-
Book Overview & Buying
-
Table Of Contents
-
Feedback & Rating
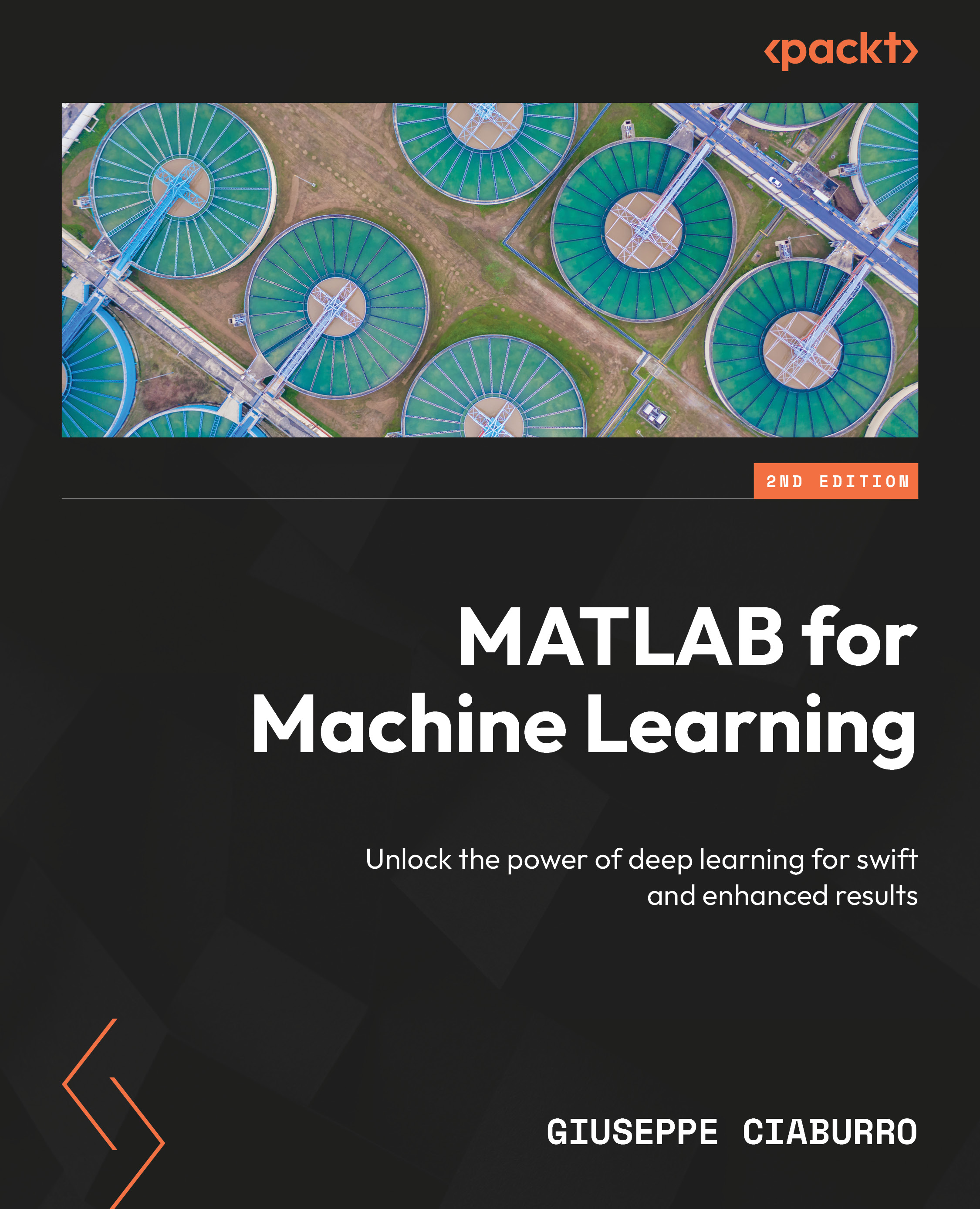
MATLAB for Machine Learning
By :
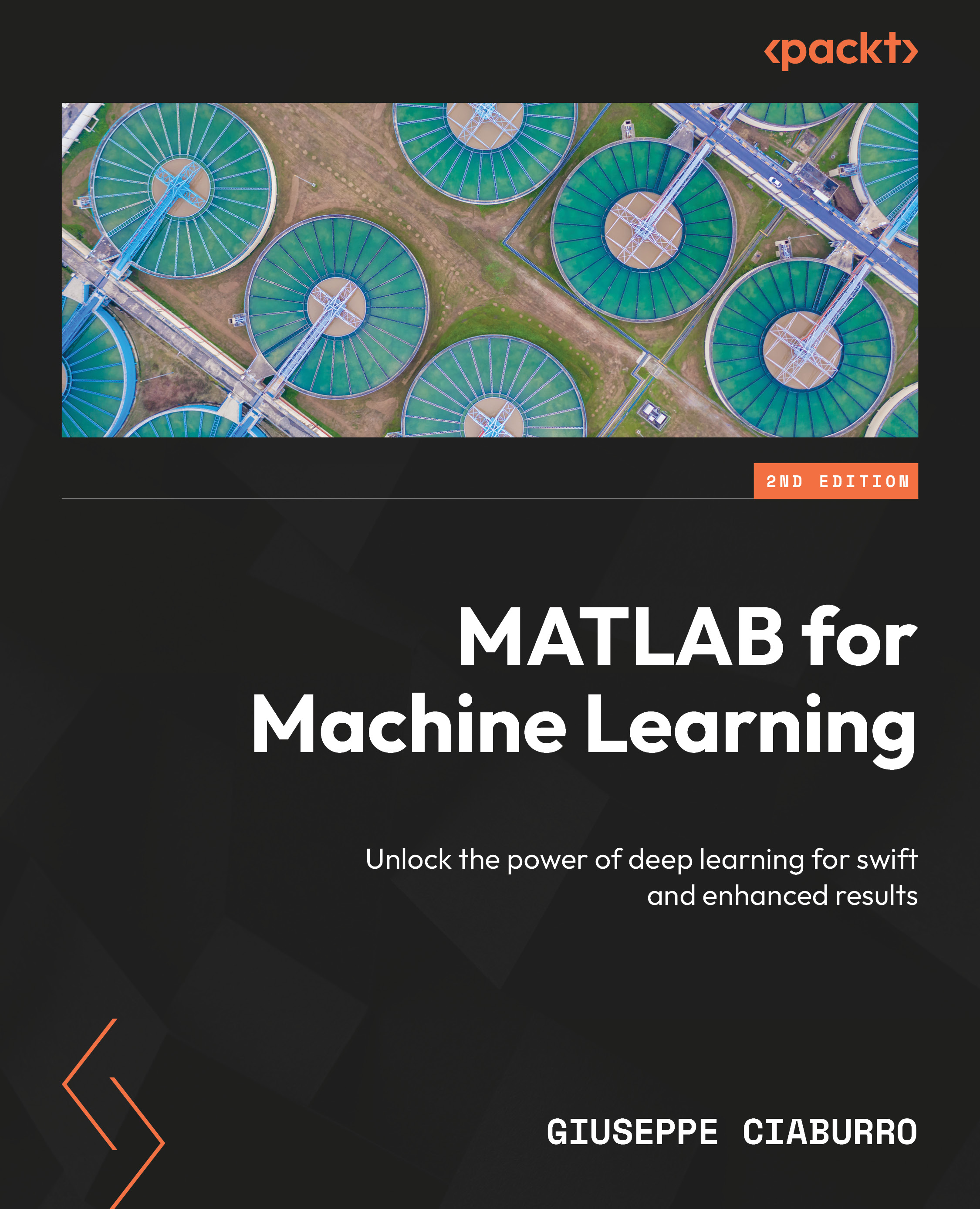
DL models are essentially multi-layered NNs, which refers to NNs that comprise multiple hidden layers (at least two) structured hierarchically. This hierarchical arrangement facilitates the sharing and reuse of information. Across this hierarchy, one can pinpoint features while disregarding unnecessary intricacies, thereby enhancing invariance. Within the realm of multi-level ML, deeper tiers acquire inputs from the outputs of prior layers and execute more complex transformations and abstractions. This layering approach to learning draws inspiration from the information processing and learning methods of mammalian brains, enabling them to react to external stimuli.
DL architectures are the fundamental blueprints that underlie the construction of DNNs, enabling them to effectively learn and represent complex patterns and features from data. These architectures define the layout, connections, and flow of information within the network, determining how...