-
Book Overview & Buying
-
Table Of Contents
-
Feedback & Rating
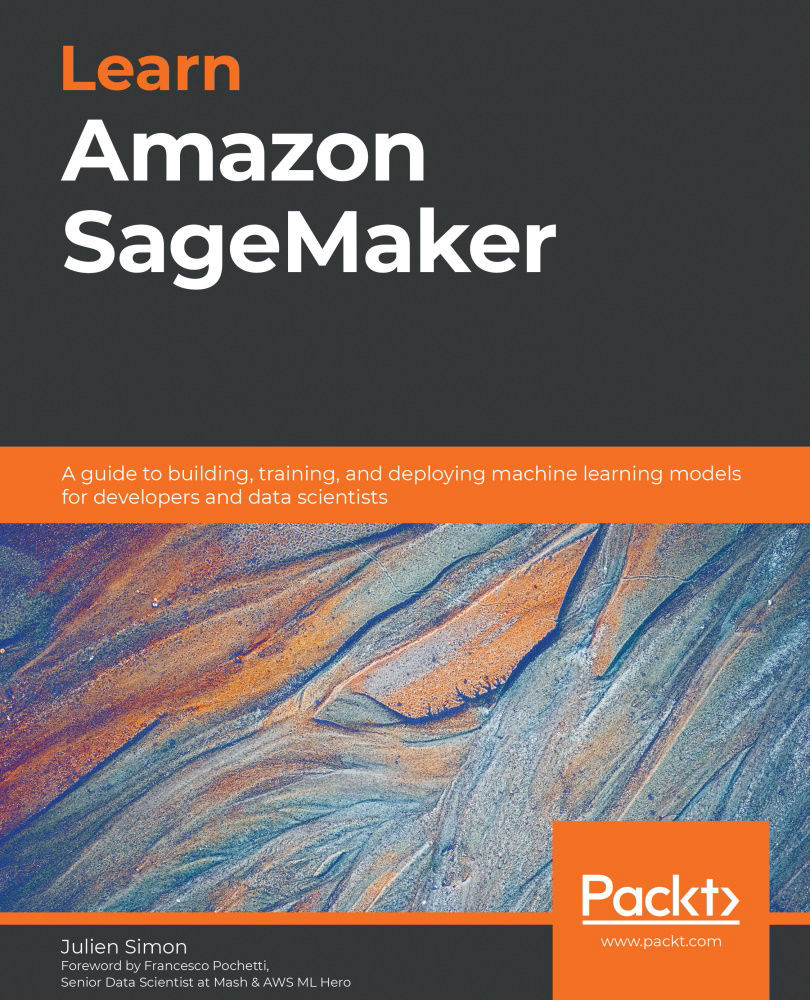
Learn Amazon SageMaker
By :
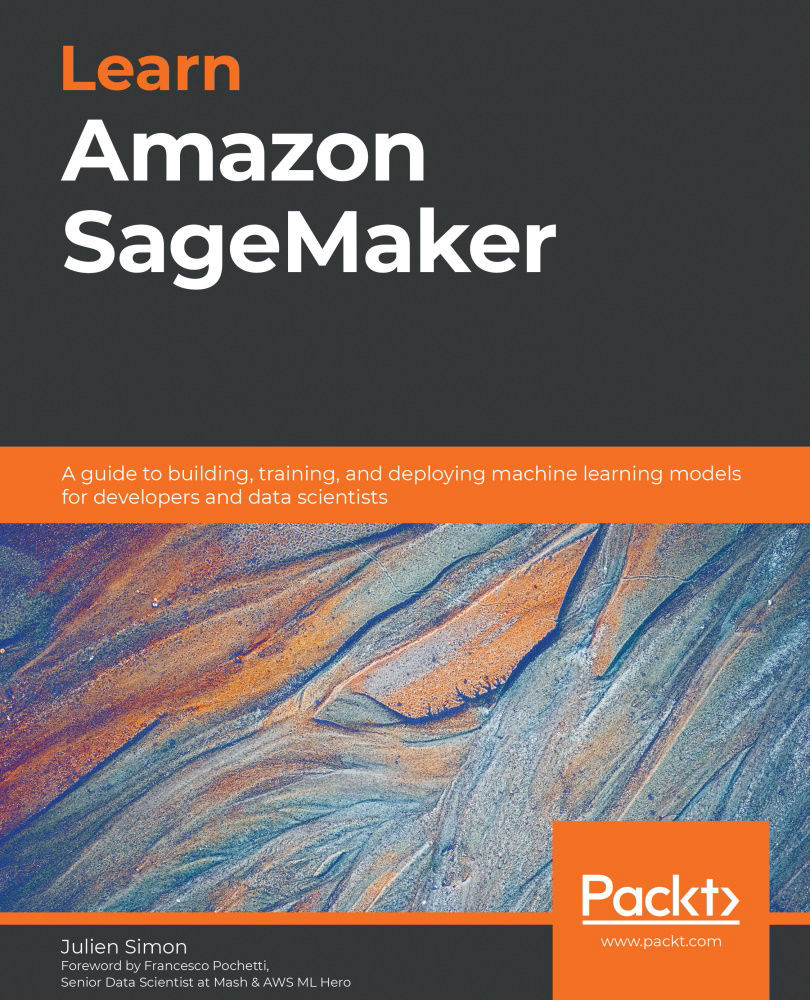
Learn Amazon SageMaker
By:
Overview of this book
Amazon SageMaker enables you to quickly build, train, and deploy machine learning (ML) models at scale, without managing any infrastructure. It helps you focus on the ML problem at hand and deploy high-quality models by removing the heavy lifting typically involved in each step of the ML process. This book is a comprehensive guide for data scientists and ML developers who want to learn the ins and outs of Amazon SageMaker.
You’ll understand how to use various modules of SageMaker as a single toolset to solve the challenges faced in ML. As you progress, you’ll cover features such as AutoML, built-in algorithms and frameworks, and the option for writing your own code and algorithms to build ML models. Later, the book will show you how to integrate Amazon SageMaker with popular deep learning libraries such as TensorFlow and PyTorch to increase the capabilities of existing models. You’ll also learn to get the models to production faster with minimum effort and at a lower cost. Finally, you’ll explore how to use Amazon SageMaker Debugger to analyze, detect, and highlight problems to understand the current model state and improve model accuracy.
By the end of this Amazon book, you’ll be able to use Amazon SageMaker on the full spectrum of ML workflows, from experimentation, training, and monitoring to scaling, deployment, and automation.
Table of Contents (19 chapters)
Preface
Section 1: Introduction to Amazon SageMaker
Chapter 1: Introduction to Amazon SageMaker
Chapter 2: Handling Data Preparation Techniques
Section 2: Building and Training Models
Chapter 3: AutoML with Amazon SageMaker Autopilot
Chapter 4: Training Machine Learning Models
Chapter 5: Training Computer Vision Models
Chapter 6: Training Natural Language Processing Models
Chapter 7: Extending Machine Learning Services Using Built-In Frameworks
Chapter 8: Using Your Algorithms and Code
Section 3: Diving Deeper on Training
Chapter 9: Scaling Your Training Jobs
Chapter 10: Advanced Training Techniques
Section 4: Managing Models in Production
Chapter 11: Deploying Machine Learning Models
Chapter 12: Automating Machine Learning Workflows
Chapter 13: Optimizing Prediction Cost and Performance
Other Books You May Enjoy
Customer Reviews