-
Book Overview & Buying
-
Table Of Contents
-
Feedback & Rating
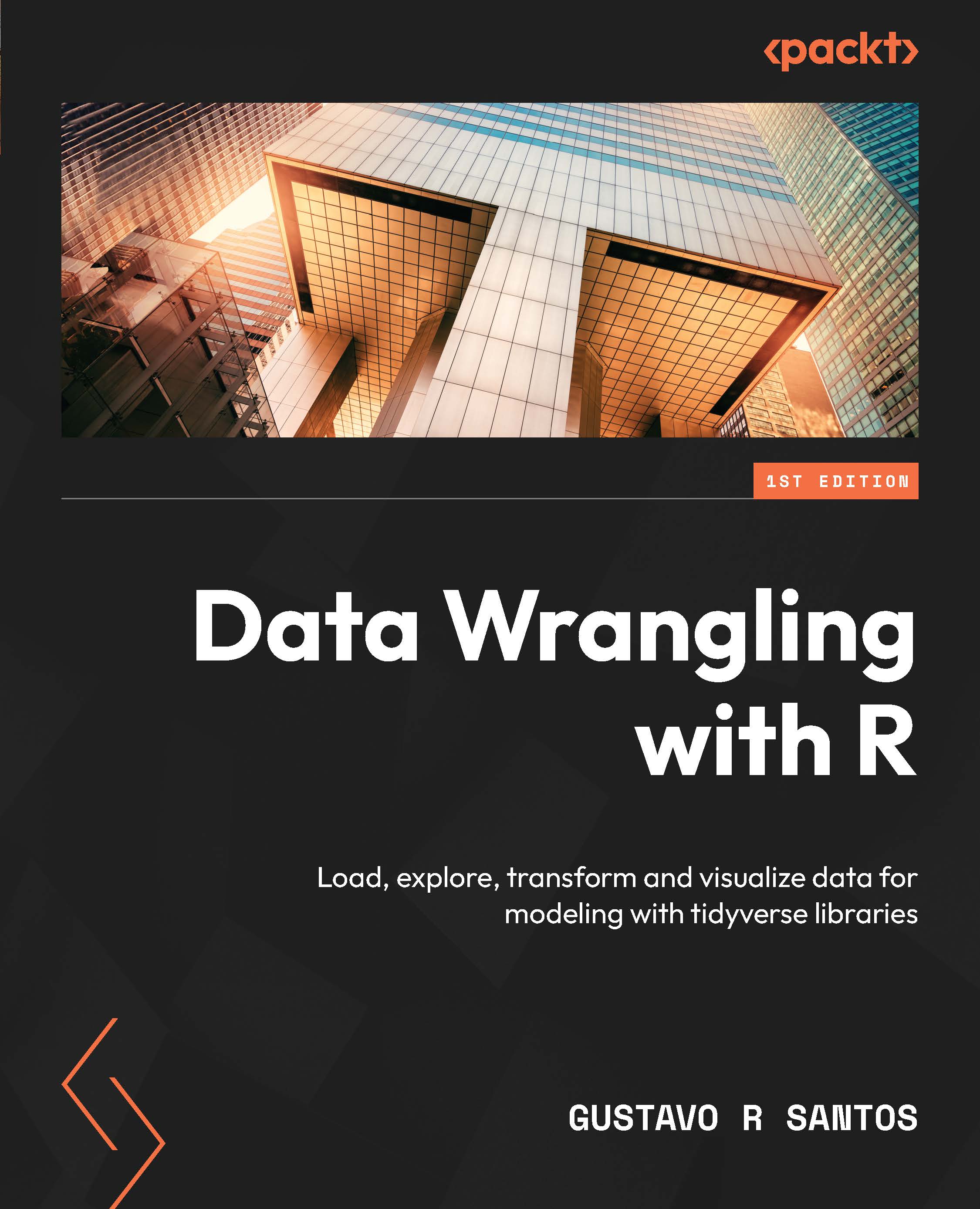
Data Wrangling with R
By :
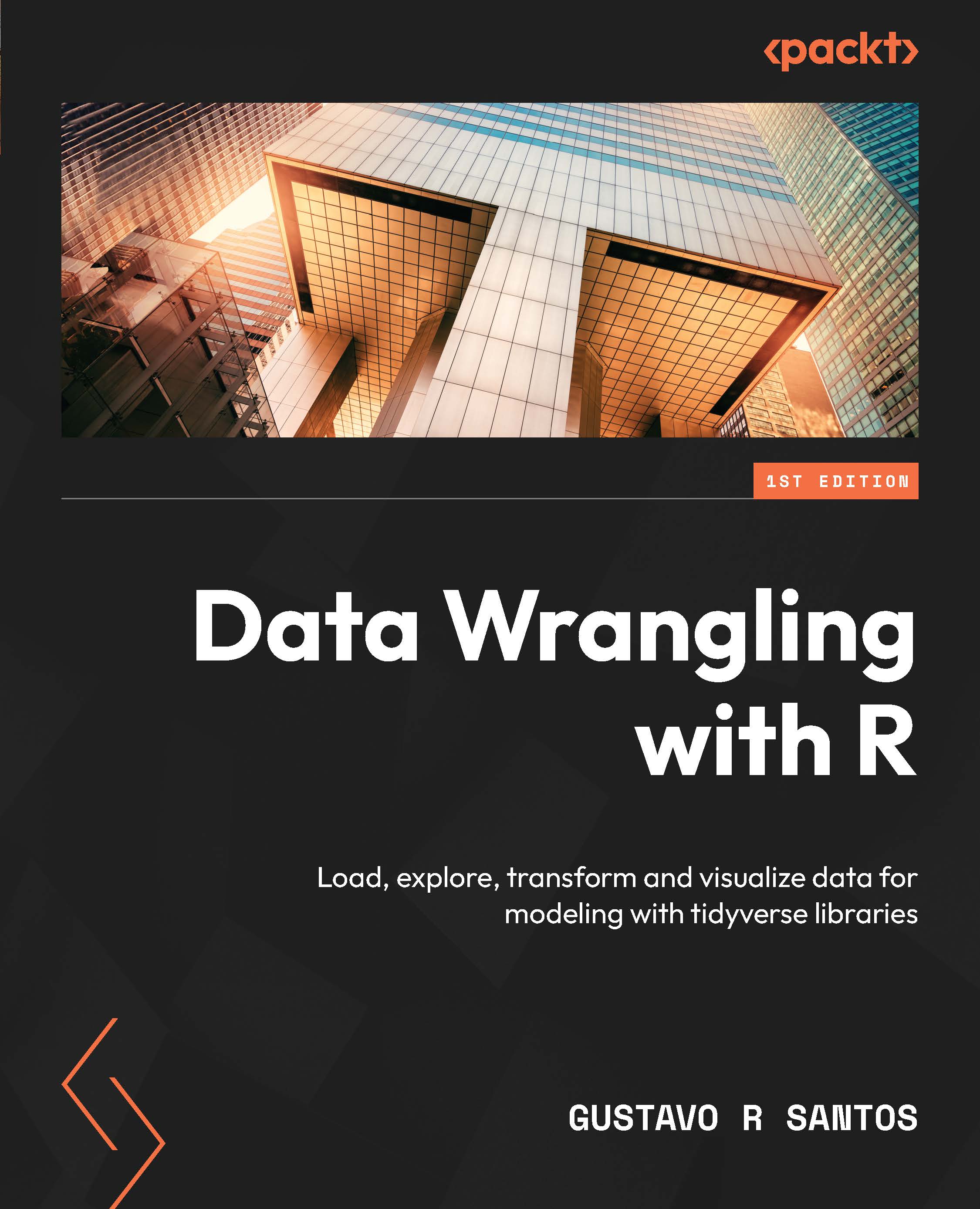
A dataset can and certainly will be acquired with imperfections. An example of imperfection is the use of the ?
sign instead of the default NA
for missing values for the Census Income dataset. This problem will require the question mark to be replaced with NA
first, and then filled with another value, such as the mean, the most frequent observation, or using more complex methods, even machine learning.
This case clearly illustrates the necessity of replacing and filling data points from a dataset. Using tidyr, there are specific functions to replace and fill in missing data.
First, the ?
sign needs to be replaced with NA
, before we can think of filling the missing values. As seen in Chapter 7, there are only missing values for the workclass
(1836
), occupation
(1843
), and native_country
(583
) columns. To confirm that, a loop through the variables searching for ?
would be the fastest resource:
# Loop through variables looking for cells == "...
Change the font size
Change margin width
Change background colour