The process of aggregating data
Although the objective of data aggregation is largely straightforward, you should follow a definitive process. The data aggregation process is a co-shared responsibility between the customer and the data engineer. Each entity needs to contribute toward the objective through a combination of business and technical knowledge:
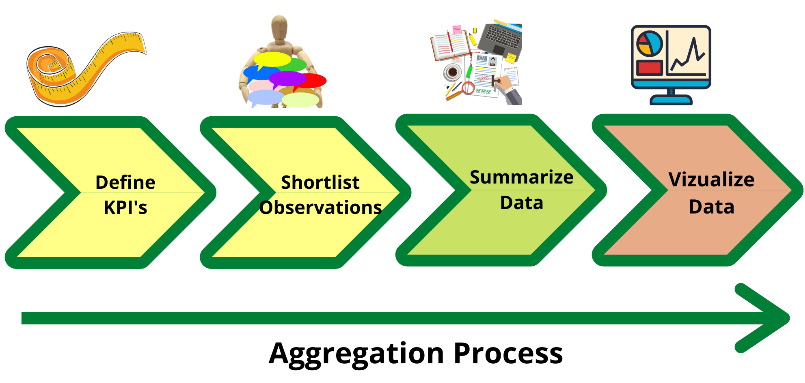
Figure 8.2 – Data aggregation process
This process can be explained as follows:
- Define KPIs: The process of data aggregation starts by defining the key performance indicators (KPIs). Data engineers are not the best resources for making such business-oriented decisions. Therefore, it is important to seek customer help on this one since they are closer to the business and can make these decisions more accurately.
Important
A quantifiable metric that's used to measure a company's success or failure is called a KPI. KPIs are often used to evaluate whether the company has met its objectives...