-
Book Overview & Buying
-
Table Of Contents
-
Feedback & Rating
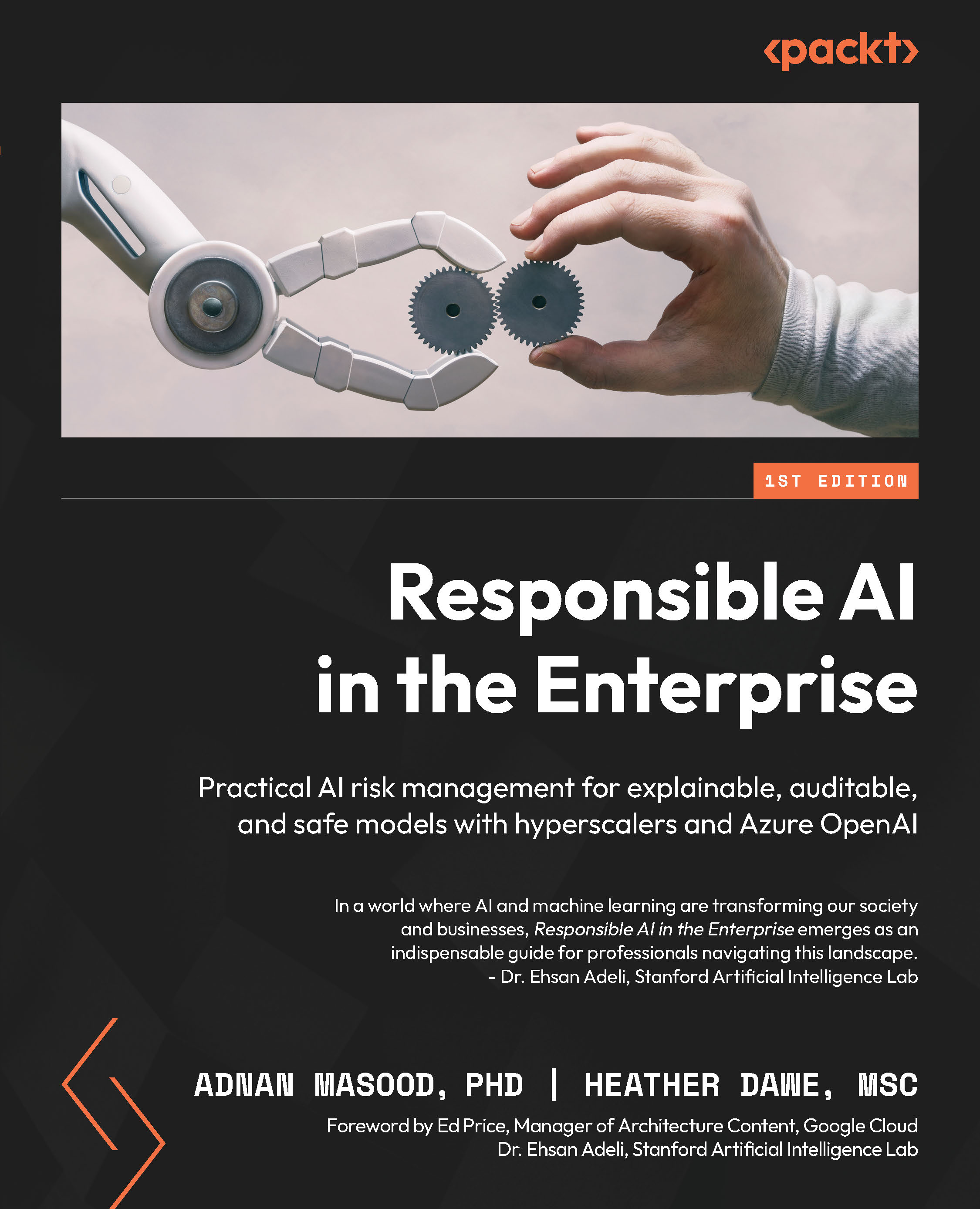
Responsible AI in the Enterprise
By :
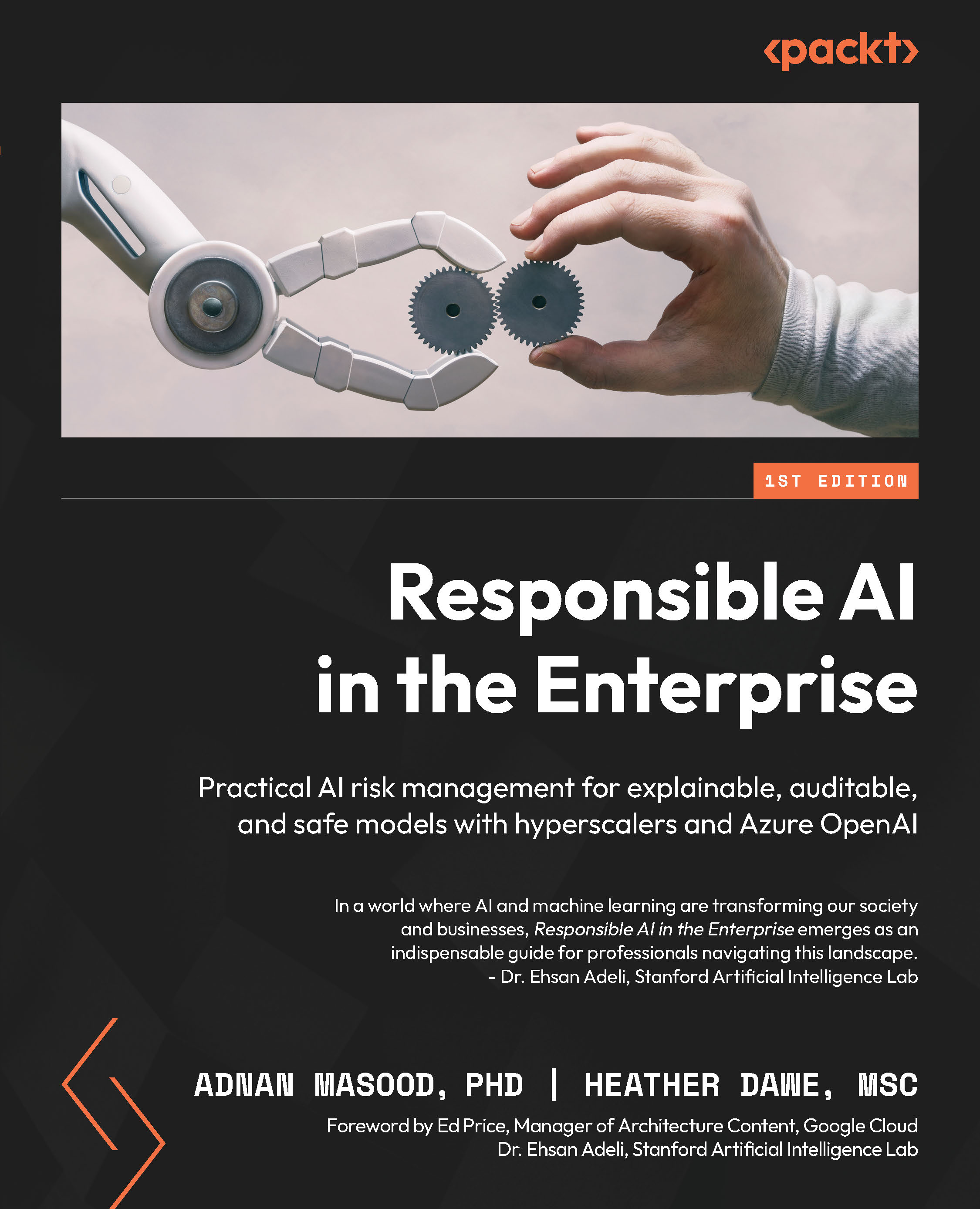
“Starting Jan 1st 2029, all manual, and semi-autonomous operating vehicles on highways will be prohibited. This restriction is in addition to pedestrians, bicycles, motorized bicycles, and non-motorized vehicle traffic. Only fully autonomous land vehicles compliant with intelligent traffic grid are allowed on the highways.”
– Hill Valley Telegraph, June 2028
Does this headline look very futuristic? Probably a decade ago, but today, you could see this as a reality in 5 to 10 years. With the current speed of automation, humans behind the wheel of vehicles weighing thousands of pounds would sound irresponsible in the next 10 years. Human driving will quickly become a novelty sport, as thousands of needless vehicle crash deaths caused by human mistakes can be avoided, thanks to self-driving vehicles.
Figure 1.1: The upper row shows an image from the validation set of Cityscapes and its prediction. The lower row shows the image perturbed with universal adversarial noise and the resulting prediction. Image Courtesy Metzen et al – Universal Adversarial Perturbations Against Semantic Image Segmentation – source: https://arxiv.org/pdf/1704.05712.pdf
As we race toward delegating decision-making to algorithms, we need to ask ourselves whether we have the capability to clearly understand and justify how an AI model works and predicts. It might not be important to fully interpret how your next Netflix movie has been recommended, but when it comes to the critical areas of human concerns such as healthcare, recruitment, higher education admissions, legal, commercial aircraft collision avoidance, financial transactions, autonomous vehicles, or control of massive