-
Book Overview & Buying
-
Table Of Contents
-
Feedback & Rating
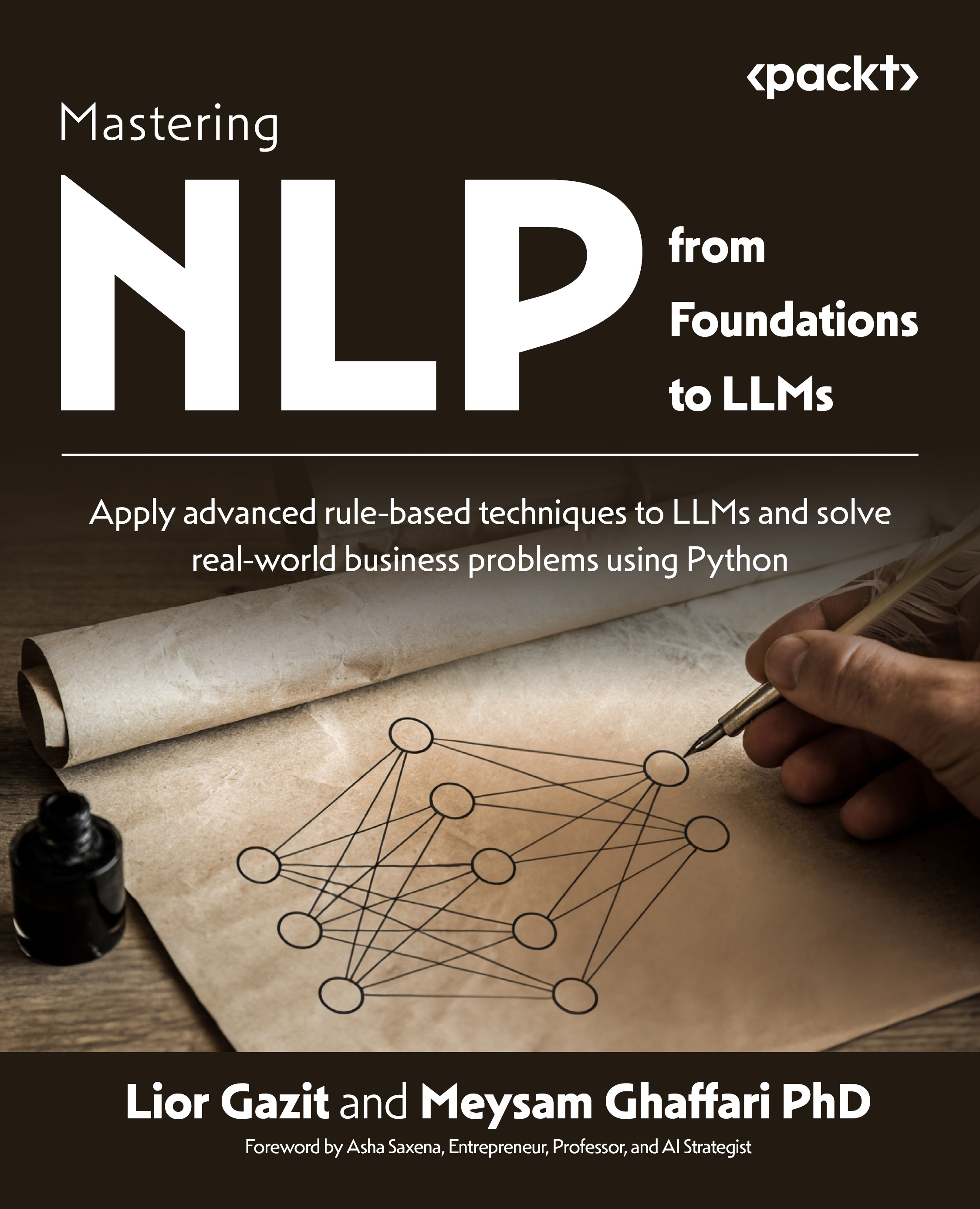
Mastering NLP from Foundations to LLMs
By :
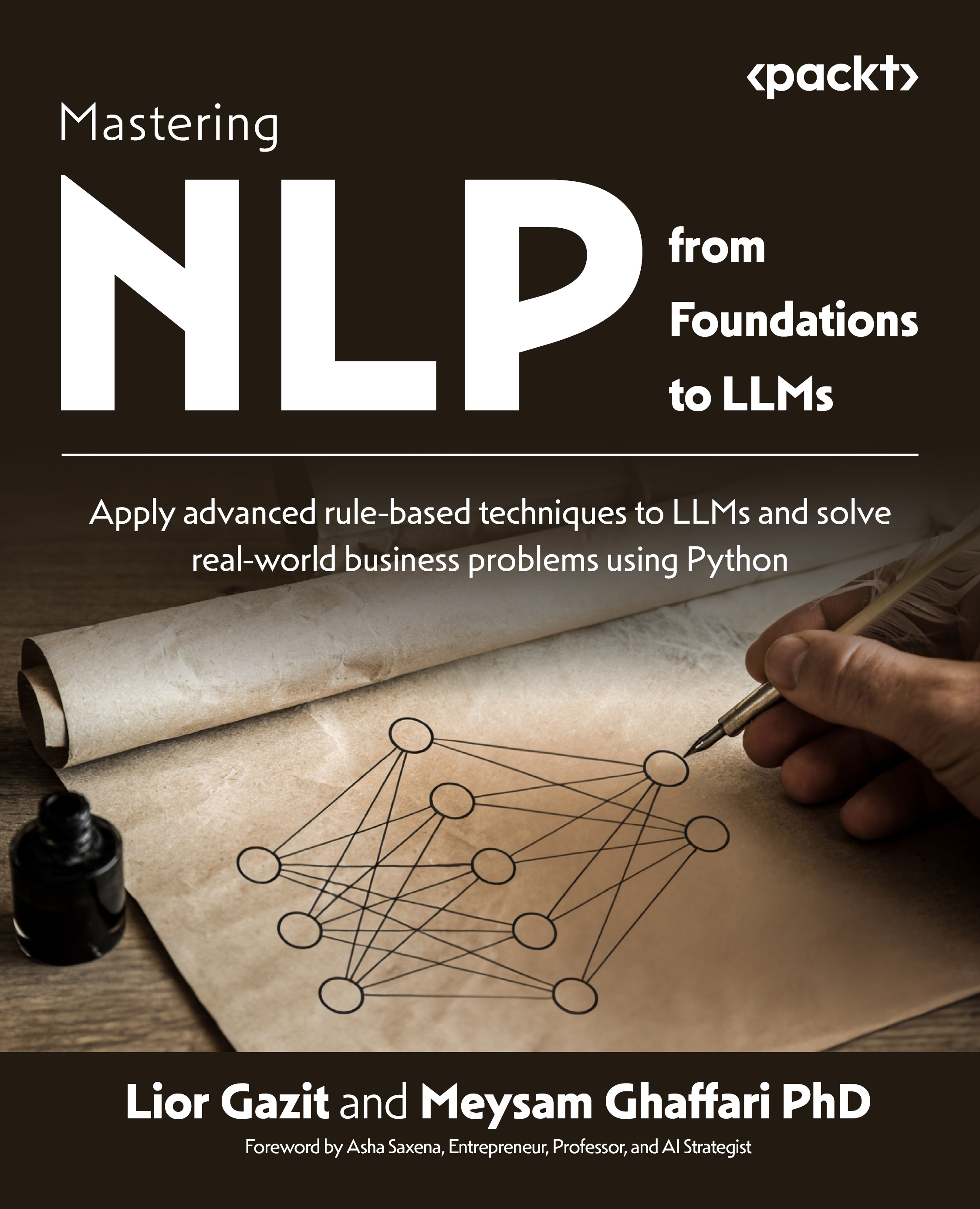
Mastering NLP from Foundations to LLMs
By:
Overview of this book
Do you want to master Natural Language Processing (NLP) but don’t know where to begin? This book will give you the right head start. Written by leaders in machine learning and NLP, Mastering NLP from Foundations to LLMs provides an in-depth introduction to techniques. Starting with the mathematical foundations of machine learning (ML), you’ll gradually progress to advanced NLP applications such as large language models (LLMs) and AI applications. You’ll get to grips with linear algebra, optimization, probability, and statistics, which are essential for understanding and implementing machine learning and NLP algorithms. You’ll also explore general machine learning techniques and find out how they relate to NLP. Next, you’ll learn how to preprocess text data, explore methods for cleaning and preparing text for analysis, and understand how to do text classification. You’ll get all of this and more along with complete Python code samples.
