-
Book Overview & Buying
-
Table Of Contents
-
Feedback & Rating
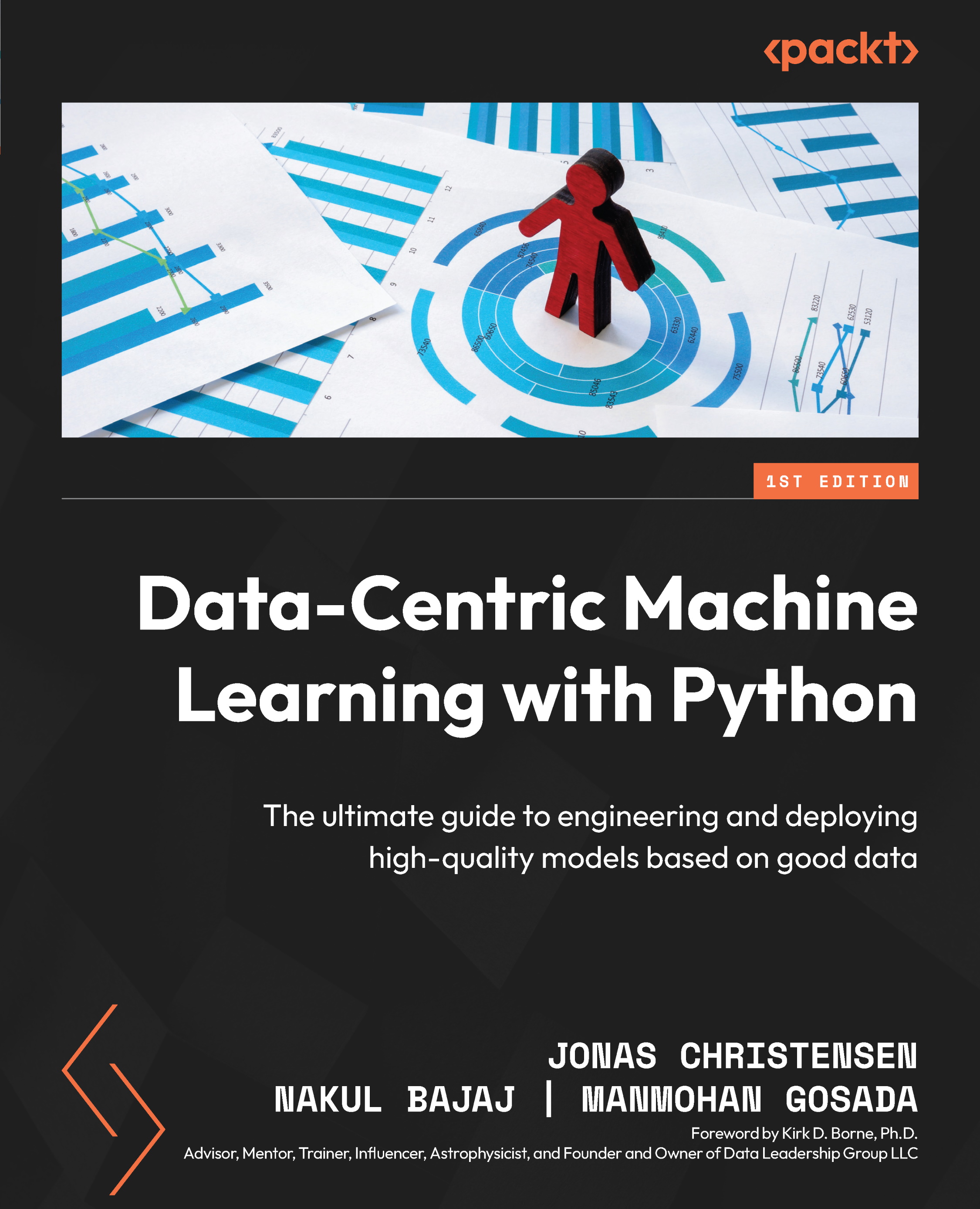
Data-Centric Machine Learning with Python
By :
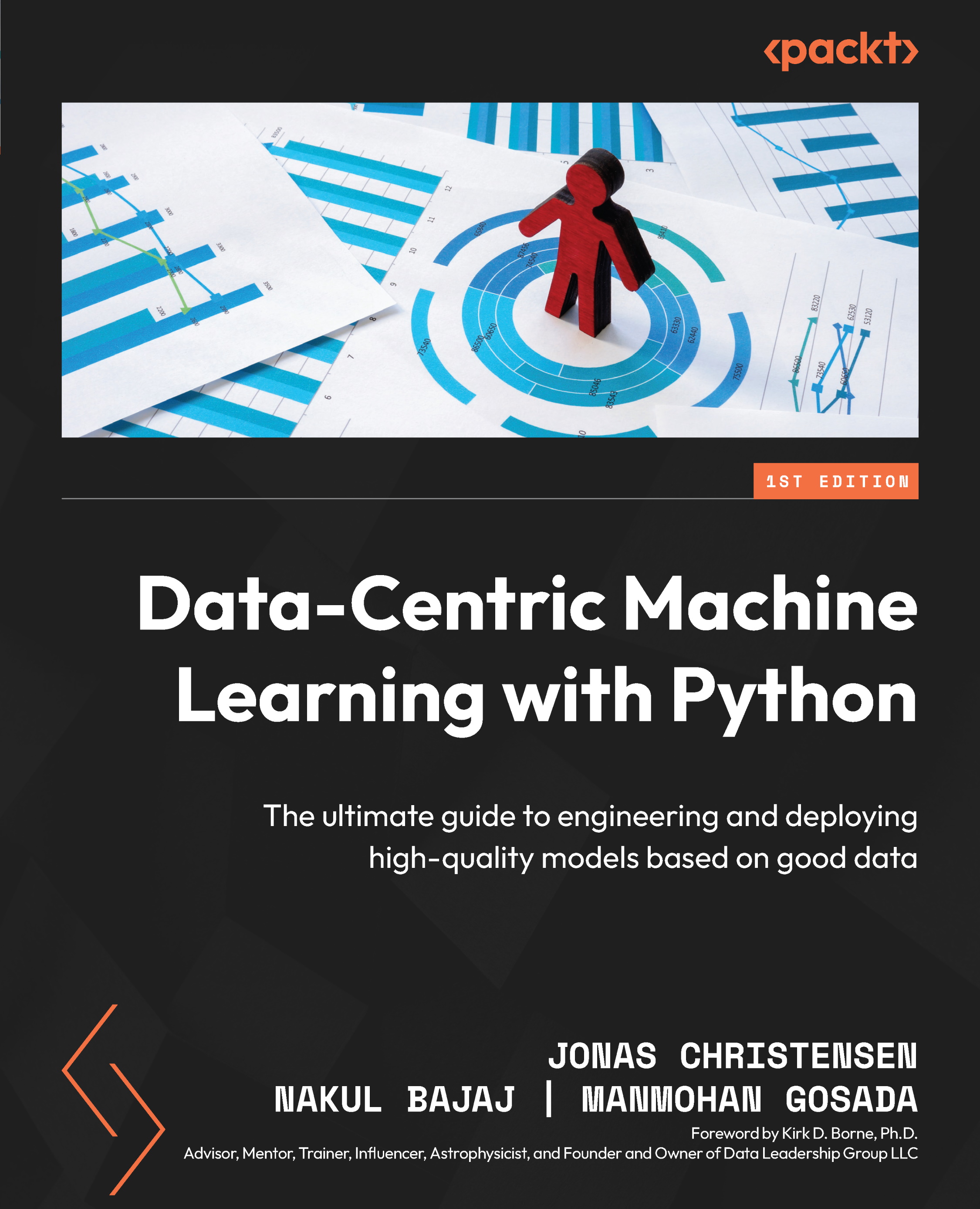
If you’re reading this, you’ve taken the first steps on a pioneering journey to building and implementing machine learning models that are more robust, accurate, fairer, less biased, and easier to explain.
This is a big claim, we know. We are comfortable making it, however, on the basis of the huge and relatively untapped potential we see in the data-centric approach to machine learning development.
Why do we consider data-centric machine learning pioneering?
It may seem obvious that improving data quality will lead to more predictive models. However, machine learning research to date has mainly focused on evolving the various algorithms and tools to build and tune models.
As a result, we have available at our fingertips a vast array of machine learning algorithms, tools, and techniques that can give us great models at a low cost, given the right quality and volume of input data.
Model architectures are largely a solved problem in most situations. What data scientists, and the organizations they work in, typically lack are best-practice frameworks, tools, and techniques for improving data quality.
Data-centric machine learning builds on the predominant model-centric approach to model development by exploiting the big opportunities that lie in better input data.
Putting a bigger emphasis on data collection and engineering requires us to streamline our processes for collecting quality data and invent new techniques for engineering datasets that provide more signals with much less data.
Many of the techniques and examples you will learn about in this book are based on cutting-edge research and the application of modern practices to collecting, engineering, and synthetically generating great datasets.
Data-centric machine learning also necessitates a much stronger collaboration between data scientists, subject-matter experts, and data labelers. As you will learn throughout this book, data-centricity typically starts with humans collecting and labeling data in a way that serves operational and data science needs.
