Mahout is the Apache library for open source learning. Mahout mainly uses, but is not limited to, the classification and dimensional algorithms of clustering recommend engines (collaborative filtering and classification). Mahout's objective is to provide the usual machine learning algorithms with a highly scalable implementation. If the historical data to be used is large, then Mahout is the machinery of choice. We generally find that it is not possible to process the data on a single device. With large data becoming an important area of focus, Mahout meets the need for a machine learning tool that can extend beyond a single computer. Mahout is different from other tools such as R, Weka, and so on, as its emphasis on scalability. The Mahout learning implementations are written in Java, and most but not all of them are compiled using the MapReduce paradigm...
-
Book Overview & Buying
-
Table Of Contents
-
Feedback & Rating
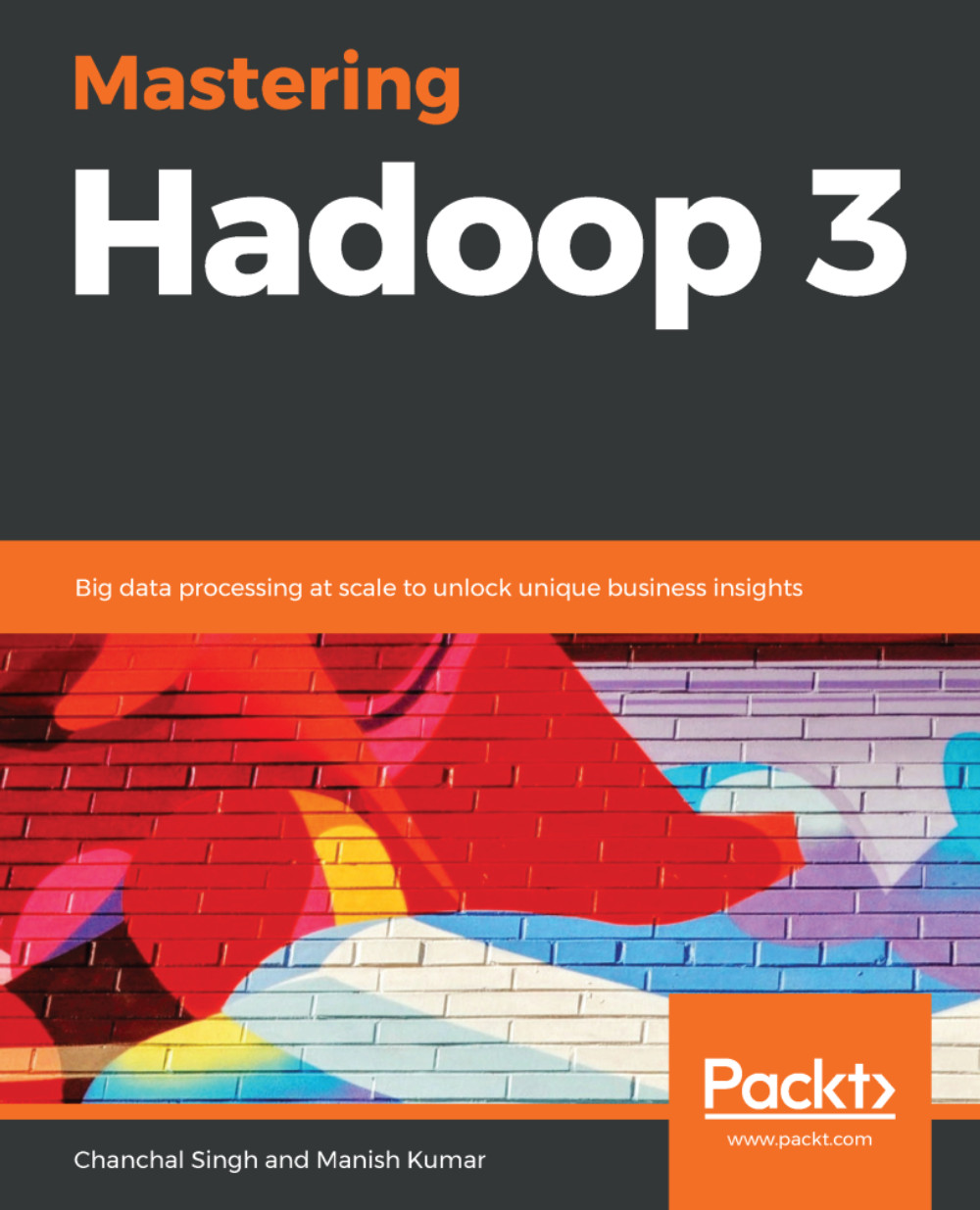
Mastering Hadoop 3
By :
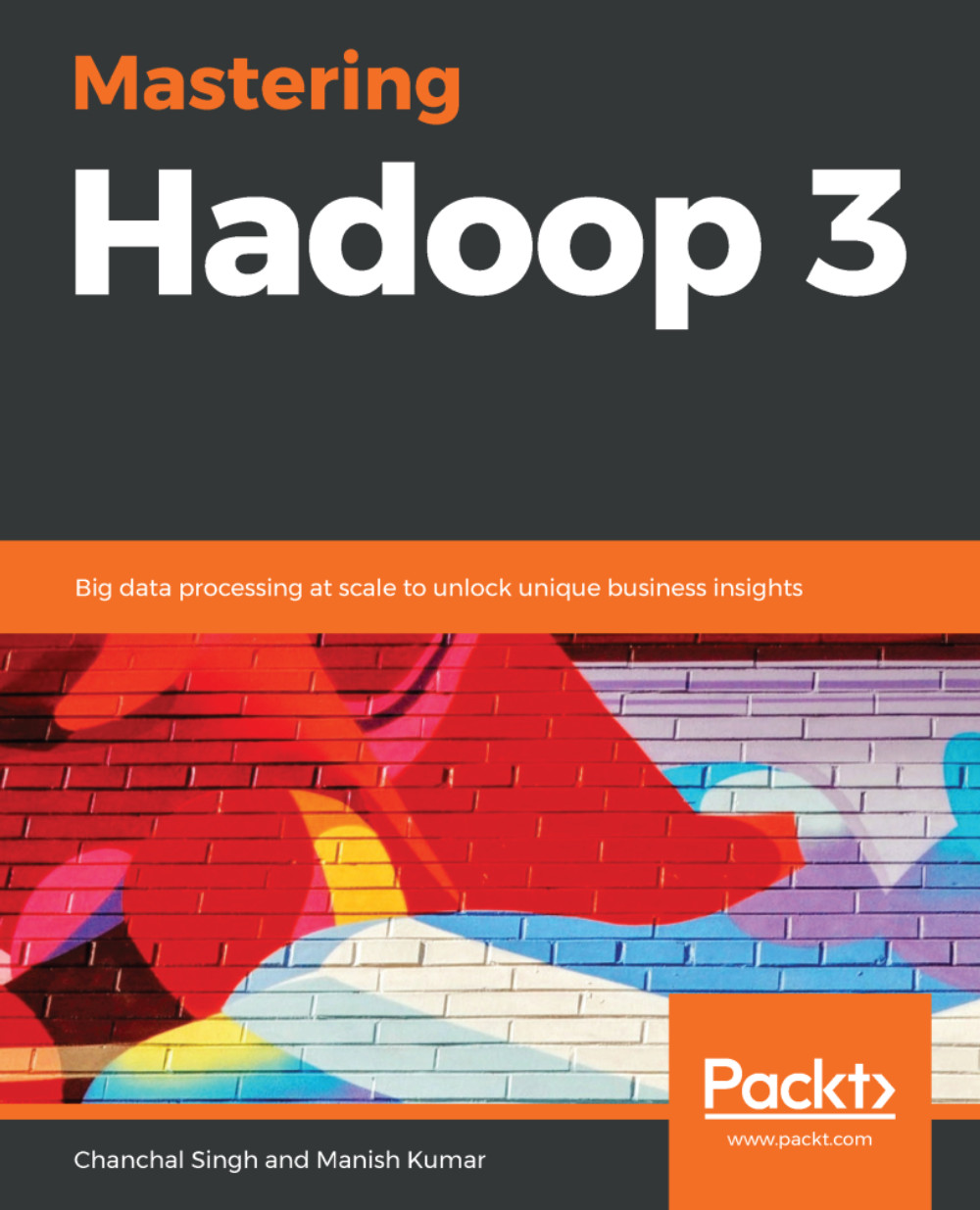
Mastering Hadoop 3
By:
Overview of this book
Apache Hadoop is one of the most popular big data solutions for distributed storage and for processing large chunks of data. With Hadoop 3, Apache promises to provide a high-performance, more fault-tolerant, and highly efficient big data processing platform, with a focus on improved scalability and increased efficiency.
