-
Book Overview & Buying
-
Table Of Contents
-
Feedback & Rating
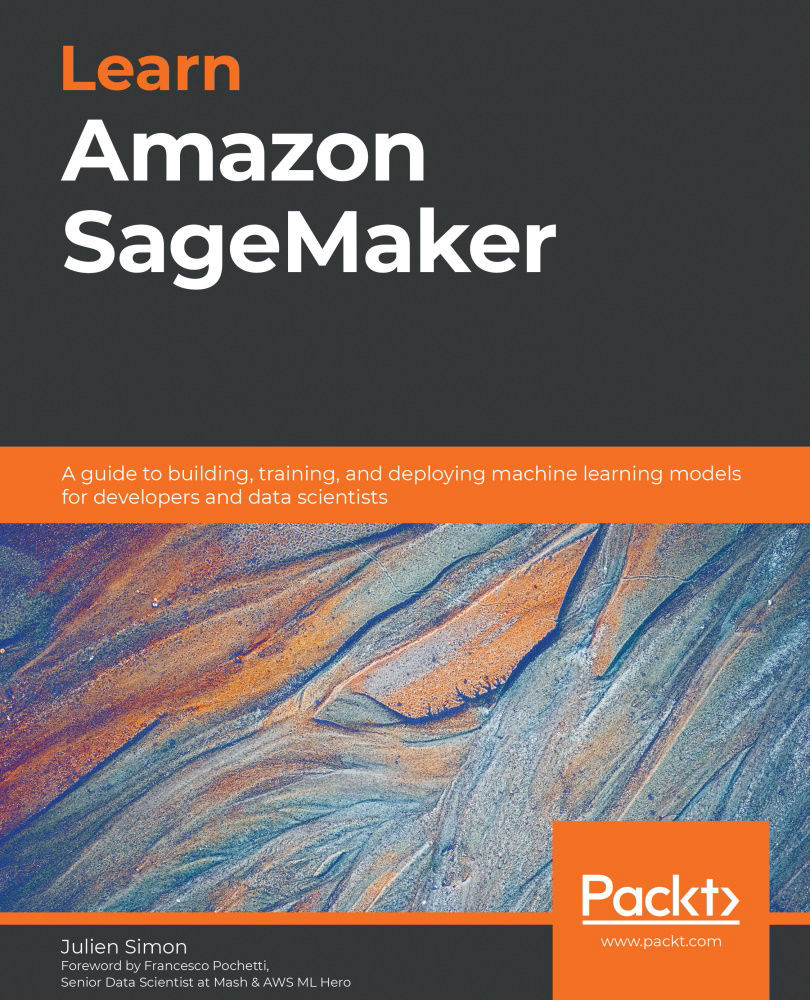
Learn Amazon SageMaker
By :
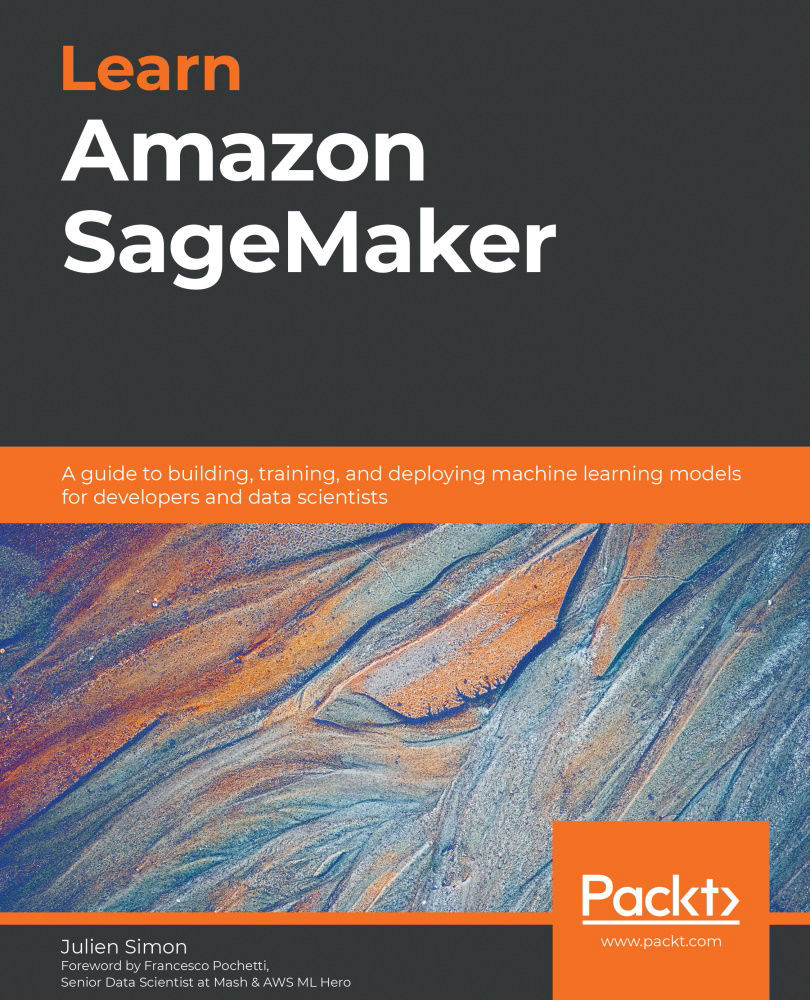
Amazon SageMaker was launched at AWS re:Invent 2017. Since then, a lot of new features have been added: you can see the full (and ever-growing) list at https://aws.amazon.com/about-aws/whats-new/machine-learning.
