-
Book Overview & Buying
-
Table Of Contents
-
Feedback & Rating
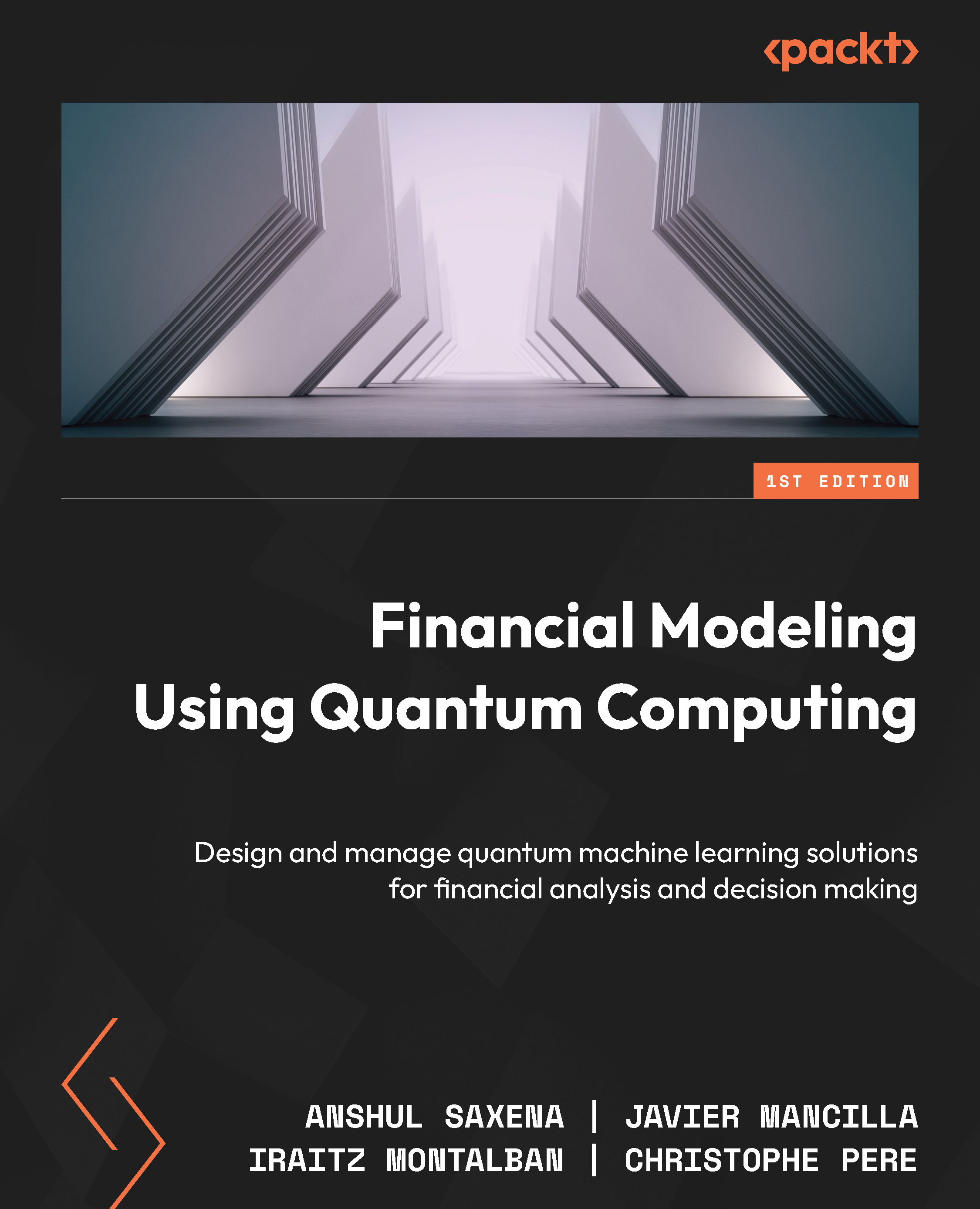
Financial Modeling Using Quantum Computing
By :
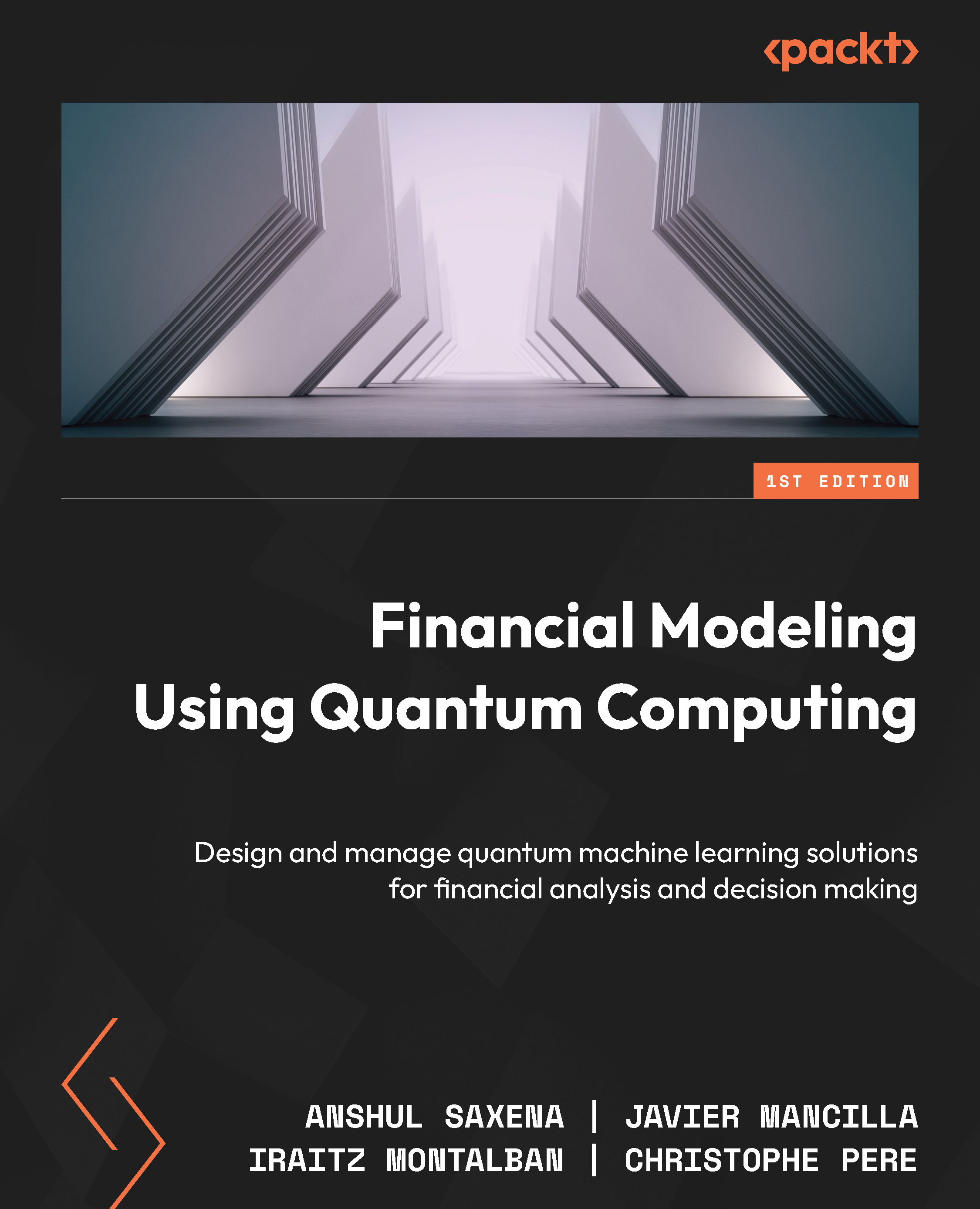
Computing paradigms can be defined as the significant milestones that have been achieved over the years. To say that computers have made the lives of humans easier is an understatement. On a daily basis, we need machines that can analyze, simulate, and optimize solutions to complex problems. Although the shapes and sizes of computers have changed over time, they still operate on the doctrines proposed by Alan Turing and John von Neumann.
In this section, we will study the evolution of quantum technology over the years. We will also study some of the technology’s limitations in the face of certain business challenges.