With this guide, you’ll understand advanced concepts of the Hadoop ecosystem tool. You’ll learn how Hadoop works internally, study advanced concepts of different ecosystem tools, discover solutions to real-world use cases, and understand how to secure your cluster. It will then walk you through HDFS, YARN, MapReduce, and Hadoop 3 concepts. You’ll be able to address common challenges like using Kafka efficiently, designing low latency, reliable message delivery Kafka systems, and handling high data volumes. As you advance, you’ll discover how to address major challenges when building an enterprise-grade messaging system, and how to use different stream processing systems along with Kafka to fulfil your enterprise goals.
By the end of this book, you’ll have a complete understanding of how components in the Hadoop ecosystem are effectively integrated to implement a fast and reliable data pipeline, and you’ll be equipped to tackle a range of real-world problems in data pipelines.
Table of Contents (21 chapters)
Preface
Journey to Hadoop 3
Deep Dive into the Hadoop Distributed File System
YARN Resource Management in Hadoop
Internals of MapReduce
Section 2: Hadoop Ecosystem
SQL on Hadoop
Real-Time Processing Engines
Widely Used Hadoop Ecosystem Components
Section 3: Hadoop in the Real World
Designing Applications in Hadoop
Real-Time Stream Processing in Hadoop
Machine Learning in Hadoop
Hadoop in the Cloud
Hadoop Cluster Profiling
Section 4: Securing Hadoop
Who Can Do What in Hadoop
Network and Data Security
Monitoring Hadoop
Other Books You May Enjoy
Customer Reviews