-
Book Overview & Buying
-
Table Of Contents
-
Feedback & Rating
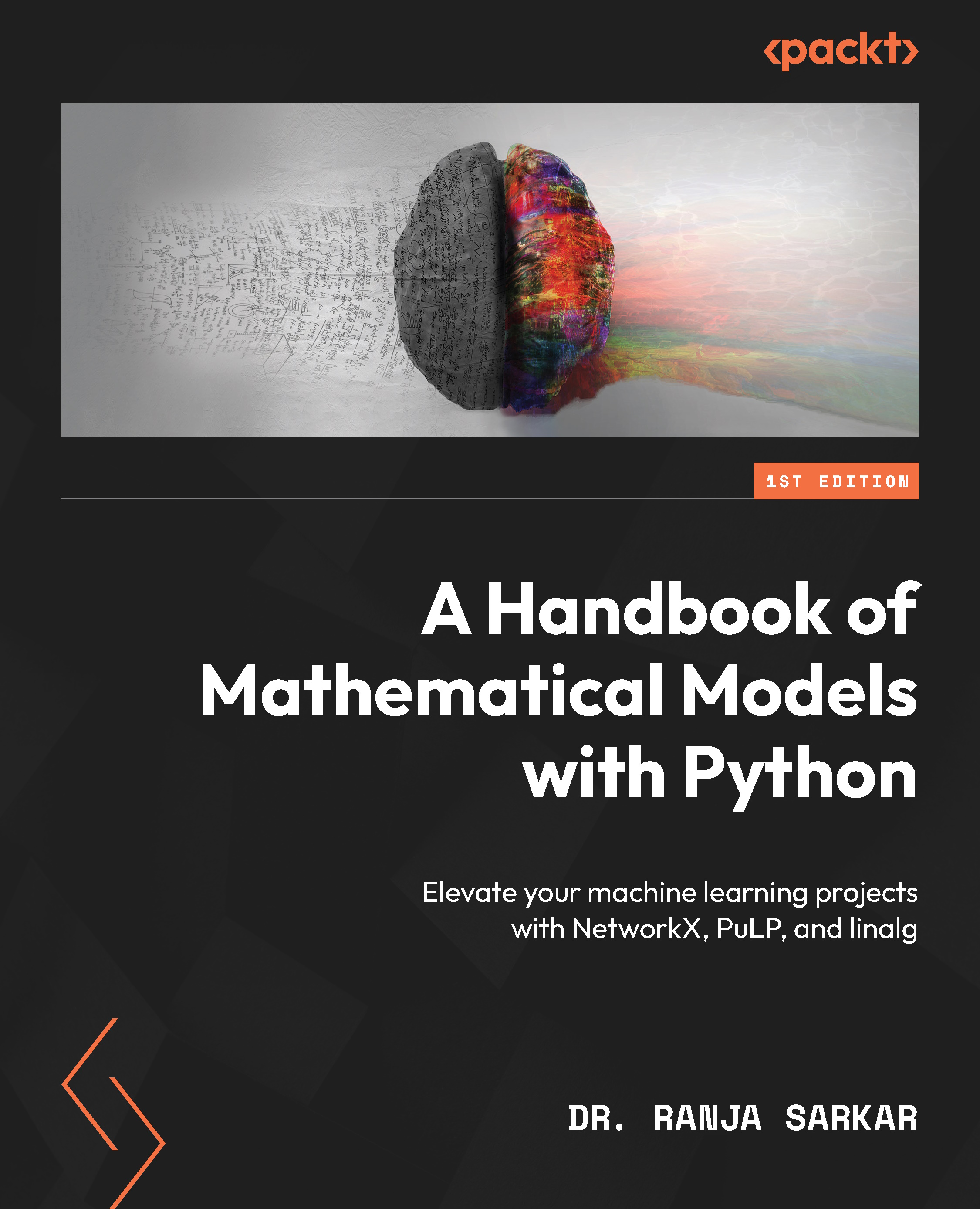
A Handbook of Mathematical Models with Python
By :
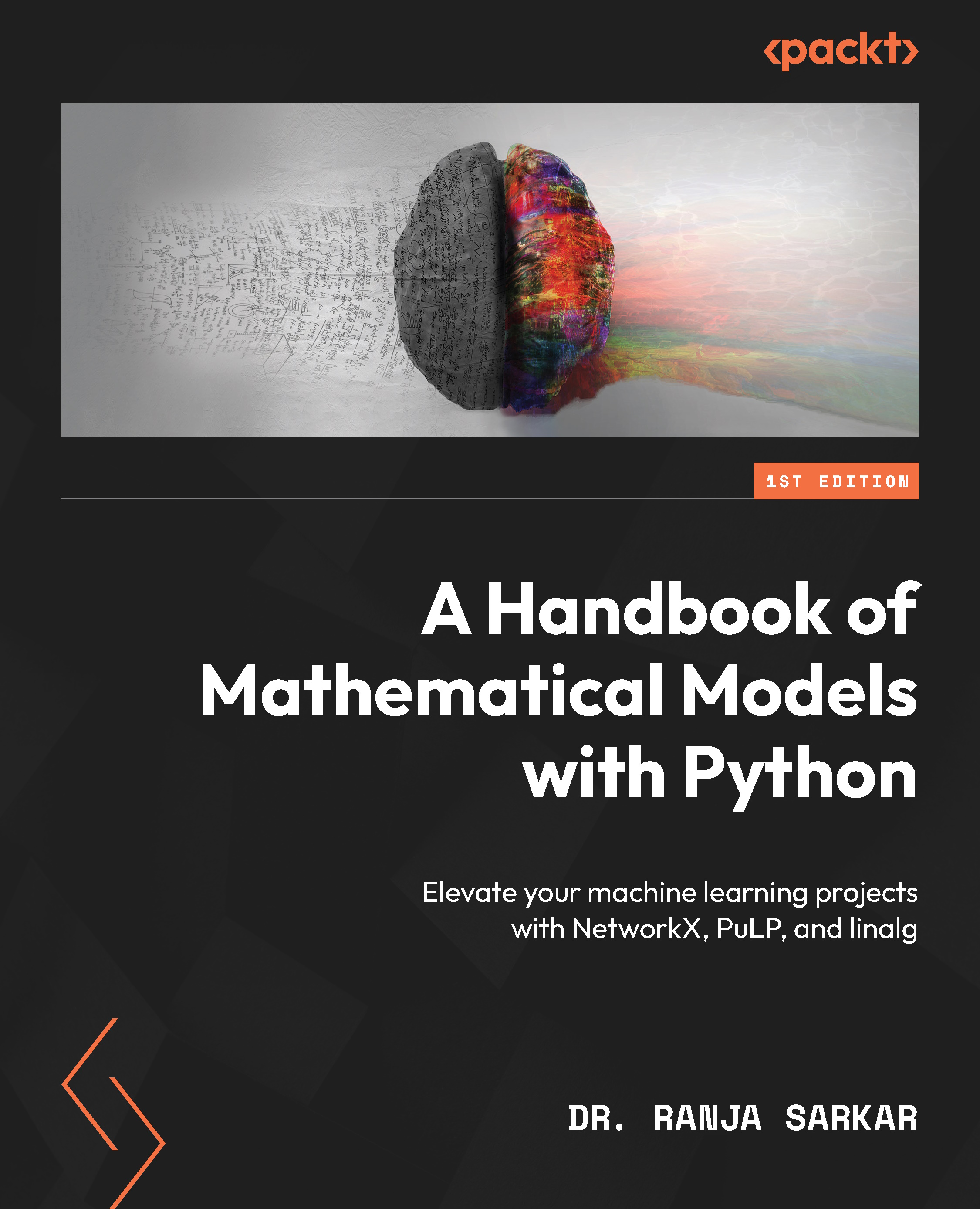
The Kalman filter has inputs and outputs like any filter. The inputs are noisy and inaccurate measurements, while the outputs are much less noisy and more accurate estimates. Mathematically speaking, the inputs to the filter are a measured value and measurement covariance matrix. The dynamic system model is the state transition matrix (representing equations) and process noise covariance matrix, the Kalman gain is internal and dependent on the system, and the outputs from the filter are the state variable and state covariance matrix. This is illustrated in Figure 7.4a:
Figure 7.4a: Input and output of the Kalman filter
When an estimate is propagated in time, the future state is inherently uncertain and hence, the error covariance matrix grows with time. The dynamic model (equations of motion) is approximate; the process noise (uncertainty) adds to the existing noise, and this is represented by the process noise covariance matrix...