Turing showed us the types of problems computers can solve, von Neumann built programmable computers, and Michael Moore’s pioneering work in semiconductors made computers more capable. Figure 1.1 shows the advancement of computing paradigms over the years, and their ability to affect growth in human history:
1821 |
Mechanical calculator |
Has Enabled humans to migrate from mechanical devices to electronic devices with better accuracy in calculations. |
1890 |
Punch-card system |
Demonstrated first use case of large-scale computing by aiding in the US Census. |
1936 |
Turing machine |
Theoretical conceptual framework was laid down to solve large computational problems. |
1941 |
Digital electronic computer |
First time a computer was able to store information on its main memory. |
1945 |
Electronic Numerical Integrator and Calculator (ENIAC) |
First digital computer to perform large class of numerical problems through reprogramming. |
1958 |
Integrated Circuit (IC) |
Helped in the transition of enterprise-level computing to personal computing. |
1976 |
Cray-1 Supercomputer |
Aided 240 million calculations useful for large-scale scientific applications and simulations. |
1997 |
Parallel computing |
Multiple-CPU core was used to solve complex problems in a limited timeframe, enabling Google to form a better search engine. |
2006 |
Cloud computing |
Technology has enabled users to access large computational resources from remote locations. |
2016 |
Reprogrammable quantum computer |
Offers a better platform to solve complex simulation or optimization problems in comparison to classical computers |
2017 |
Molecular informatics |
Harnesses molecular properties for rapid, scalable information storage and processing. |
Figure 1.1 – Evolution of computing paradigms
The evolution of computing technology has enabled humans to evolve from an agrarian society to an industrial society. Progress in computing prowess has catapulted society from bartering goods to building e-commerce platforms. Figure 1.1 has given a conclusive summary of how computing technology has benefitted society through its progression from a device that merely performs calculations to the multifunction device in its present form. In the next section, we are going to assess the challenges faced by large-scale businesses and the limitations of current digital technology in addressing them.
Current digital technologies have advantages as well as limitations in providing solutions and insights in real time. The rise of numerous variables and their increasing complexity can affect decision-making in the real world. It is essential to have technology that is reliable and accurate, and fast-paced at the same time. The need for a reliable technology stack has prompted scientists worldwide to investigate technology that is beyond the reach of humans. The current challenges faced by large-scale businesses are as follows:
In order to perform a business task more efficiently and optimally, the business fraternity has started looking for technological solutions. Digital computing in its current state has helped businesses to achieve more efficiency via automation and augmented intelligence. However, current hardware technology has not been able to solve a few complex tasks, which can be associated with an abundance of data and the limitation of computing memory. The following section highlights the types of problems that can be solved by digital computing, and other problems that have generated the need to look beyond the current computing paradigm.
Digital computers are powered by integrated circuits (ICs), a technology that reached its peak in the 20th century. According to Moore’s law, the number of transistors powering microchips will double every year. In 2021, IBM announced that it can fit 50 billion transistors into its 2 nm chip technology, which basically allows a chip to fit in a space the size of a fingernail. The presence of a large number of transistors has enabled the classical computer to perform large calculations and complex procedures that help in solving day-to-day problems much faster.
However, due to internal leakages and the miniaturization effect, classical gates (OR and AND gates) have been showcasing the quantum effect. Also, digital computers are traditionally unable to solve NP-hard problems (Figure 1.2). In layman’s language, NP-hard problems are measured by the amount of time it takes to solve a problem based on the complexity and number of variables. An example of this, as discussed previously, is how to choose the optimum route out of the 8,543,811,434,435,330 combinations determined for charging station locations in the San Francisco Bay Area. While it would take years for a classical computer to solve the aforementioned problem, ideally, quantum computers can solve it in 3 seconds.
Figure 1.2 – Classification of NP-hard problems based on level of complexity
To understand the limitations of classical computers in a better way, imagine that you have to pick a portfolio of 100 penny stocks with a limited budget, and let’s assume that the prices are discrete (for example, tick size on stock markets). Suppose you have to construct a portfolio in polynomial time (p = problems a computer can solve in a reasonable amount of time) and assume that it takes 100 steps (n = no. of inputs) to obtain an optimized portfolio or, in other words, n3 time. Theoretically, digital computers will solve the problem in three hours. This problem was easy to solve, and experts can easily verify the solution since we are dealing with stocks of the same class. Hence, we can confidently say that p-class problems are easy to check and solve. Now, the same problem but with a variation (a portfolio optimization of 100 stocks belonging to different risk classes in a limited time) will take around 300 quintillion years to solve, because although the solution is verifiable in a polynomial (n) timeframe, it is obtained in an exponential (NP) timeframe. This problem is classified as an NP problem. For an analogy, imagine a sudoku or tic-tac-toe problem: this is an NP problem for which it is difficult to obtain the solution (it takes exponential time), but easy to verify in polynomial time.
Following on from the preceding discussion, four types of NP problems that are deemed difficult to be solved by digital computers are as follows:
To summarize, it will suffice to say that with the limitations observed in current technology, it is time to explore new computing paradigms that can help solve the problems faced by the business fraternity at large and help the industry bring in innovations and creativity.
Change the font size
Change margin width
Change background colour