-
Book Overview & Buying
-
Table Of Contents
-
Feedback & Rating
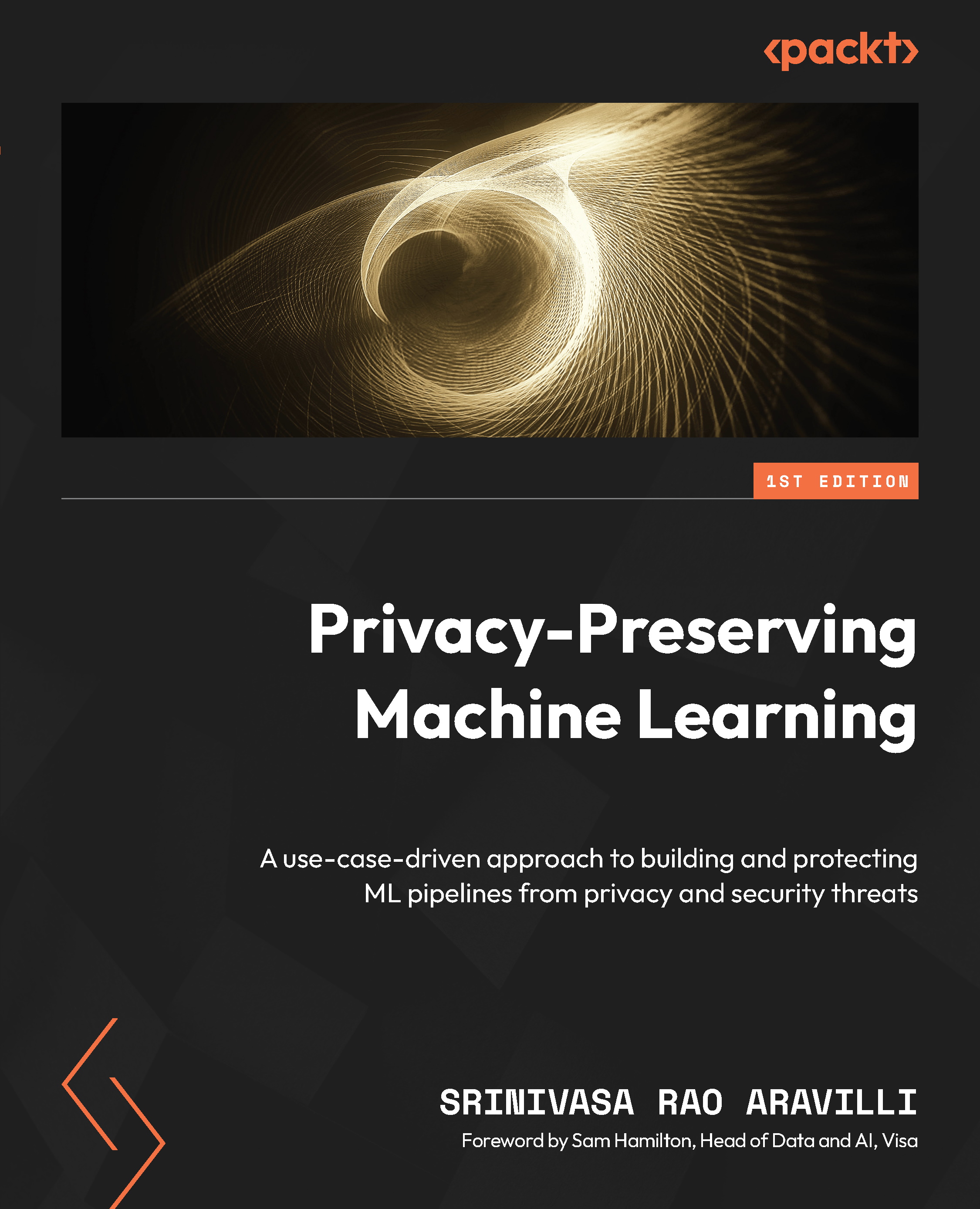
Privacy-Preserving Machine Learning
By :
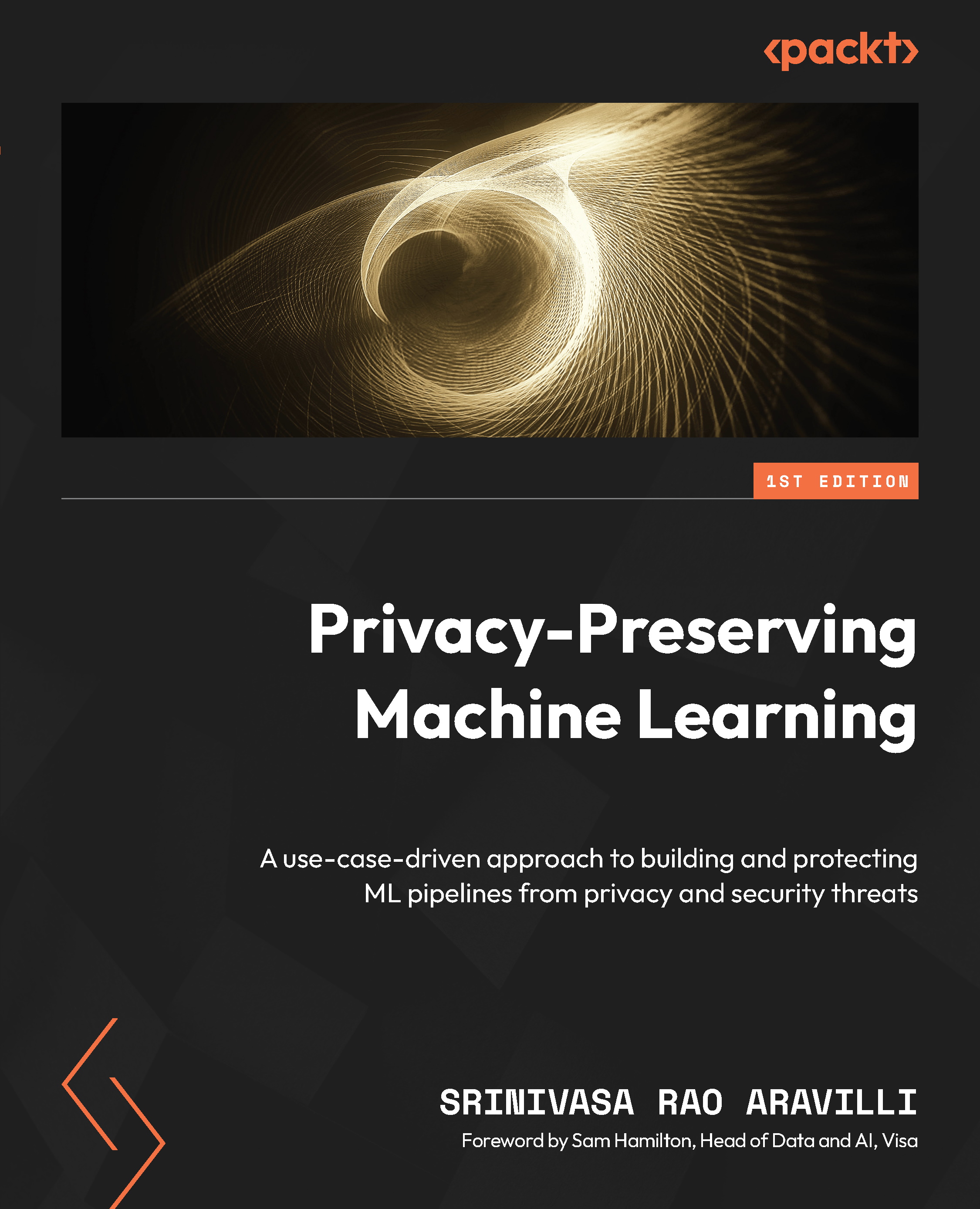
In this section, we will focus on developing a fraud detection model using the PyTorch framework. Additionally, we will train deep learning models with differential privacy using open source frameworks such as PyTorch and Opacus. Using the PyTorch framework, we will develop a deep learning model specifically designed for fraud detection. PyTorch is a popular open source deep learning library that provides a flexible and efficient platform for building and training neural networks. Its rich set of tools and APIs make it well-suited for developing sophisticated machine learning models.
To incorporate differential privacy into the training process, we will utilize the Opacus library. Opacus is an open source PyTorch extension that provides tools for training deep learning models with differential privacy. It offers mechanisms such as gradient clipping, noise addition, and privacy analysis, which help ensure that the trained model preserves the...