-
Book Overview & Buying
-
Table Of Contents
-
Feedback & Rating
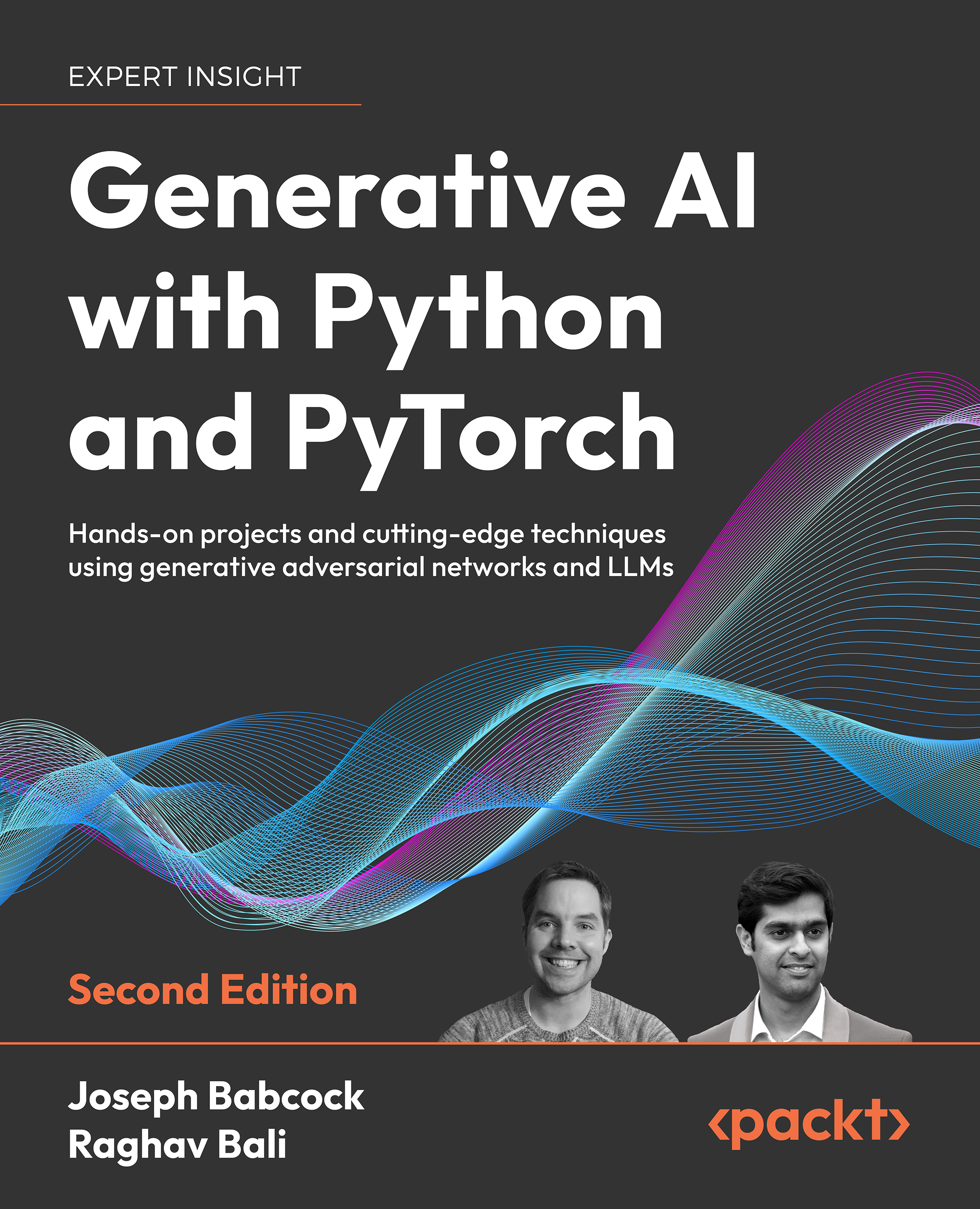
Generative AI with Python and PyTorch
By :
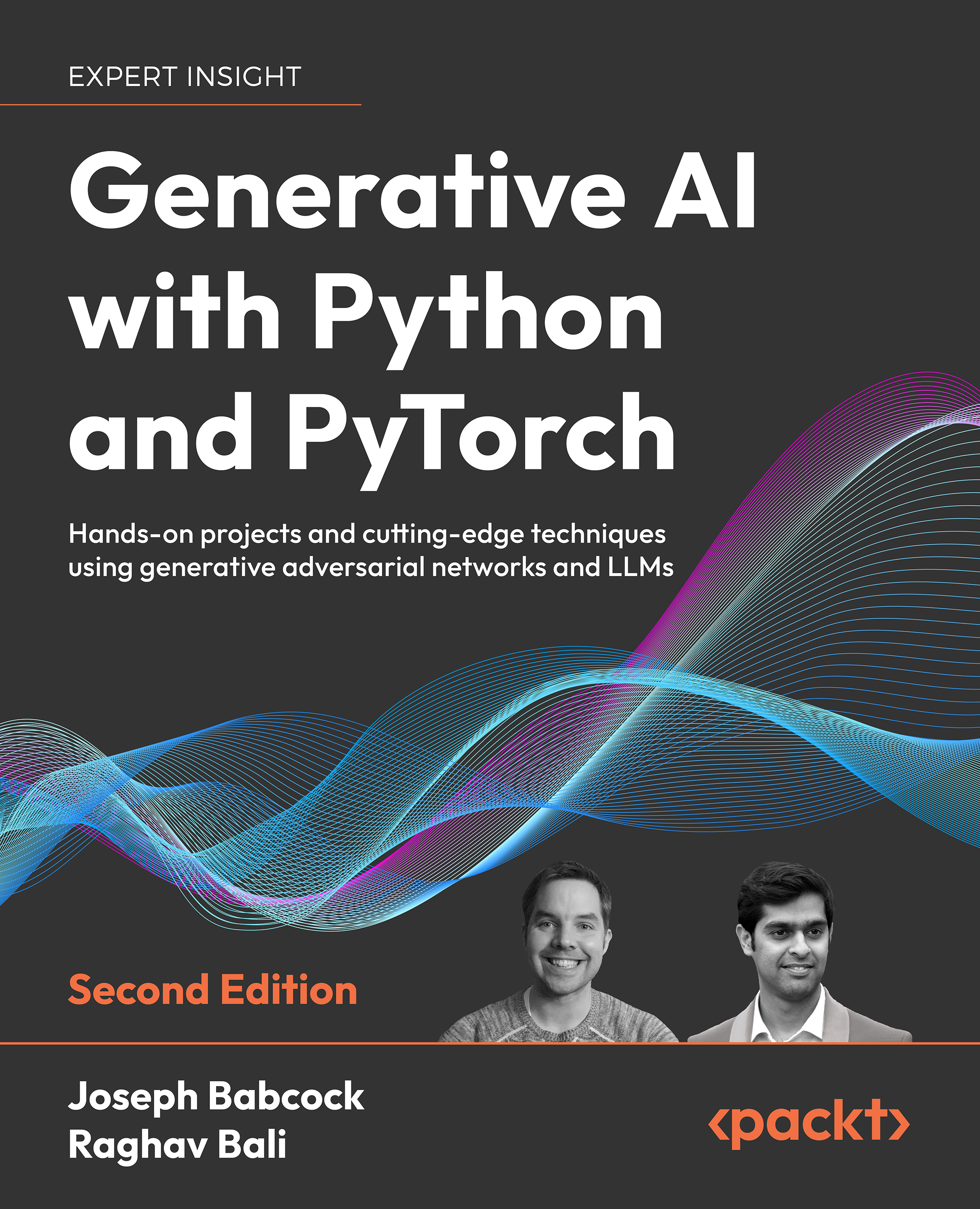
In this chapter, we've covered the basic vocabulary of deep learning—how initial research into perceptrons and MLPs led to simple learning rules being abandoned for backpropagation. We also looked at specialized neural network architectures such as CNNs, based on the visual cortex, and recurrent networks, specialized for sequence modeling. Finally, we examined variants of the gradient descent algorithm proposed originally for backpropagation, which have advantages such as momentum, and described weight initialization schemes that place the parameters of the network in a range that is easier to navigate to a local minimum.
With this context in place, we are all set to dive into projects in generative modeling, beginning with the generation of MNIST digits using Deep Belief Networks in Chapter 11, Painting Pictures with Neural Networks Using VAEs.