power generating or chemical manufacturing plants, these decisions are critical. It is pretty self-explanatory and logical that if we can understand what algorithms do, we can debug, improve, and build upon them easily. Therefore, we can extrapolate that in order to build an ethical AI – an AI that is congruent with our current interpretation of ethics – explainability would be one of the must-have features. Decision transparency, or understanding why an AI model predicts what it predicts, is critical to building a trustworthy and reliable AI system. In the preceding figure, you can see how an adversarial input can change the way an autonomous vehicle sees (or does not see) pedestrians. If there is an accident, an algorithm must be able to explain its action clearly in the state when the input was received – in an auditable, repeatable, and reproducible manner.
AI governance and model risk management are essential in today’s world, where AI is increasingly being used to make critical decisions that affect individuals and society as a whole. Without proper governance and risk management, AI systems could be biased, inaccurate, or unethical, leading to negative outcomes and loss of public trust. By ensuring that AI is developed, deployed, and used in a responsible and ethical manner, we can leverage its full potential to improve lives, advance research, and drive innovation. As AI researchers and practitioners, we have a responsibility to prioritize governance and risk management to create a better, more equitable future for everyone. This means that to have a safe, reliable, and trustworthy AI for human use, it must be safe, transparent, explainable, justifiable, robust, and ethical.
We have been using lots of big words, so let’s define what these terms really mean.
Change the font size
Change margin width
Change background colour