-
Book Overview & Buying
-
Table Of Contents
-
Feedback & Rating
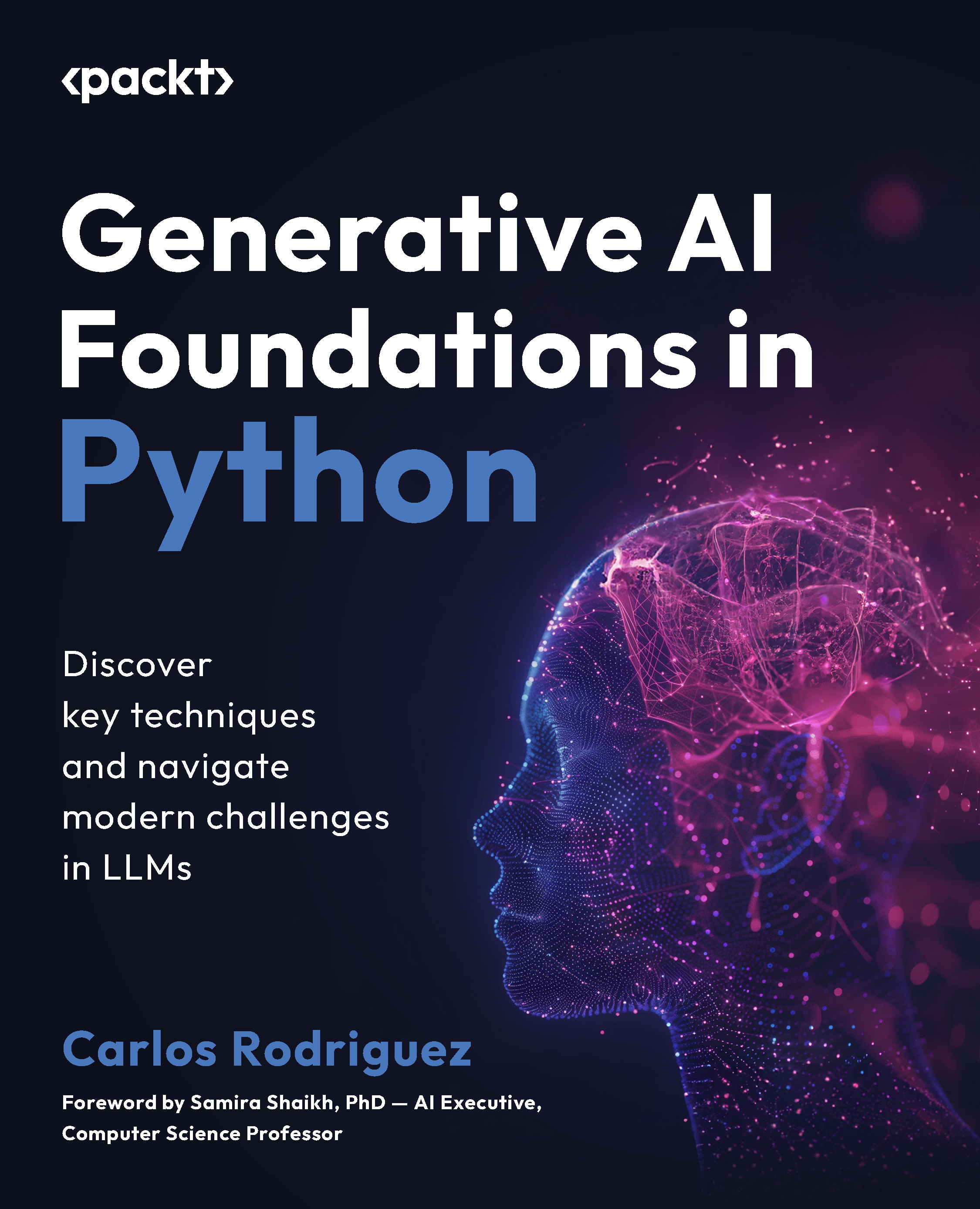
Generative AI Foundations in Python
By :
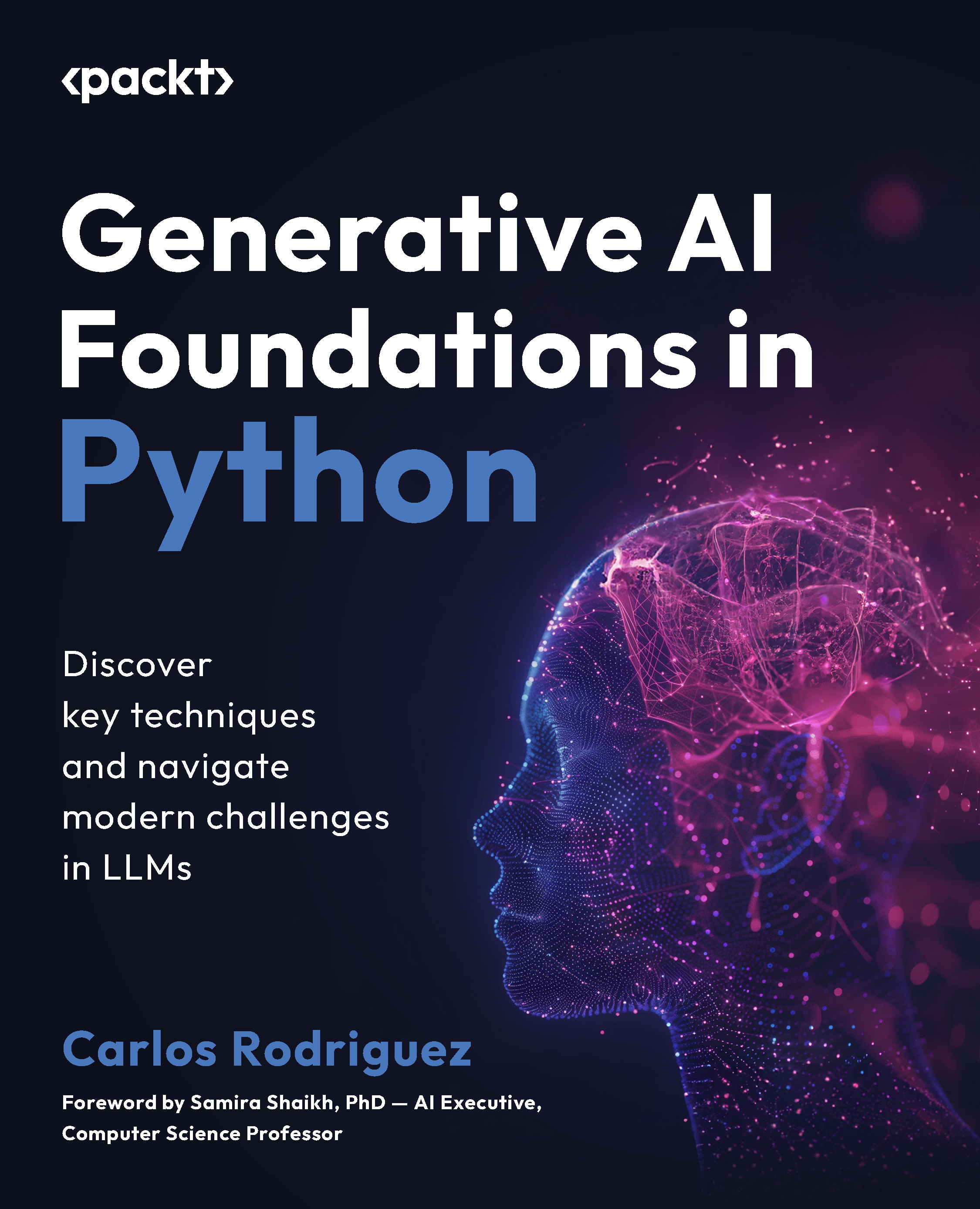
In this chapter, we focused on the strategic decision-making process between fine-tuning and in-context learning for StyleSprint’s AI-driven customer service system. While in-context learning, particularly few-shot learning, offers adaptability and resource efficiency, it may not consistently align with StyleSprint’s brand tone and customer service guidelines. This method relies heavily on the quality and relevance of the examples provided in the prompts, requiring careful crafting to ensure optimal outcomes.
On the other hand, PEFT methods such as AdaLoRA, offer a more focused approach to adapt a pre-trained model to the specific demands of customer service queries. PEFT methods modify only a small subset of a model’s parameters, reducing the computational burden while still achieving high performance. This efficiency is crucial for real-world applications where computational resources and response accuracy are both key considerations.
Ultimately...