-
Book Overview & Buying
-
Table Of Contents
-
Feedback & Rating
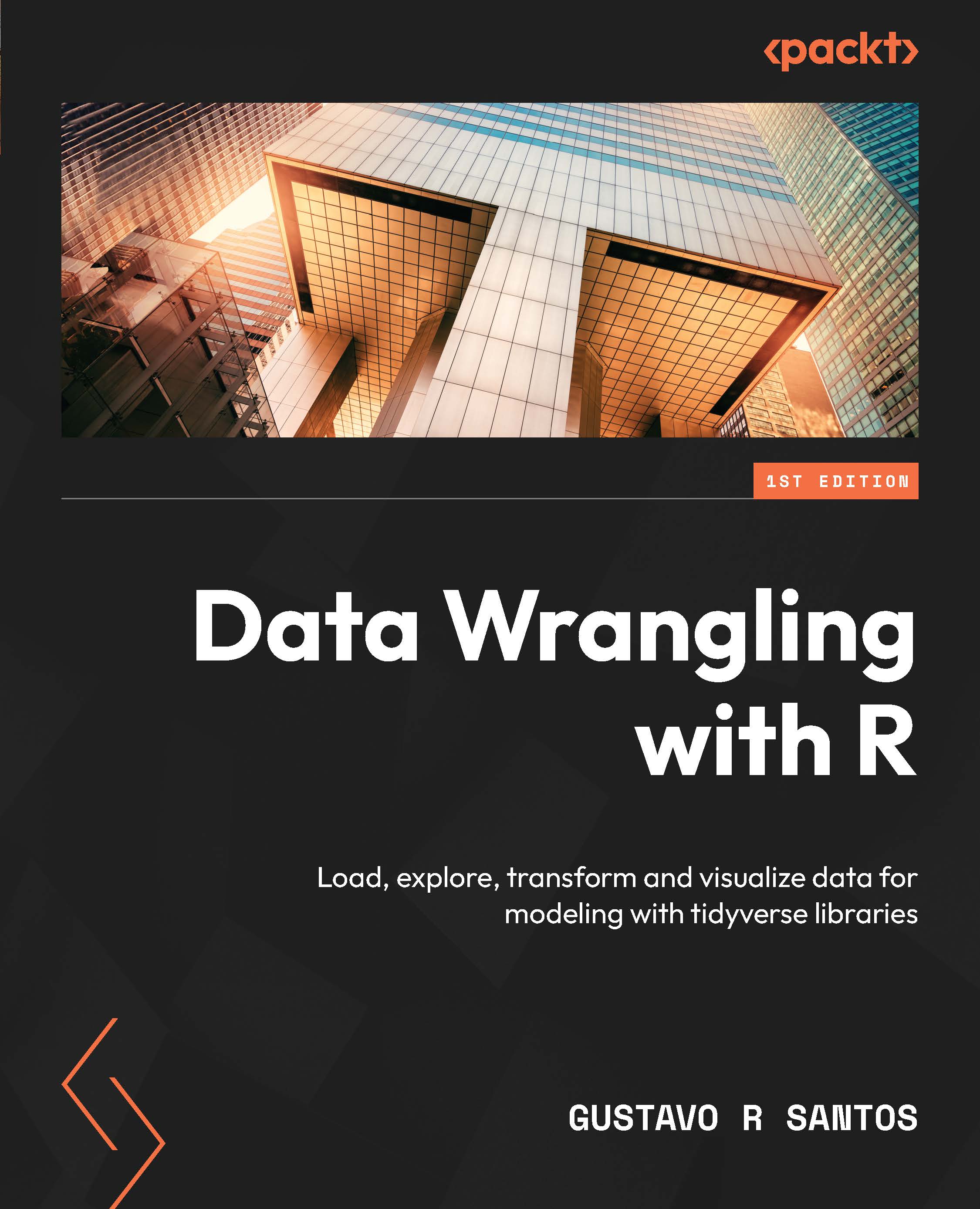
Data Wrangling with R
By :
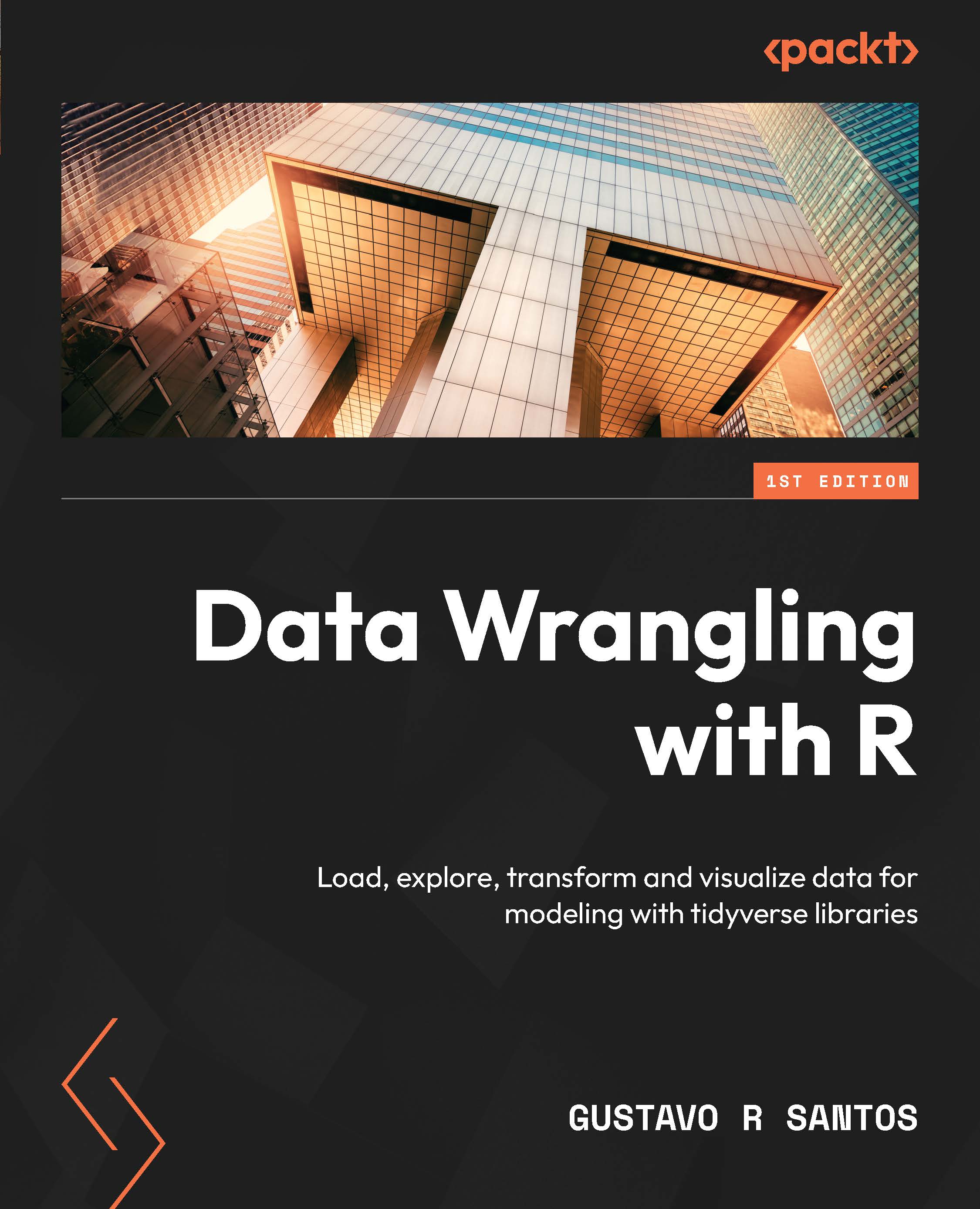
Now you know what data wrangling means, and I am sure that you share the same view as me that this is a tremendously important subject – otherwise, I don’t think you would be reading this book.
In statistics and data science areas, there is this frequently repeated phrase: garbage in, garbage out. This popular saying represents the central idea of the importance of wrangling data because it teaches us that our analysis or even our model will only be as good as the data that we present to it. You could also use the weakest link in the chain analogy to describe that importance, meaning that if your data is weak, the rest of the analysis could be easily broken by questions and arguments.
Let me give you a naïve example, but one that is still very precise, to illustrate my point. If we receive a dataset like in Figure 1.2, everything looks right at first glance. There are city names and temperatures, and it is a common format used to present data. However, for data science, this data may not be ideal for use just yet.
Figure 1.2 – Temperatures for cities
Notice that all the columns are referring to the same variable, which is Temperature
. We would have trouble plotting simple graphics in R with a dataset presented as in Figure 1.2, as well as using the dataset for modeling.
In this case, a simple transformation of the table from wide to long format would be enough to complete the data-wrangling task.
Figure 1.3 – Dataset ready for use
