-
Book Overview & Buying
-
Table Of Contents
-
Feedback & Rating
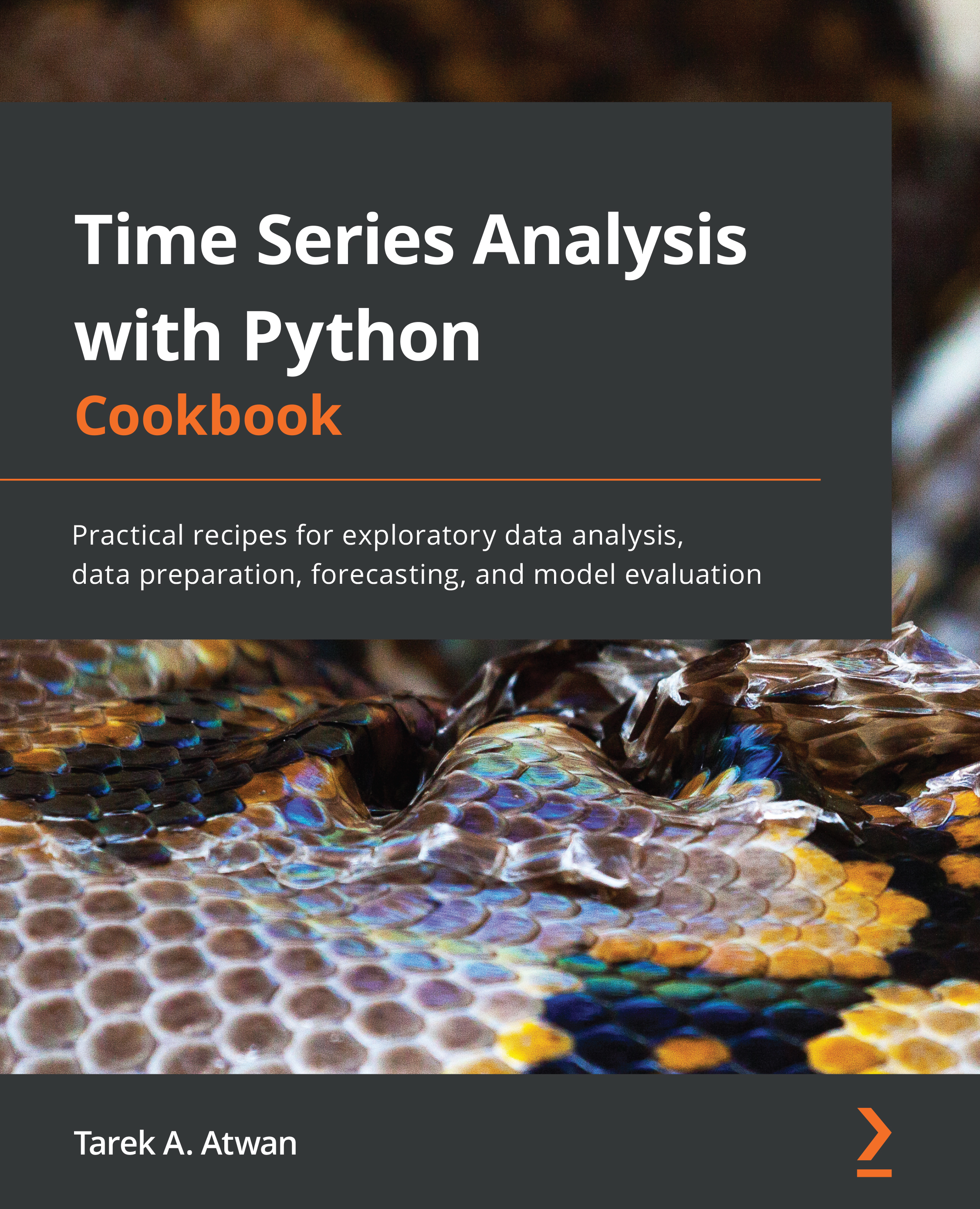
Time Series Analysis with Python Cookbook
By :
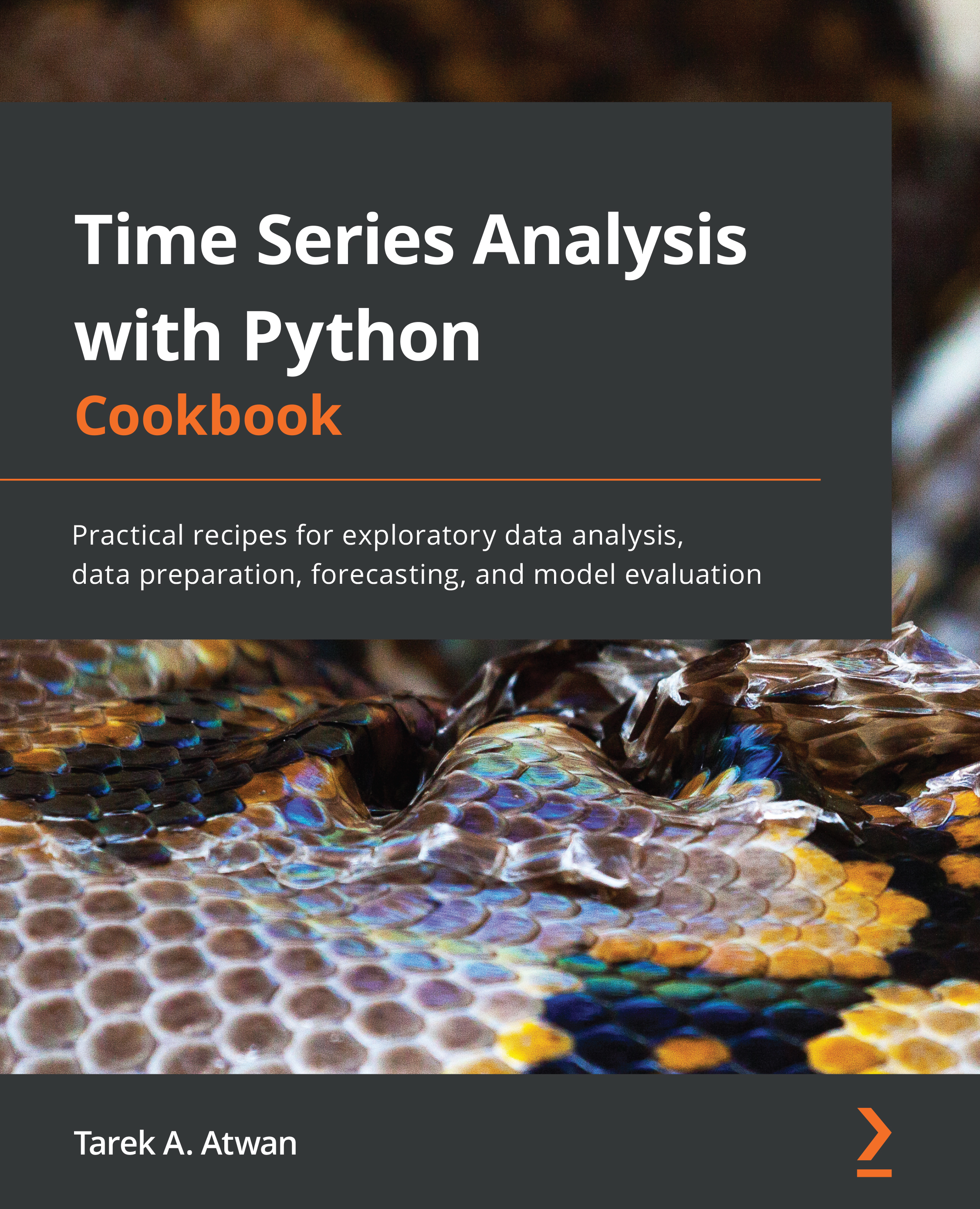
When working with time-series data that requires attention to different time zones, things can get out of hand and become more complicated. For example, when developing data pipelines, building a data warehouse, or integrating data between systems, dealing with time zones requires attention and consensus amongst the different stakeholders in the project. For example, in Python, there are several libraries and modules dedicated to working with time zone conversion; these include pytz
, dateutil
, and zoneinfo
, to name a few.
Let's discuss an inspiring example regarding time zones within time-series data. It is common for large companies that span their products and services across continents to include data from different places around the globe. For example, it would be hard to make data-driven business decisions if we neglect time zones. Let's say you want to determine whether most customers come to your e-commerce site in...