-
Book Overview & Buying
-
Table Of Contents
-
Feedback & Rating
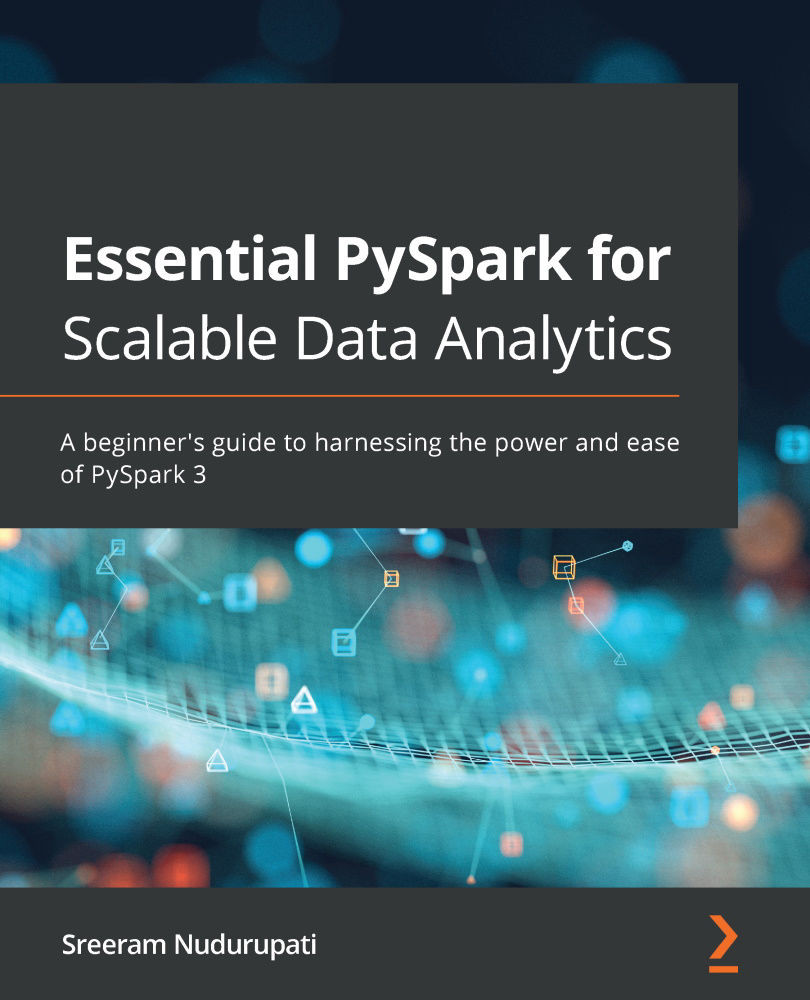
Essential PySpark for Scalable Data Analytics
By :
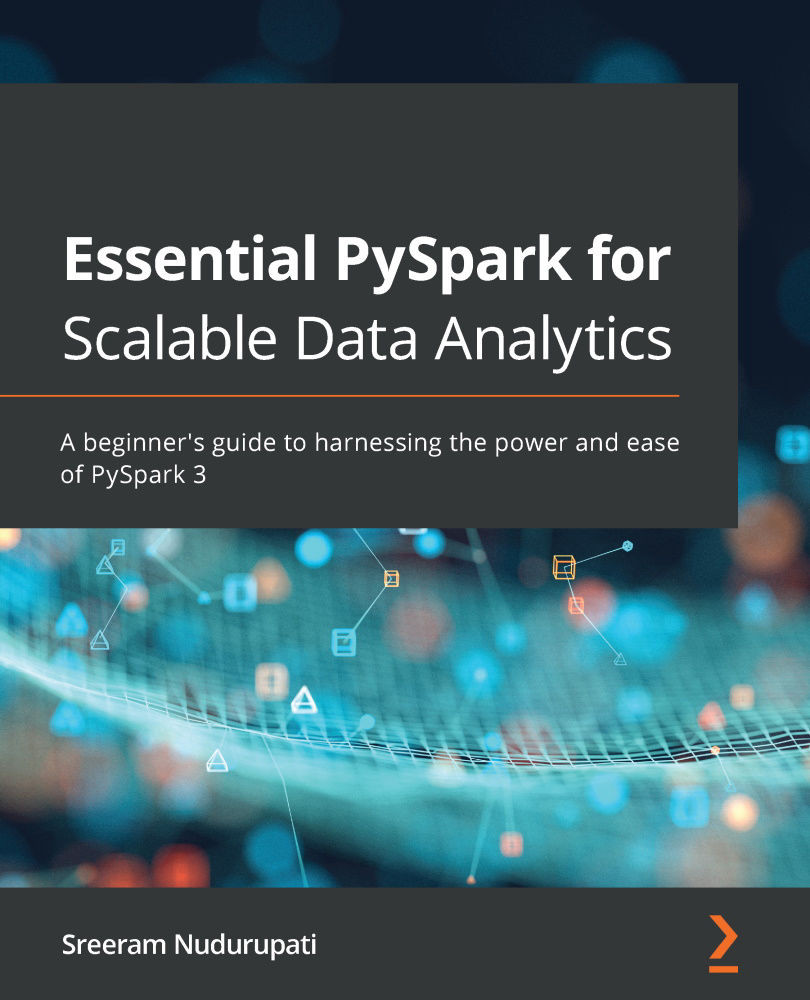
In this chapter, you were introduced to the end-to-end ML life cycle and the various steps involved in it. MLflow is a complete, end-to-end ML life cycle management tool. The MLflow Tracking component was presented, which is useful for streaming the ML experimentation process and helps you track all its attributes, including the data version, ML code, model parameters and metrics, and any other arbitrary artifacts. MLflow Model was introduced as a standards-based model format that provides model portability and reproducibility. MLflow Model Registry was also explored, which is a central model repository that supports the entire life cycle of a newly created model, from staging to production to archival. Model serving mechanisms, such as using batch and online processes, were also introduced. Finally, continuous delivery for ML was introduced. It is used to streamline the entire ML life cycle and automate the model life cycle using Model Registry features, such as the ability...