-
Book Overview & Buying
-
Table Of Contents
-
Feedback & Rating
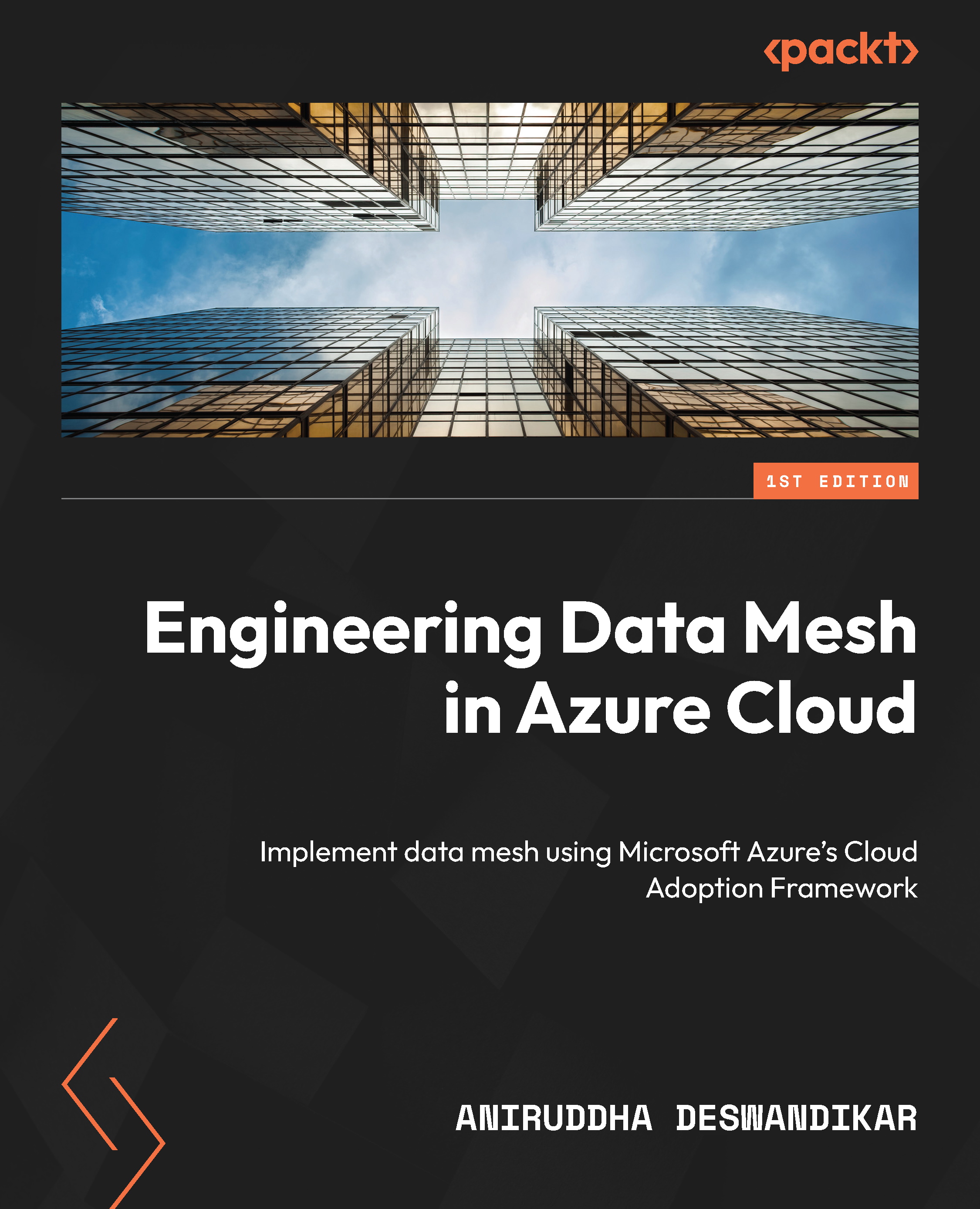
Engineering Data Mesh in Azure Cloud
By :
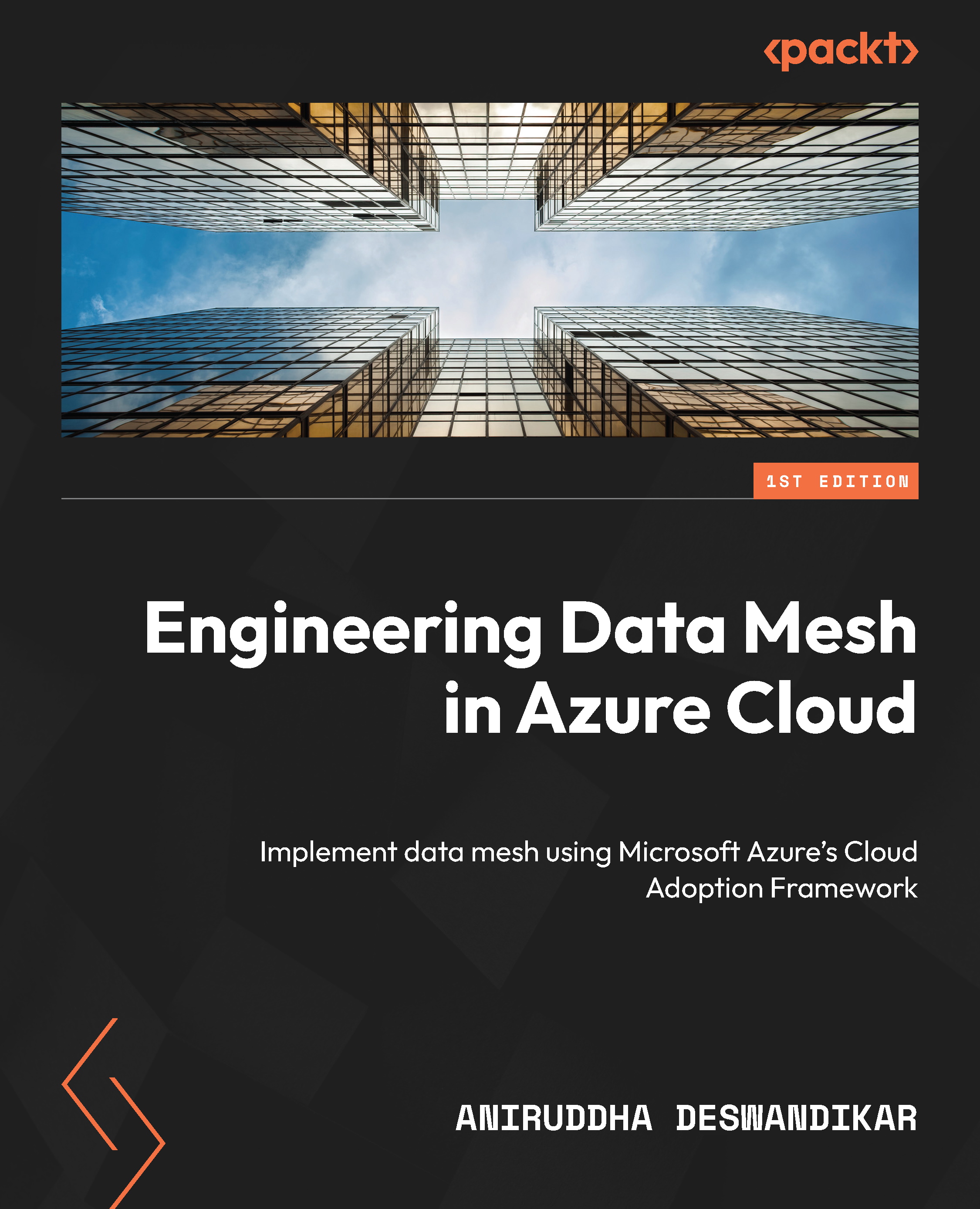
Build, buy, or both? In the previous section, we observed that data quality has many different aspects. It also is something that evolves over time. As you build your data mesh and as you onboard new projects, new data quality parameters need to be added. So, clearly, you need a solution that will change and grow with your requirements. If you build your data quality management system, you will not have to worry about this requirement. You will be able to change, modify, and upgrade the functionality whenever you need to.
If you build your own data quality engine, you will need the following components:
Figure 9.7 shows the components of a data quality management system:
Figure 9.7 – Components of DQMS architecture
The main disadvantages of building your own DQMS are the use of resources...