By the end of the book, the advanced topics of LLMs’ theory, design, and applications will be discussed along with the future trends in NLP, which will feature expert opinions. You’ll also get to strengthen your practical skills by working on sample real-world NLP business problems and solutions.
Table of Contents (14 chapters)
Preface
Chapter 1: Navigating the NLP Landscape: A Comprehensive Introduction
Chapter 2: Mastering Linear Algebra, Probability, and Statistics for Machine Learning and NLP
Chapter 3: Unleashing Machine Learning Potentials in Natural Language Processing
Chapter 4: Streamlining Text Preprocessing Techniques for Optimal NLP Performance
Chapter 5: Empowering Text Classification: Leveraging Traditional Machine Learning Techniques
Chapter 6: Text Classification Reimagined: Delving Deep into Deep Learning Language Models
Chapter 7: Demystifying Large Language Models: Theory, Design, and Langchain Implementation
Chapter 8: Accessing the Power of Large Language Models: Advanced Setup and Integration with RAG
Chapter 9: Exploring the Frontiers: Advanced Applications and Innovations Driven by LLMs
Chapter 10: Riding the Wave: Analyzing Past, Present, and Future Trends Shaped by LLMs and AI
Chapter 11: Exclusive Industry Insights: Perspectives and Predictions from World Class Experts
Index
Customer Reviews