-
Book Overview & Buying
-
Table Of Contents
-
Feedback & Rating
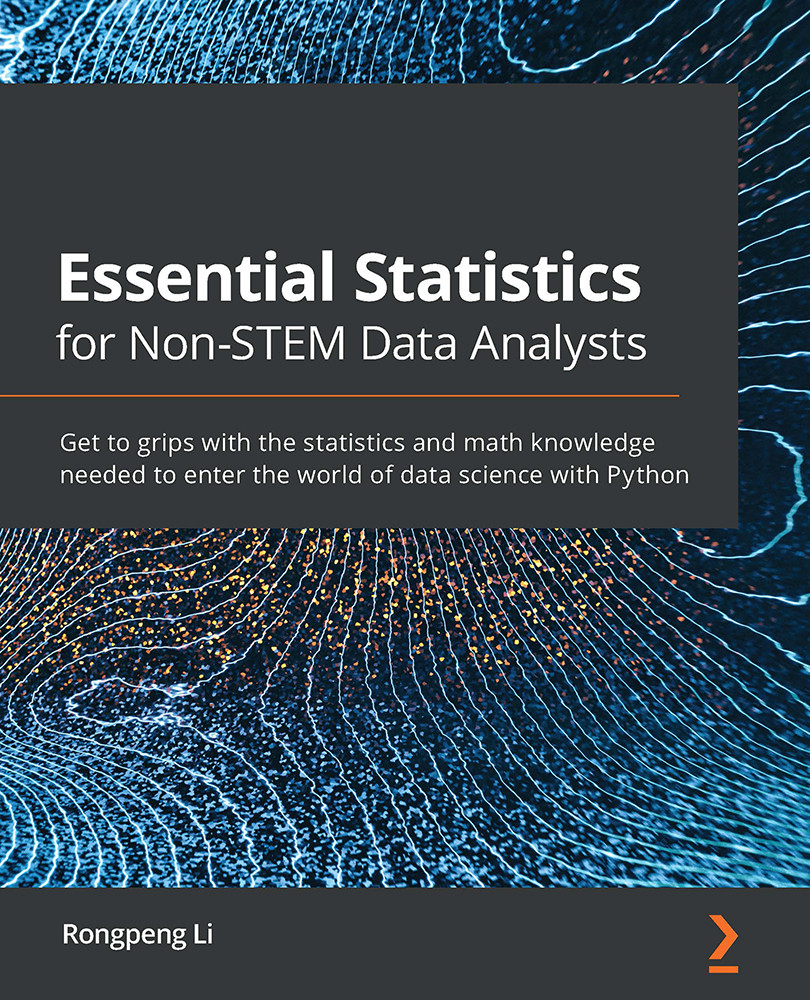
Essential Statistics for Non-STEM Data Analysts
By :
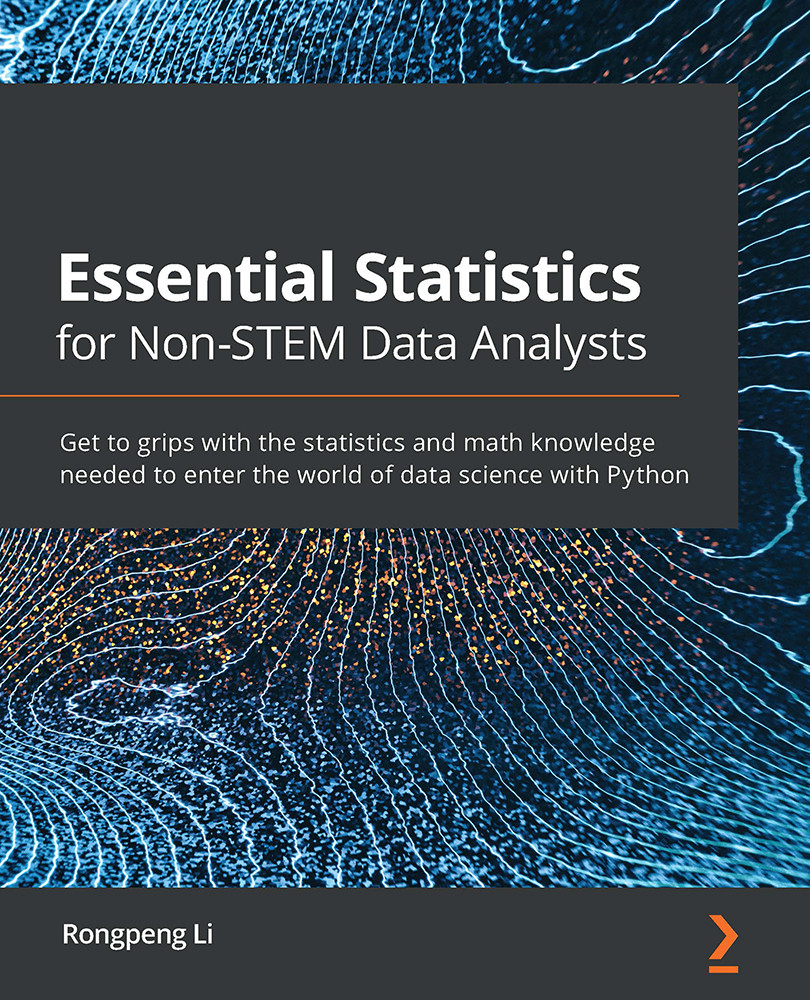
In this chapter, we will start with the most basic functionalities of the Matplotlib package. Let's first understand the elements to make a perfect statistical graph.
Before we dive into Python code, l will give you an overview of how to decompose the components of a statistical graph. I personally think the philosophy that embeds the R ggplot2
package is very concise and clear.
Note
R is another famous programming language for data science and statistical analysis. There are also successful R packages. The counterpart of Matplotlib is the R ggplot2
package mentioned previously.
ggplot2
is a very successful visualization tool developed by Hadley Wickman. It decomposes a statistical plot into the following three components: