-
Book Overview & Buying
-
Table Of Contents
-
Feedback & Rating
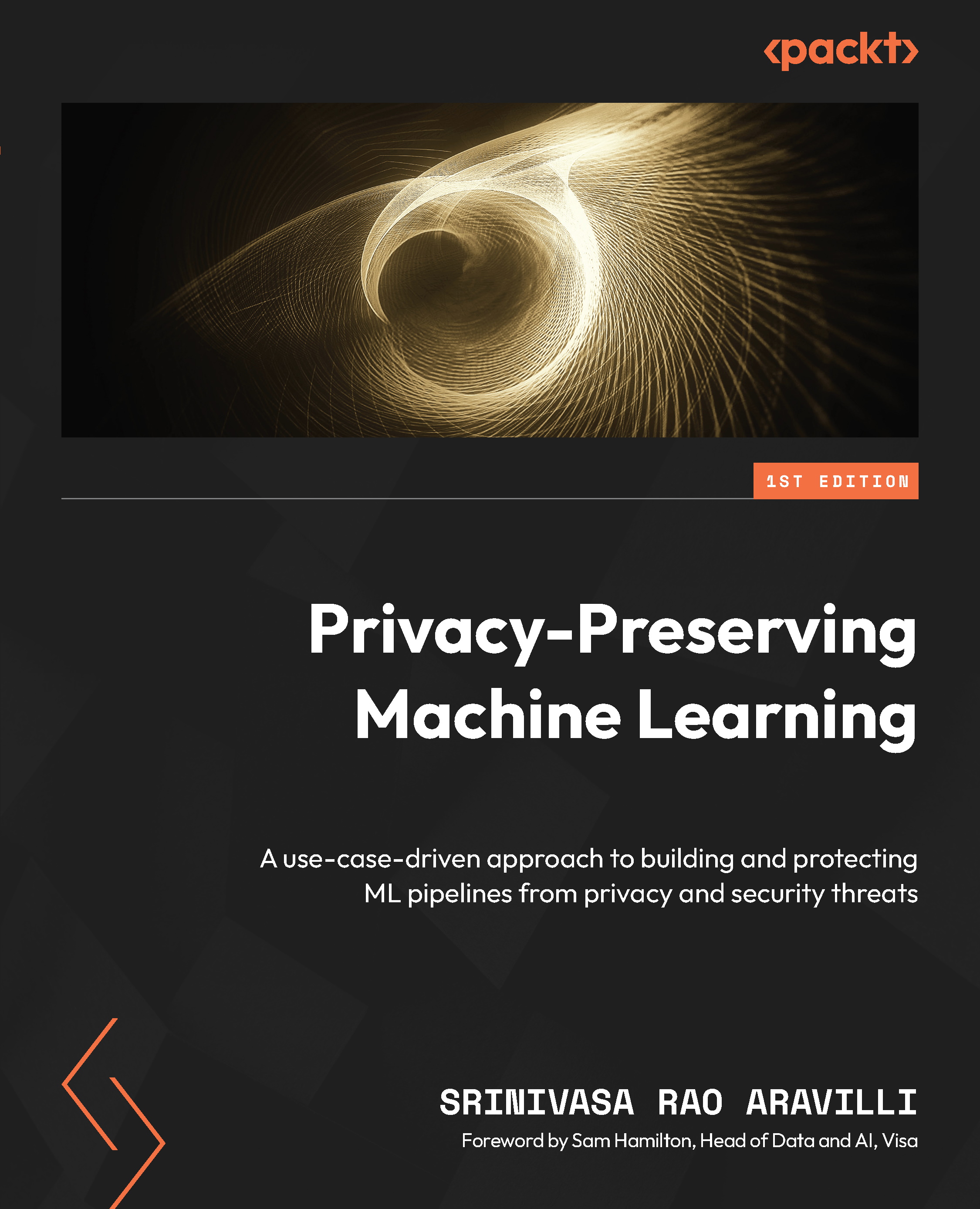
Privacy-Preserving Machine Learning
By :
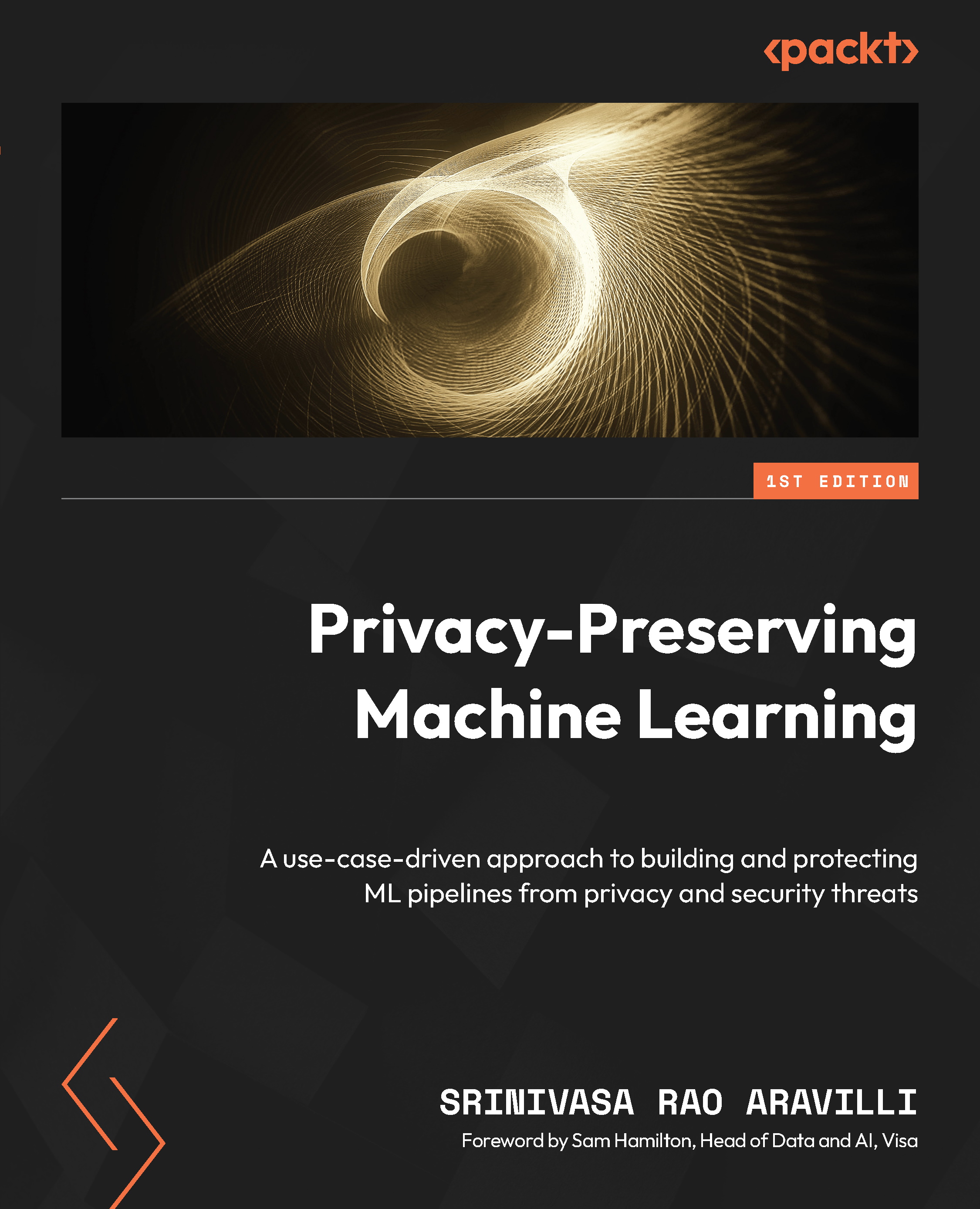
Privacy-Preserving Machine Learning
By:
Overview of this book
– In an era of evolving privacy regulations, compliance is mandatory for every enterprise
– Machine learning engineers face the dual challenge of analyzing vast amounts of data for insights while protecting sensitive information
– This book addresses the complexities arising from large data volumes and the scarcity of in-depth privacy-preserving machine learning expertise, and covers a comprehensive range of topics from data privacy and machine learning privacy threats to real-world privacy-preserving cases
– As you progress, you’ll be guided through developing anti-money laundering solutions using federated learning and differential privacy
– Dedicated sections will explore data in-memory attacks and strategies for safeguarding data and ML models
– You’ll also explore the imperative nature of confidential computation and privacy-preserving machine learning benchmarks, as well as frontier research in the field
– Upon completion, you’ll possess a thorough understanding of privacy-preserving machine learning, equipping them to effectively shield data from real-world threats and attacks
Table of Contents (17 chapters)
Preface
Part 1: Introduction to Data Privacy and Machine Learning
Chapter 1: Introduction to Data Privacy, Privacy Breaches, and Threat Modeling
Chapter 2: Machine Learning Phases and Privacy Threats/Attacks in Each Phase
Part 2: Use Cases of Privacy-Preserving Machine Learning and a Deep Dive into Differential Privacy
Chapter 3: Overview of Privacy-Preserving Data Analysis and an Introduction to Differential Privacy
Chapter 4: Overview of Differential Privacy Algorithms and Applications of Differential Privacy
Chapter 5: Developing Applications with Differential Privacy Using Open Source Frameworks
Part 3: Hands-On Federated Learning
Chapter 6: Federated Learning and Implementing FL Using Open Source Frameworks
Chapter 7: Federated Learning Benchmarks, Start-Ups, and the Next Opportunity
Part 4: Homomorphic Encryption, SMC, Confidential Computing, and LLMs
Chapter 8: Homomorphic Encryption and Secure Multiparty Computation
Chapter 9: Confidential Computing – What, Why, and the Current State
Chapter 10: Preserving Privacy in Large Language Models
Index
Customer Reviews