-
Book Overview & Buying
-
Table Of Contents
-
Feedback & Rating
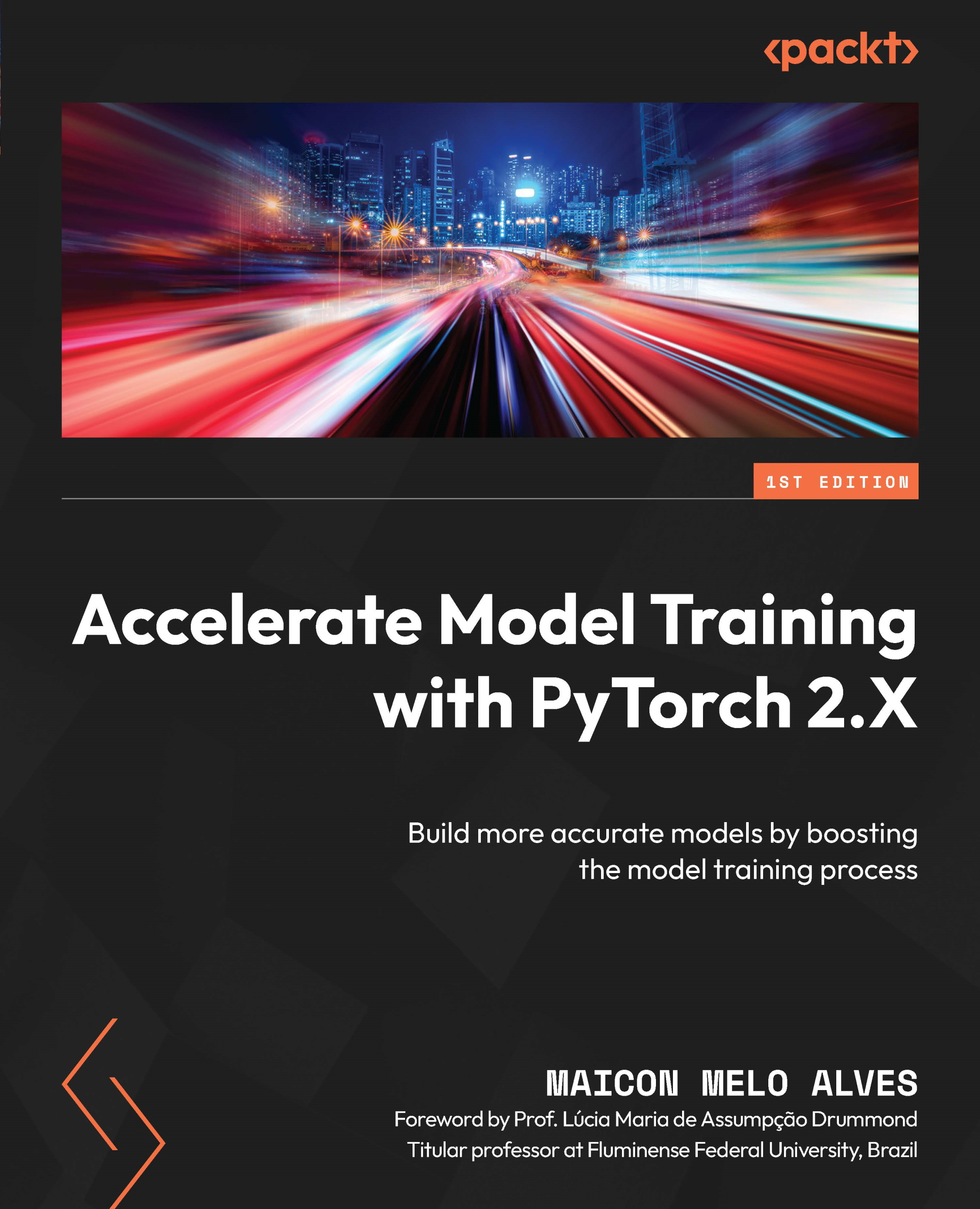
Accelerate Model Training with PyTorch 2.X
By :
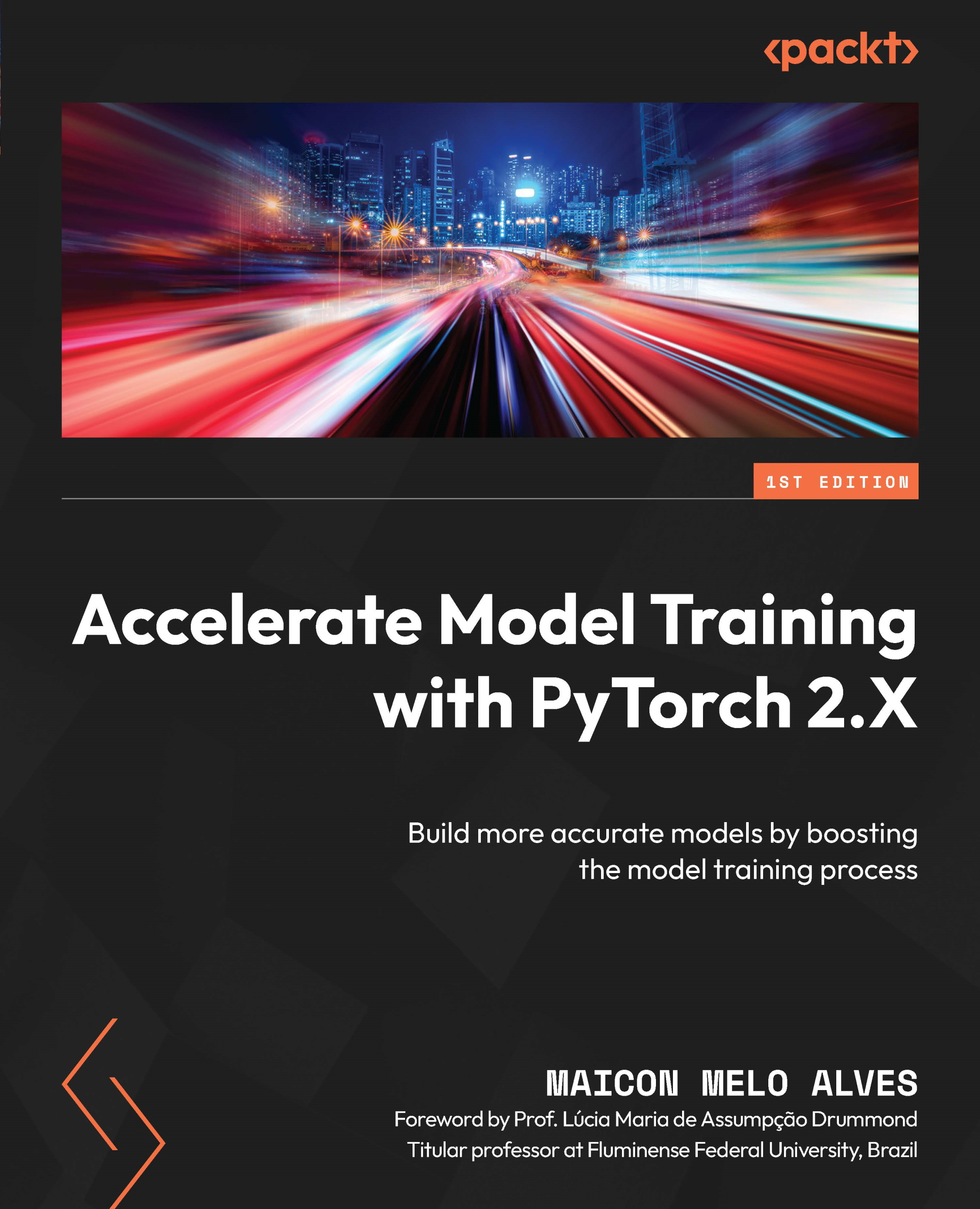
Now that we’ve brushed up on how the training process works, let’s understand the computational cost required to train a model. By using the terms computational cost or burden, we mean the computing power needed to execute the training process. The higher the computational cost, the higher the time taken to train the model. In the same way, the higher the computational burden, the higher the computing resources required to train the model.
Essentially, we can say the computational burden to train a model is defined by a three-fold factor, as illustrated in Figure 1.6:
Figure 1.6 – Factors that influence the training computational burden
Each one of these factors contributes (to some degree) to the computational complexity imposed by the training process. Let’s talk about each one of them.
Hyperparameters define two aspects of neural networks...