-
Book Overview & Buying
-
Table Of Contents
-
Feedback & Rating
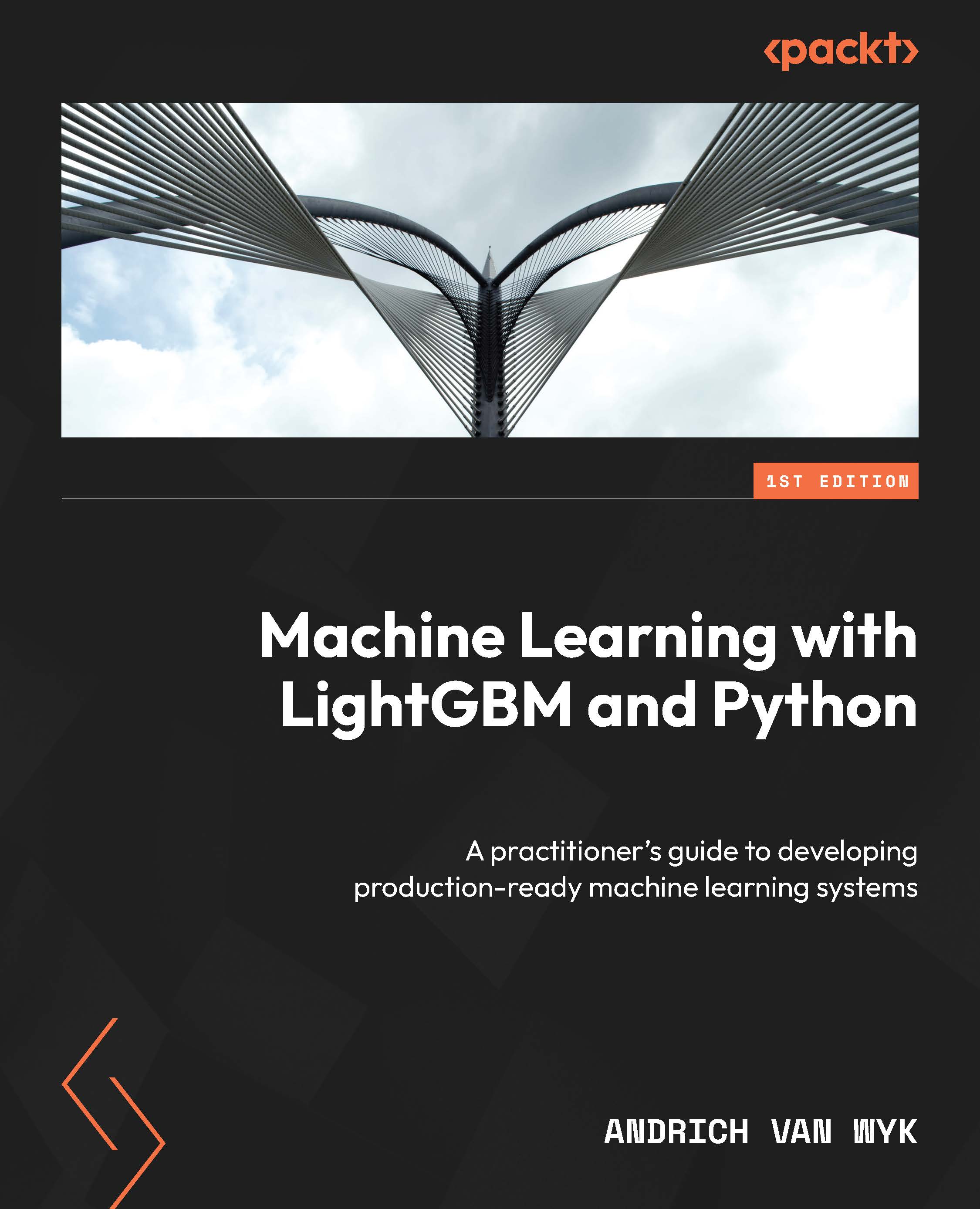
Machine Learning with LightGBM and Python
By :
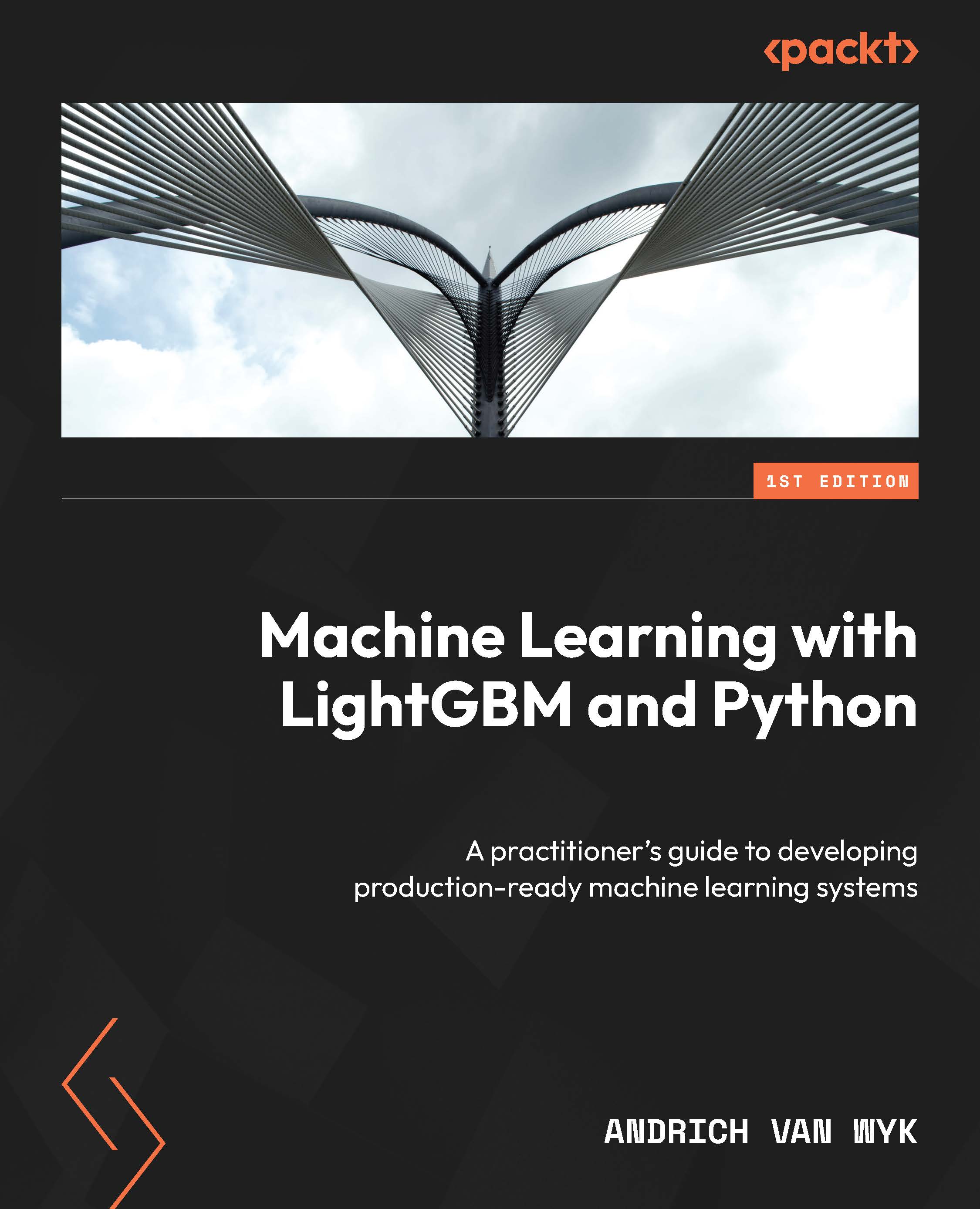
In summary, this chapter discussed AutoML systems and their uses. Typical approaches to automating feature engineering, model selection, and tuning were discussed. We also mentioned the risks and caveats associated with using these systems.
The chapter also introduced FLAML, a library for AutoML that provides tools for automating the model selection and tuning process. We also presented CFO and BlendSearch, two efficient hyperparameter optimization algorithms provided by FLAML.
The practicalities of applying FLAML were shown in the form of a case study. In addition to FLAML, we showcased an open source tool called Featuretools, which provides functionality to automate feature engineering. We showed how to develop optimized models in fixed-time budgets using FLAML. Finally, we provided examples of using FLAML’s zero-shot AutoML functionality, which analyzes datasets against configurations for known problems to determine suitable hyperparameters, eliminating the...