-
Book Overview & Buying
-
Table Of Contents
-
Feedback & Rating
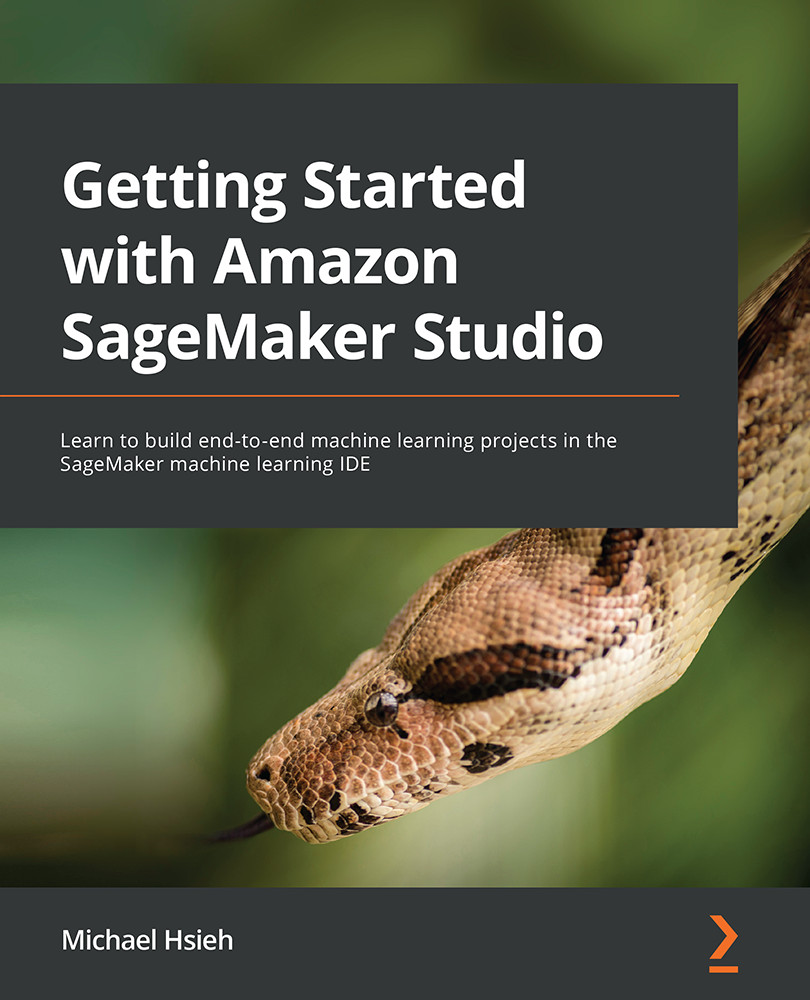
Getting Started with Amazon SageMaker Studio
By :
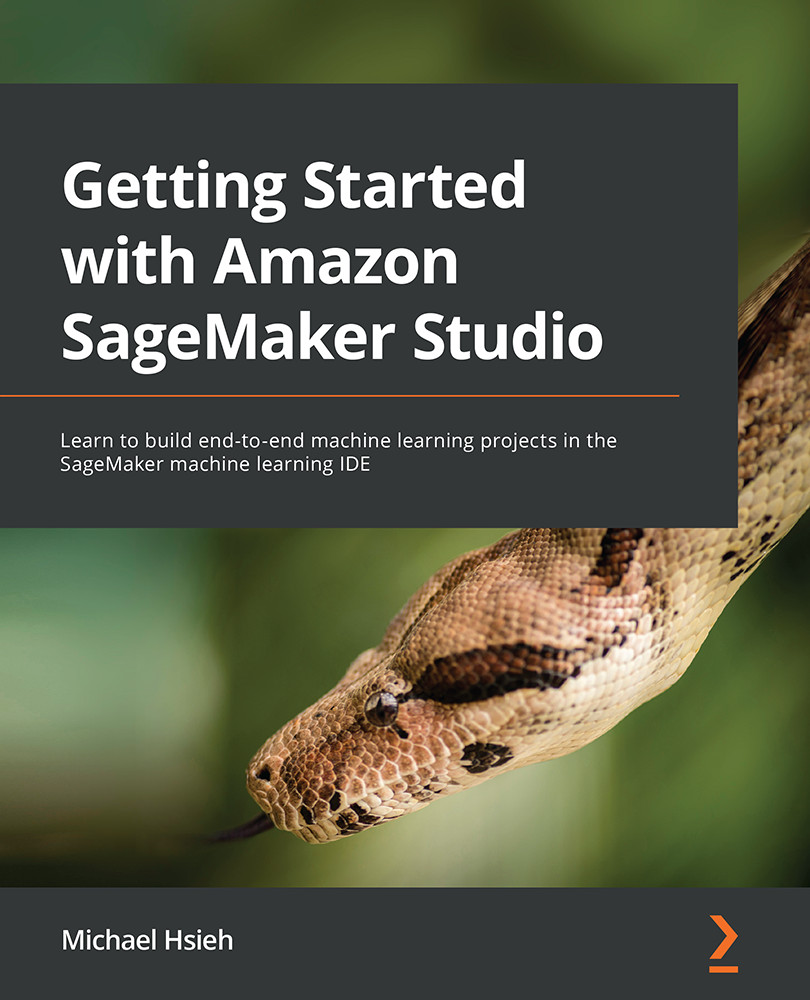
Getting Started with Amazon SageMaker Studio
By:
Overview of this book
Amazon SageMaker Studio is the first integrated development environment (IDE) for machine learning (ML) and is designed to integrate ML workflows: data preparation, feature engineering, statistical bias detection, automated machine learning (AutoML), training, hosting, ML explainability, monitoring, and MLOps in one environment.
In this book, you'll start by exploring the features available in Amazon SageMaker Studio to analyze data, develop ML models, and productionize models to meet your goals. As you progress, you will learn how these features work together to address common challenges when building ML models in production. After that, you'll understand how to effectively scale and operationalize the ML life cycle using SageMaker Studio.
By the end of this book, you'll have learned ML best practices regarding Amazon SageMaker Studio, as well as being able to improve productivity in the ML development life cycle and build and deploy models easily for your ML use cases.
Table of Contents (16 chapters)
Preface
Part 1 – Introduction to Machine Learning on Amazon SageMaker Studio
Chapter 1: Machine Learning and Its Life Cycle in the Cloud
Chapter 2: Introducing Amazon SageMaker Studio
Part 2 – End-to-End Machine Learning Life Cycle with SageMaker Studio
Chapter 3: Data Preparation with SageMaker Data Wrangler
Chapter 4: Building a Feature Repository with SageMaker Feature Store
Chapter 5: Building and Training ML Models with SageMaker Studio IDE
Chapter 6: Detecting ML Bias and Explaining Models with SageMaker Clarify
Chapter 7: Hosting ML Models in the Cloud: Best Practices
Chapter 8: Jumpstarting ML with SageMaker JumpStart and Autopilot
Part 3 – The Production and Operation of Machine Learning with SageMaker Studio
Chapter 9: Training ML Models at Scale in SageMaker Studio
Chapter 10: Monitoring ML Models in Production with SageMaker Model Monitor
Chapter 11: Operationalize ML Projects with SageMaker Projects, Pipelines, and Model Registry
Other Books You May Enjoy
Customer Reviews