At first glance, Figure 1.2 might appear to be the better-looking option. And, in fact, it is for human eyes. The presentation of the dataset in Figure 1.2 makes it much easier for us to compare values and draw conclusions. However, we must not forget that we are dealing with computers, and machines don’t process data the same way humans do. To a computer, Figure 1.2 has seven variables: City
, Jan
, Feb
, Mar
, Apr
, May
, and Jun
, while Figure 1.3 has only three: City
, Month
, and Temperature
.
Now comes the fun part; let’s compare how a computer would receive both sets of data. A command to plot the temperature timeline by city for Figure 1.2 would be as follows: Computer, take a city and the temperatures during the months of Jan, Feb, Mar, Apr, May, and Jun in that city. Then consider each of the names of the months as a point on the x axis and the temperature associated as a point on the y axis. Plot a line for the temperature throughout the months for each of the cities.
Figure 1.3 is much clearer to the computer. It does not need to separate anything. The dataset is ready, so look how the command would be given: Computer, for each city, plot the month on the x axis and the temperature on the y axis.
Much simpler, agree? That is the importance of data wrangling for Data Science.
Performing good data wrangling will improve the overall quality of the entire analysis process. Here are the benefits:
Every project, being related with Data Science or not, can benefit from data wrangling. As we just listed, it brings many benefits to the analysis, impacting the quality of the deliverables in the end. But to get the best from it, there are steps to follow.
Change the font size
Change margin width
Change background colour