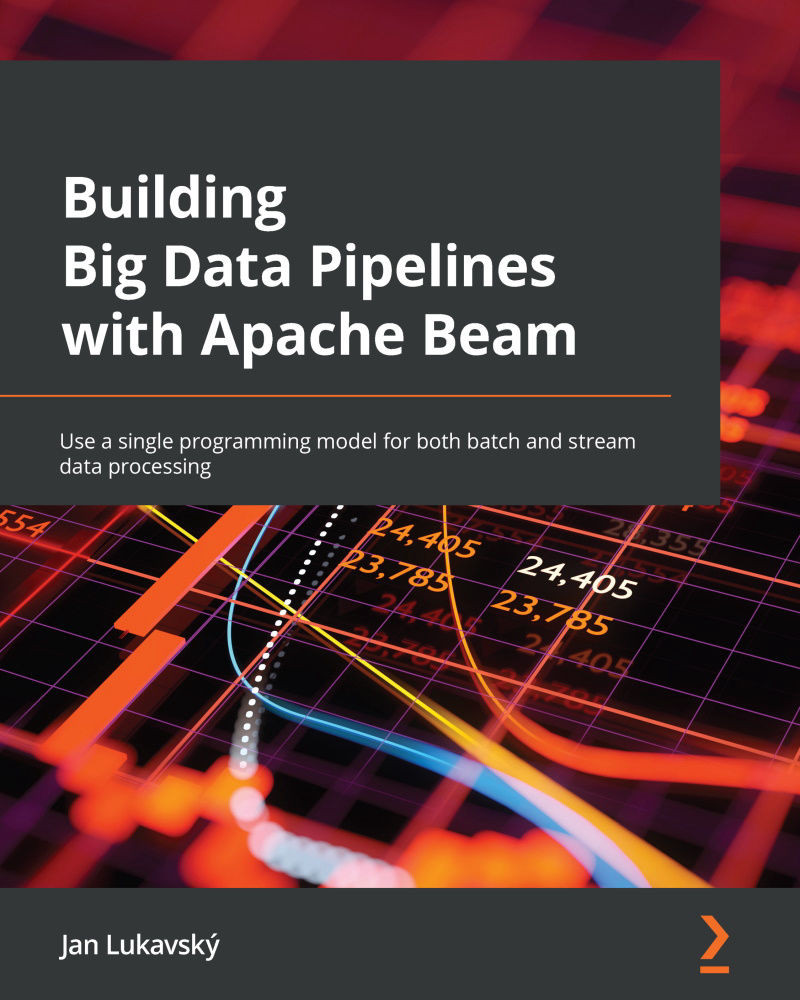
Building Big Data Pipelines with Apache Beam
By :
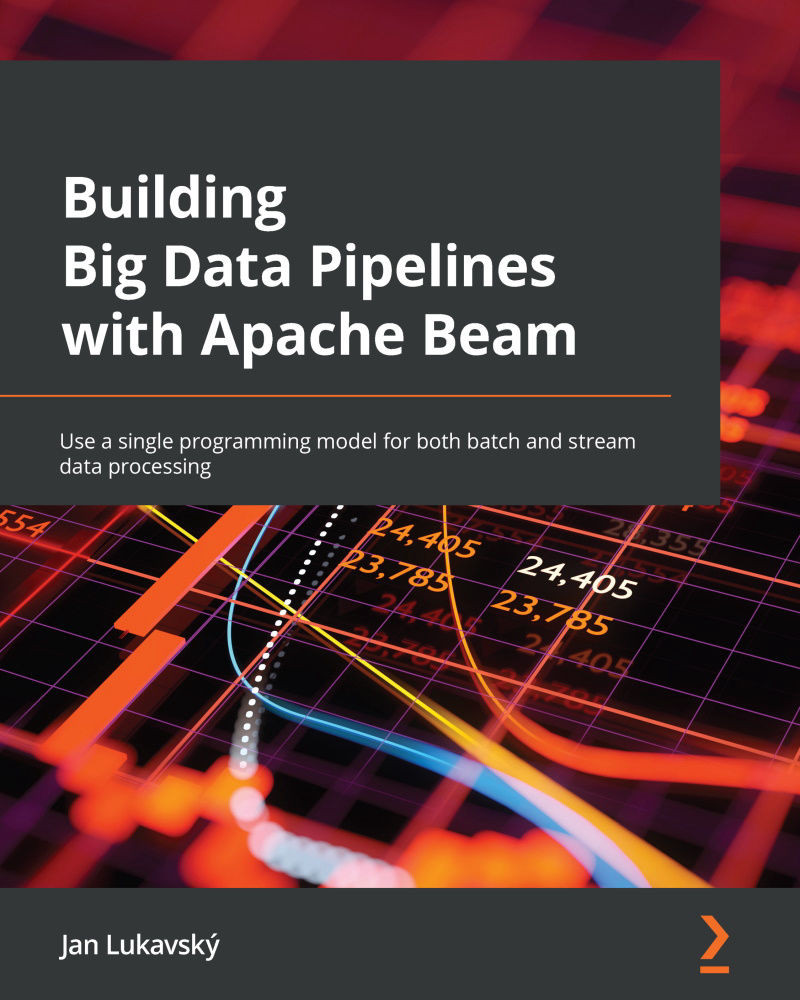
Building Big Data Pipelines with Apache Beam
By:
Overview of this book
Apache Beam is an open source unified programming model for implementing and executing data processing pipelines, including Extract, Transform, and Load (ETL), batch, and stream processing.
This book will help you to confidently build data processing pipelines with Apache Beam. You’ll start with an overview of Apache Beam and understand how to use it to implement basic pipelines. You’ll also learn how to test and run the pipelines efficiently. As you progress, you’ll explore how to structure your code for reusability and also use various Domain Specific Languages (DSLs). Later chapters will show you how to use schemas and query your data using (streaming) SQL. Finally, you’ll understand advanced Apache Beam concepts, such as implementing your own I/O connectors.
By the end of this book, you’ll have gained a deep understanding of the Apache Beam model and be able to apply it to solve problems.
Table of Contents (13 chapters)
Preface
Section 1 Apache Beam: Essentials
Chapter 1: Introduction to Data Processing with Apache Beam
Chapter 2: Implementing, Testing, and Deploying Basic Pipelines
Chapter 3: Implementing Pipelines Using Stateful Processing
Section 2 Apache Beam: Toward Improving Usability
Chapter 4: Structuring Code for Reusability
Chapter 5: Using SQL for Pipeline Implementation
Chapter 6: Using Your Preferred Language with Portability
Section 3 Apache Beam: Advanced Concepts
Chapter 7: Extending Apache Beam's I/O Connectors
Chapter 8: Understanding How Runners Execute Pipelines
Other Books You May Enjoy
Customer Reviews