-
Book Overview & Buying
-
Table Of Contents
-
Feedback & Rating
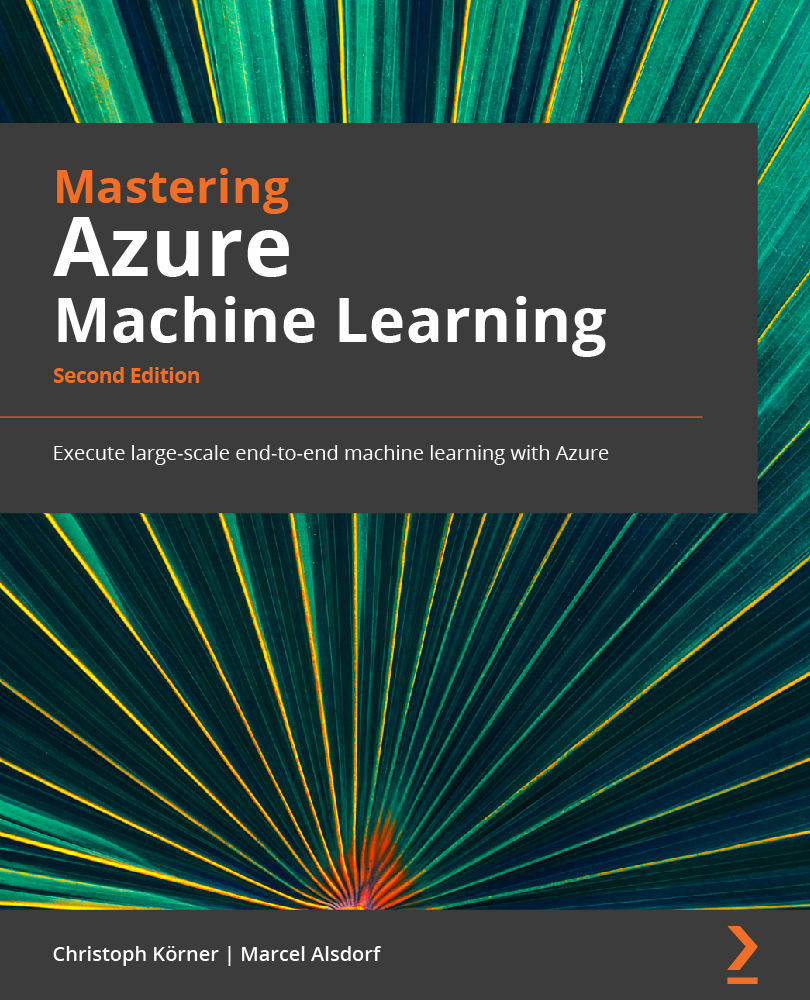
Mastering Azure Machine Learning
By :
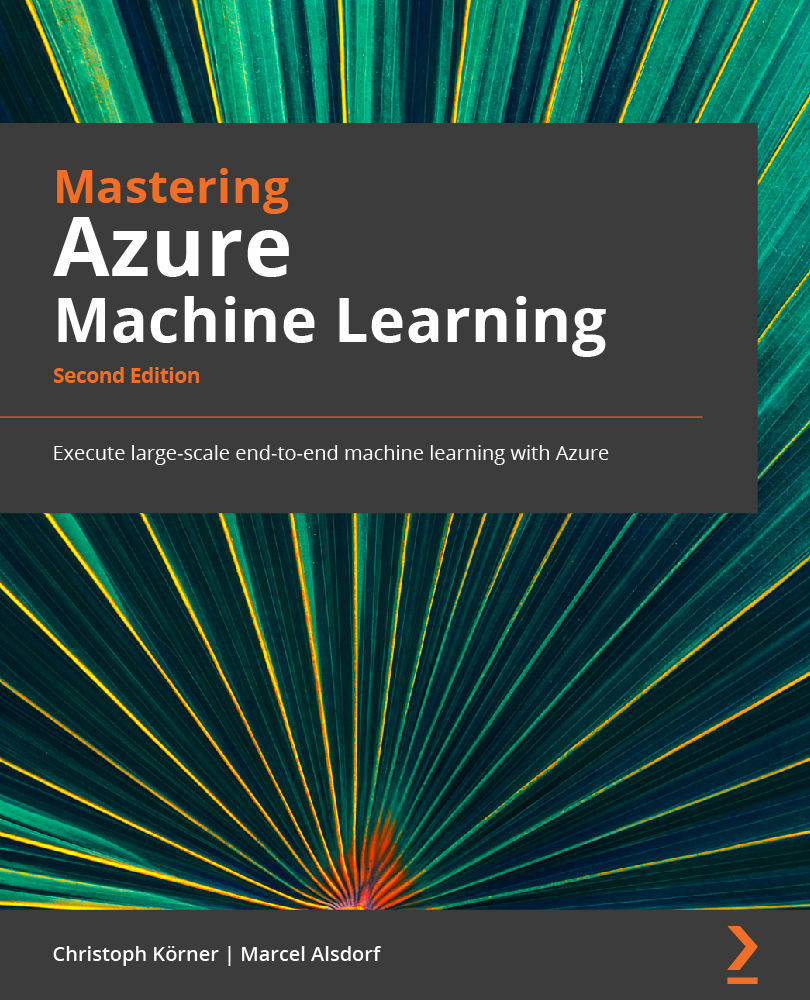
Supervised tree-based ensemble classification and regression techniques have proven very successful in many practical real-world applications in recent years. Hence, they are widely used today in various applications, including fraud detection, recommendation engines, tagging engines, and many more. All your favorite mobile and desktop operating systems, Office programs, and audio or video streaming services make heavy use of them every day.
Therefore, in this section, we will dive into the main reasons for their popularity and performance, both for training and scoring. If you are an expert on traditional ML algorithms and know the difference between boosting and bagging, you might as well jump right to the next section, Training an ensemble classifier model using LightGBM, where we put the theory into practice.
We will first look at decision trees, a very simple technique that is decades old. We encourage you to follow along even...