-
Book Overview & Buying
-
Table Of Contents
-
Feedback & Rating
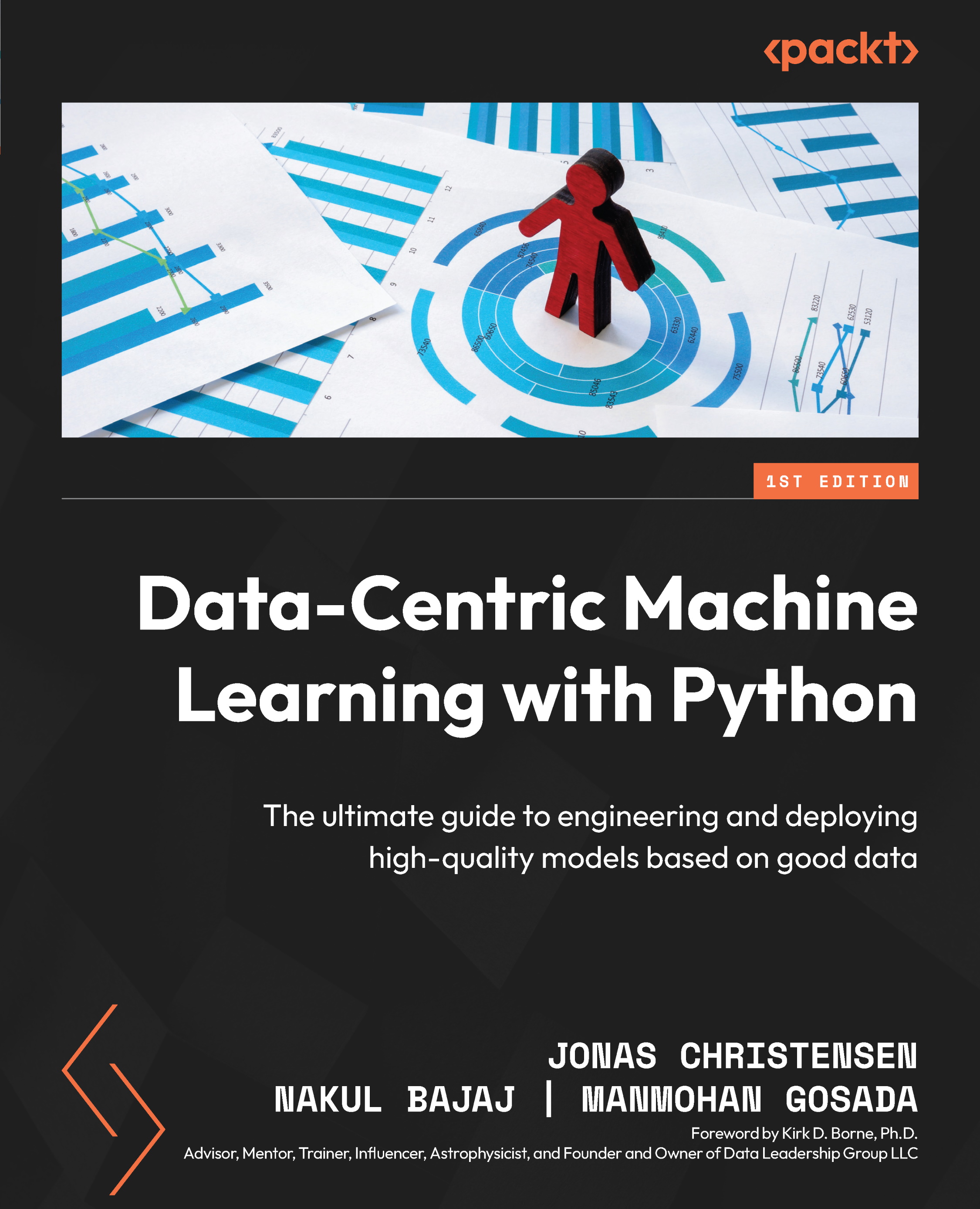
Data-Centric Machine Learning with Python
By :
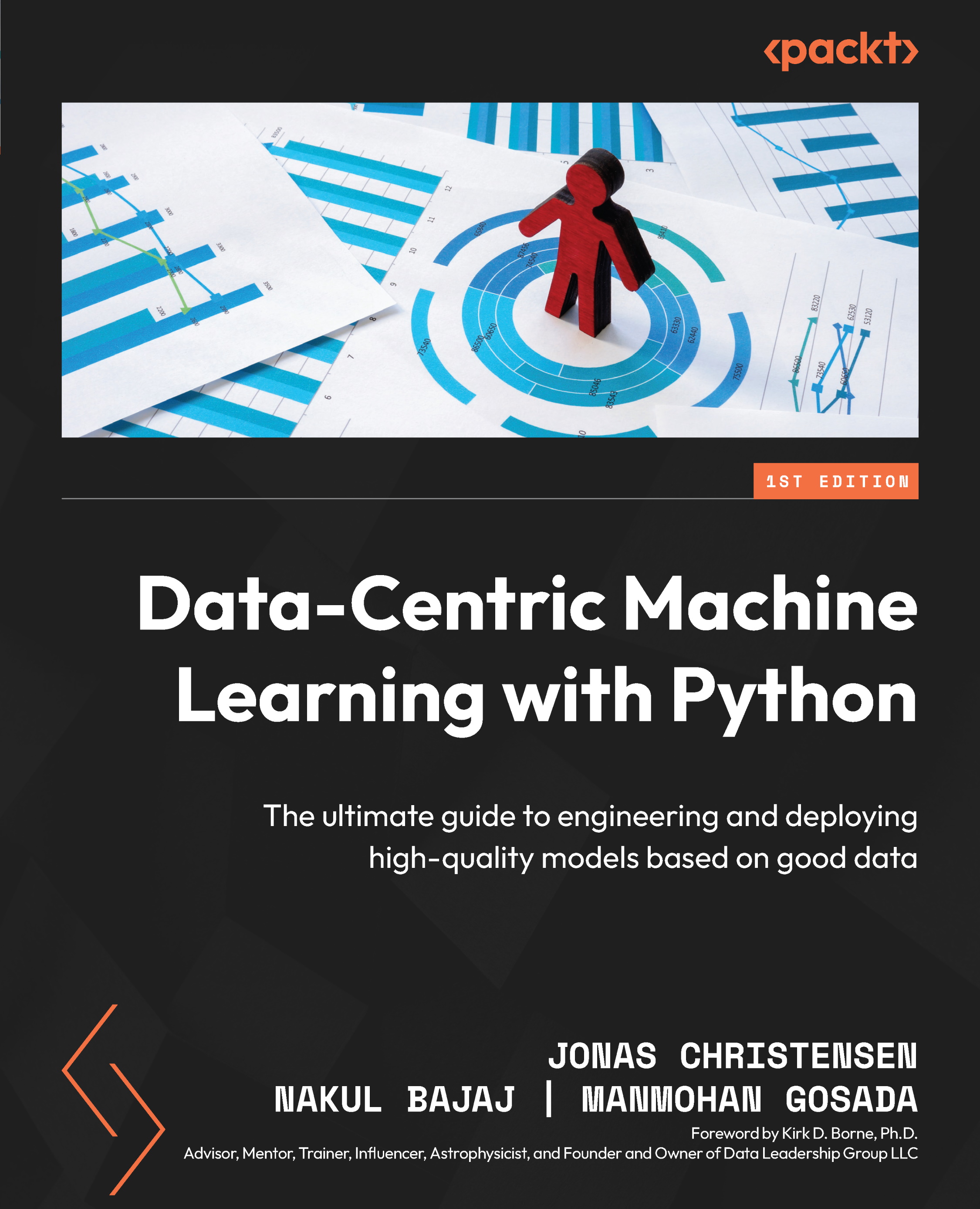
Semi-weak supervision is a technique used in machine learning to improve the accuracy of a model by combining a small set of labeled data with a larger set of weakly labeled data. In this approach, the labeled data is used to guide the learning process, while the weakly labeled data provides additional information to improve the accuracy of the model.
Semi-weak supervision is particularly useful when labeled data is limited or expensive to obtain and can be applied to a wide range of machine learning tasks, such as text classification, image recognition, and object detection.
In the loan prediction dataset, we have a set of data points representing loan applications, each with a set of features such as income, credit history, and loan amount, and a label indicating whether the loan was approved or not. However, this labeled data may be incomplete or inaccurate, which can lead to poor model performance.
To address this issue, we can use semi-weak supervision...