In this section, you'll learn about the main capabilities of Amazon SageMaker and their purpose. Don't worry, we'll dive deep on each of them in later chapters. We will also talk about the SageMaker Application Programming Interfaces (APIs), and the Software Development Kits (SDKs) that implement them.
At the core of Amazon SageMaker is the ability to build, train, optimize, and deploy models on fully managed infrastructure, and at any scale. This lets you focus on studying and solving the ML problem at hand, instead of spending time and resources on building and managing infrastructure. Simply put, you can go from building to training to deploying more quickly. Let's zoom in on each step and highlight relevant SageMaker capabilities.
Amazon SageMaker provides you with two development environments:
When it comes to experimenting with algorithms, you can choose from the following:
In addition, Amazon SageMaker Autopilot uses AutoML to automatically build, train, and optimize models without the need to write a single line of ML code.
Amazon SageMaker also includes two major capabilities that help with building and preparing datasets:
As mentioned earlier, Amazon SageMaker takes care of provisioning and managing your training infrastructure. You'll never spend any time managing servers, and you'll be able to focus on ML. On top of this, SageMaker brings advanced capabilities such as the following:
Just as with training, Amazon SageMaker takes care of all your deployment infrastructure, and brings a slew of additional features:
Just like all other AWS services, Amazon SageMaker is driven by APIs that are implemented in the language SDKs supported by AWS (https://aws.amazon.com/tools/). In addition, a dedicated Python SDK, aka the 'SageMaker SDK,' is also available. Let's look at both, and discuss their respective benefits.
Language SDKs implement service-specific APIs for all AWS services: S3, EC2, and so on. Of course, they also include SageMaker APIs, which are documented at https://docs.aws.amazon.com/sagemaker/latest/dg/api-and-sdk-reference.html.
When it comes to data science and ML, Python is the most popular language, so let's take a look at the SageMaker APIs available in boto3
, the AWS SDK for the Python language (https://boto3.amazonaws.com/v1/documentation/api/latest/reference/services/sagemaker.html). These APIs are quite low level and verbose: for example, create_training_job()
has a lot of JSON parameters that don't look very obvious. You can see some of them in the next screenshot. You may think that this doesn't look very appealing for everyday ML experimentation… and I would totally agree!
Figure 1.1 A partial view of the create_training_job() API in boto3
Indeed, these service-level APIs are not meant to be used for experimentation in notebooks. Their purpose is automation, through either bespoke scripts or Infrastructure-as-Code tools such as AWS CloudFormation (https://aws.amazon.com/cloudformation) and Terraform (https://terraform.io). Your DevOps team will use them to manage production, where they do need full control over each possible parameter.
So, what should you use for experimentation? You should use the Amazon SageMaker SDK.
The Amazon SageMaker SDK (https://github.com/aws/sagemaker-python-sdk) is a Python SDK specific to Amazon SageMaker. You can find its documentation at https://sagemaker.readthedocs.io/en/stable/.
Note:
The code examples in this book are based on the first release of the SageMaker SDK v2, released in August 2020. For the sake of completeness, and to help you migrate your own notebooks, the companion GitHub repository includes examples for SDK v1 and v2.
Here, the abstraction level is much higher: the SDK contains objects for models, estimators, models, predictors, and so on. We're definitely back into ML territory.
For instance, this SDK makes it extremely easy and comfortable to fire up a training job (one line of code) and to deploy a model (one line of code). Infrastructure concerns are abstracted away, and we can focus on ML instead. Here's an example. Don't worry about the details for now:
# Configure the training job my_estimator = TensorFlow( 'my_script.py', role=my_sageMaker_role, instance_type='ml.p3.2xlarge', instance_count=1, framework_version='2.1.0') # Train the model my_estimator.fit('s3://my_bucket/my_training_data/') # Deploy the model to an HTTPS endpoint my_predictor = my_estimator.deploy( initial_instance_count=1, instance_type='ml.c5.2xlarge')
Now that we know a little more about Amazon SageMaker, let's see how it helps typical customers make their ML workflows more agile and more efficient.
Change the font size
Change margin width
Change background colour