-
Book Overview & Buying
-
Table Of Contents
-
Feedback & Rating
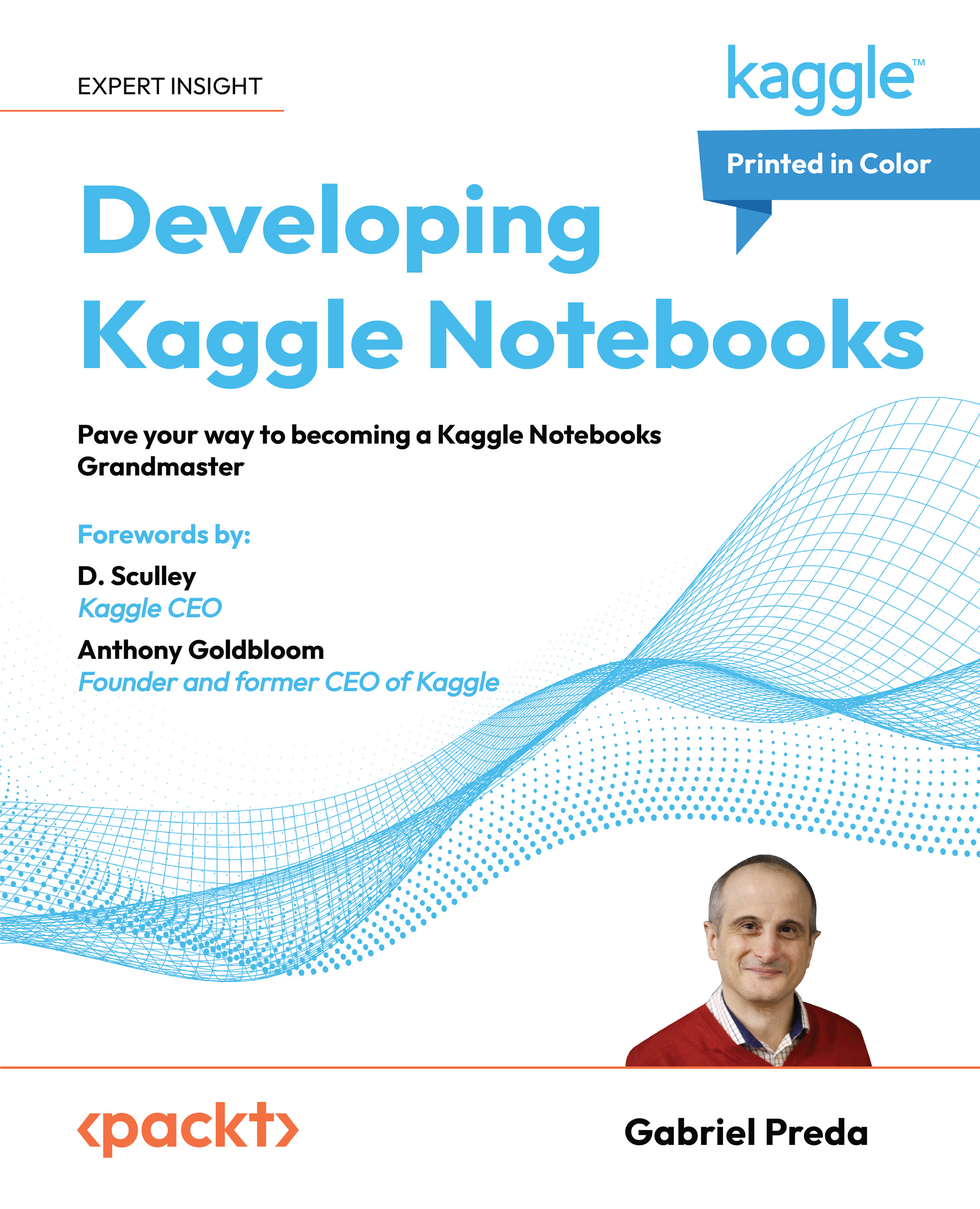
Developing Kaggle Notebooks
By :
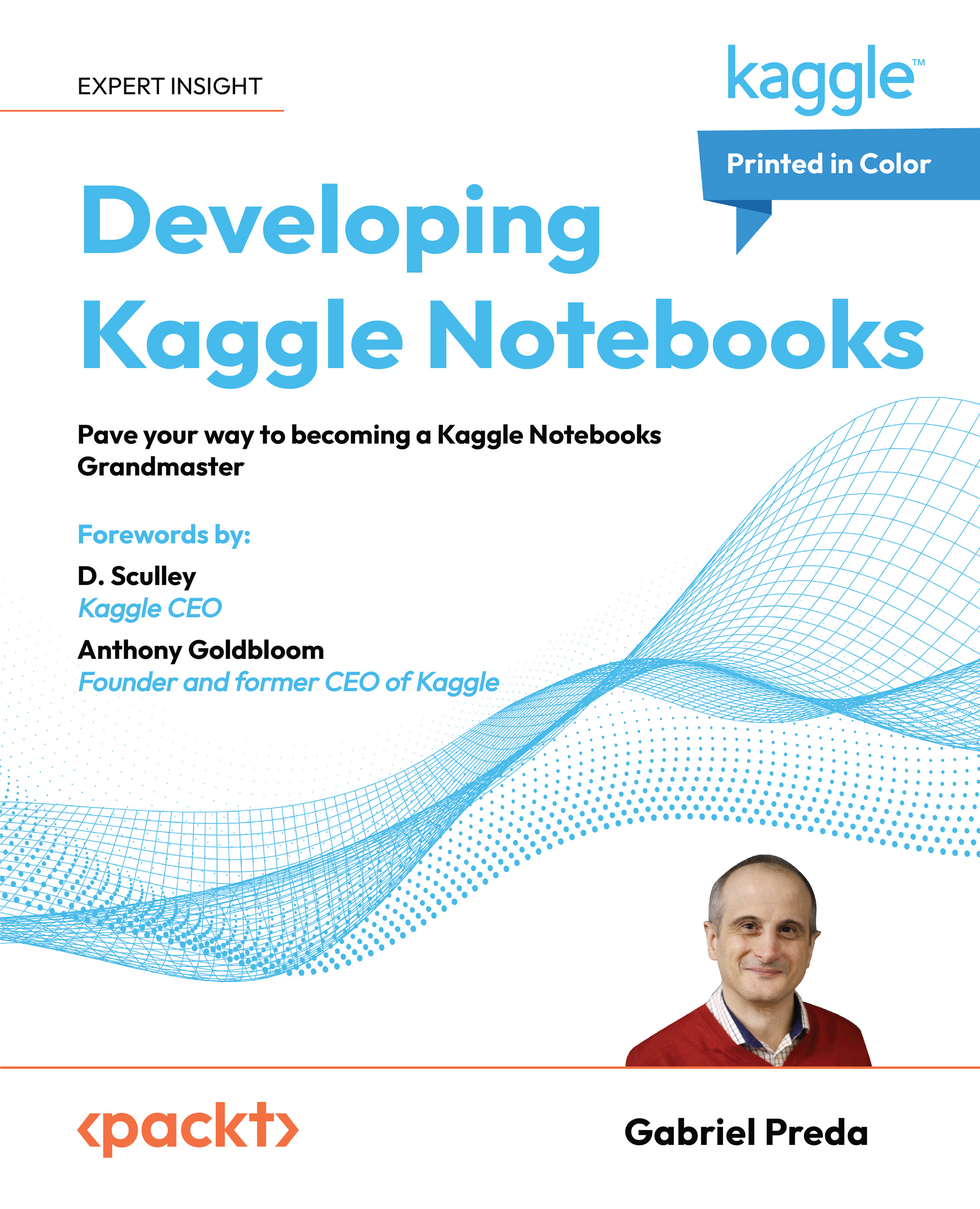
You should have a basic understanding of Python and familiarity with Jupyter Notebooks. Ideally, you will also need some basic knowledge of libraries like pandas and NumPy.
The chapters contain both theory and code. If you want to run the code in the book, the easiest way is to follow the links on the README.md
introduction page in the GitHub project for each notebook, fork the notebook, and run it on Kaggle. The Kaggle environment is pre-installed with all the needed Python libraries. Alternatively, you can download the notebooks from the GitHub project, upload them on Kaggle, attach the dataset resources mentioned in the book for each specific example, and run them. Another alternative is to download the datasets on Kaggle, install your own local environment, and run the notebooks there. In this case, however, you will need more advanced knowledge about how to set up a conda
environment locally and install Python libraries using pip install
or conda install
.
Requirements for the chapter exercises |
Version no. |
Python |
3.9 or higher |
All exercises developed on the Kaggle platform use the current Python version, which is 3.10 at the time of writing this book.
The code bundle for the book is hosted on GitHub at https://github.com/PacktPublishing/Developing-Kaggle-Notebooks. We also have other code bundles from our rich catalog of books and videos available at https://github.com/PacktPublishing/. Check them out!
We also provide a PDF file that has color images of the screenshots/diagrams used in this book. You can download it here: https://packt.link/gbp/9781805128519.
There are a number of text conventions used throughout this book.
CodeInText
: Indicates code words in text, database table names, folder names, filenames, file extensions, pathnames, dummy URLs, user input, and Twitter handles. For example: “Run the info()
function for each dataset.”
A block of code is set as follows:
for sentence in selected_text["comment_text"].head(5):
print("\n")
doc = nlp(sentence)
for ent in doc.ents:
print(ent.text, ent.start_char, ent.end_char, ent.label_)
displacy.render(doc, style="ent",jupyter=True)
Any command-line input or output is written as follows:
!pip install kaggle
Bold: Indicates a new term, an important word, or words that you see on the screen. For instance, words in menus or dialog boxes appear in the text like this. For example: “You will have to start a notebook and then choose the Set as Utility Script menu item from the File menu.”
Warnings or important notes appear like this.
Tips and tricks appear like this.