-
Book Overview & Buying
-
Table Of Contents
-
Feedback & Rating
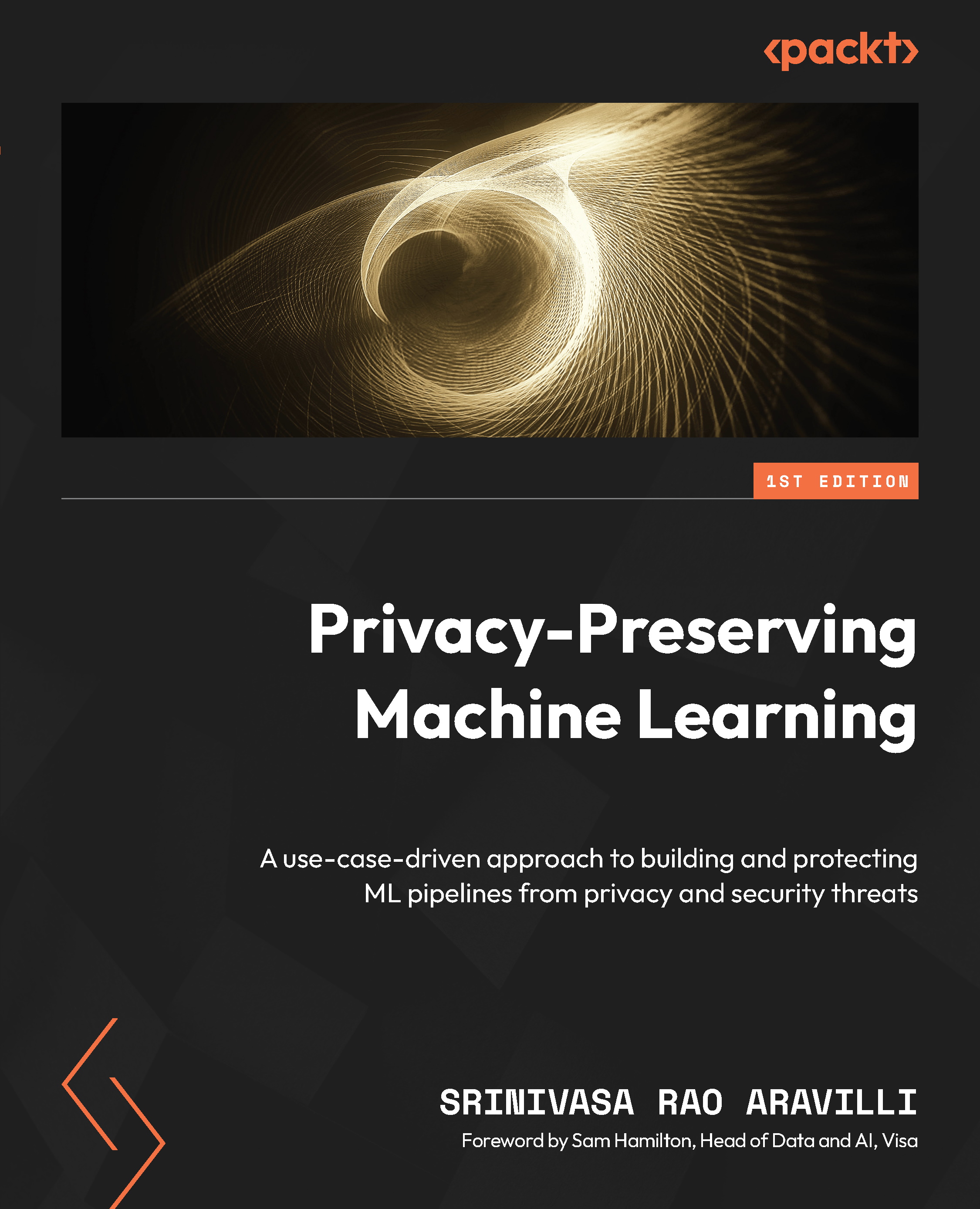
Privacy-Preserving Machine Learning
By :
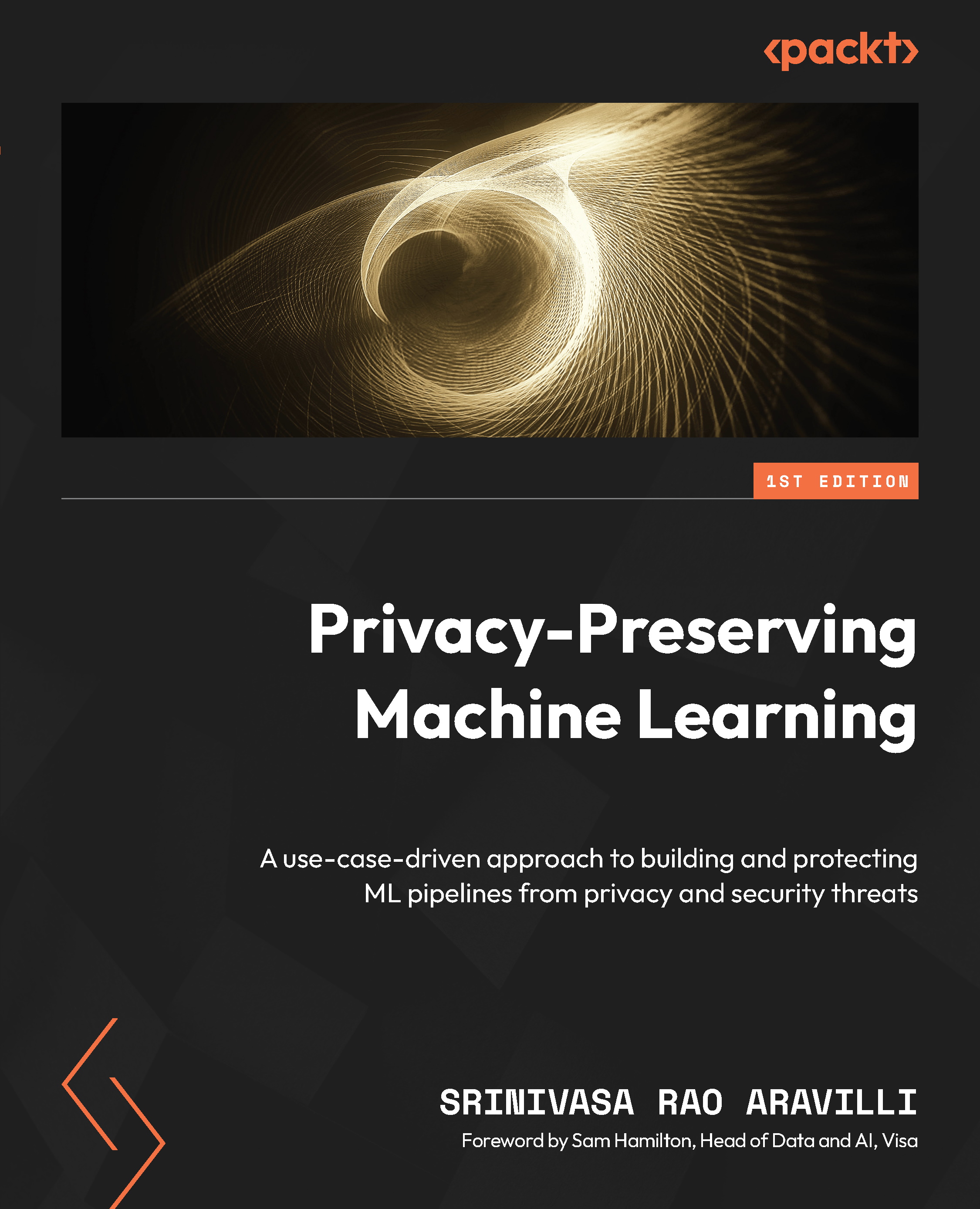
As this ebook edition doesn't have fixed pagination, the page numbers below are hyperlinked for reference only, based on the printed edition of this book.
Symbols
ℓ-diversity 89
original dataset 90
versus k-anonymity 96
versus t-closeness 96
A
adaptive federated learning (AFL) 276
Advanced Encryption Standard (AES) 285
using, for encryption example 285, 286
adversarial attacks 51
AI standards 341
NIST 341
OWASP Top 10 for LLM applications 342
Anjuna
URL 331
attestation 328
binary attestation 328
runtime attestation 328
source code attestation 328
AuthorsNN class 69
average queries 134
differential privacy algorithms, using with 136, 137
AWS Nitro Enclaves 331
Azure enclaves 331
B
backpropagation 66
BERT 352
black-box attack 52
BLAKE2 290
Blowfish 285
bootstrapping technique 293
Brain Tumor Segmentation (BraTS)...