In many organizations, it is uncommon to collect data for machine learning purposes specifically. A more systematic approach to collecting and labeling data for data science will not only lead to better data but also bring together the thinking and creativity of subject-matter experts and data scientists. This positive feedback loop between different kinds of domain experts creates new opportunities for ideas to flourish far beyond the scope of individual machine learning projects.
Why do we claim that data-centric models will be better than their model-centric counterparts in almost every aspect?
Think of any high-quality consumer product you use regularly. It may be your computer, the car you drive, the chair you sit on, or something else that has required some level of design and engineering.
What makes it high-quality?
Design and functionality have a lot to do with it, but unless the product is made of quality materials, it will not work as intended or it may break altogether. Something is only high-quality if it works as intended, and does so consistently.
The same goes for machine learning models. By systematically improving data quality – our building materials – we are able to build models that are more predictive, robust, and interpretable.
We have written this book to give you, our readers, the most important background knowledge, tools, techniques, and applied examples needed to implement data-centric machine learning and take part in the next phase of the AI revolution.
In the technical chapters of this book, we will show you how to apply the principles of data-centric machine learning to real datasets, using Python. The techniques and applied examples we explore will provide you with a toolbox to systematically and programmatically collect, clean, augment, and label data, as well as to identify and remove unwanted bias.
At the end of this book, you will have a strong appreciation for the building blocks and best-practice approaches of data-centric machine learning.
Don’t just take our word for it. Let’s explore data-centric machine learning in depth.